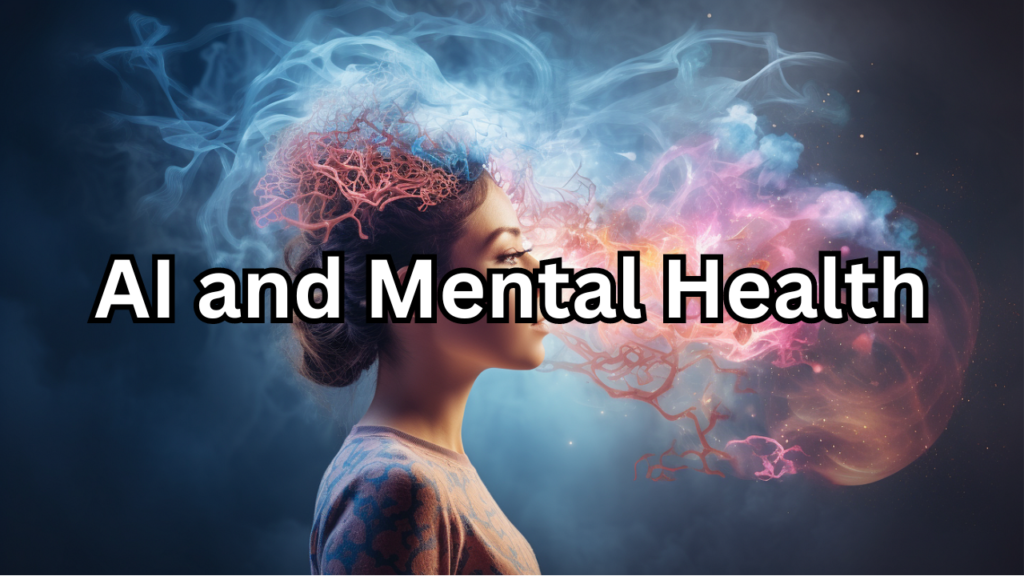
Table of Contents
Introduction
AI and Mental Health has rapidly evolved from a concept of science fiction to a transformative force across various industries, including healthcare. Its applications extend from automating routine tasks to predicting complex outcomes with remarkable accuracy. In the realm of mental health, AI holds promise as a tool to revolutionize diagnosis, treatment, and accessibility of care.
Brief Overview of AI and Its Applications
AI refers to the simulation of human intelligence processes by machines, primarily through the use of algorithms and data. Machine learning, a subset of AI, enables systems to learn and improve from experience without explicit programming. This capability allows AI to analyze vast datasets, identify patterns, and make data-driven predictions in ways that were once thought to be exclusively human.
In healthcare, AI is employed for diverse purposes:
- Diagnostic Assistance: AI algorithms can assist in diagnosing medical conditions by analyzing symptoms and medical histories.
- Personalized Treatment: AI helps in tailoring treatment plans based on individual patient data, optimizing outcomes.
- Operational Efficiency: AI streamlines administrative tasks, enhances workflow efficiency, and reduces healthcare costs.
Importance of Mental Health in Today’s Society
Mental health is increasingly recognized as a critical component of overall well-being. The World Health Organization (WHO) defines mental health as “a state of well-being in which every individual realizes their own potential, can cope with the normal stresses of life, can work productively, and is able to make a contribution to their community.” However, mental health disorders such as depression, anxiety, and schizophrenia affect millions globally, impacting not only individuals but also their families and communities.
In recent years, societal attitudes toward mental health have shifted, emphasizing the importance of early intervention, destigmatization, and accessible treatment options. The integration of mental health services into mainstream healthcare has become a priority as healthcare systems strive to meet the holistic needs of their populations.
The intersection of AI and mental health represents a promising frontier in addressing these challenges. By leveraging AI’s analytical capabilities and scalability, there is potential to enhance mental health diagnosis accuracy, personalize treatment approaches, and expand access to mental health resources globally. However, this convergence also raises ethical considerations, including data privacy, algorithm bias, and the preservation of human empathy in healthcare delivery.
Applications of AI and Mental Health
Diagnostic Tools: AI-Powered Assessment and Diagnosis
AI-powered diagnostic tools have emerged as a transformative approach in healthcare, particularly in the realm of mental health. These tools leverage artificial intelligence algorithms to analyze vast amounts of data, ranging from patient symptoms to genetic markers, enabling more accurate and timely assessments of various conditions.
AI-Powered Assessment
AI excels in assessment by:
- Data Integration: AI can process and integrate diverse data sources, including medical histories, genetic information, and real-time patient inputs.
- Pattern Recognition: Machine learning algorithms identify patterns in data that may not be apparent to human clinicians, enhancing diagnostic accuracy.
- Real-Time Analysis: AI systems can provide instant feedback and analysis, enabling continuous monitoring and rapid adjustments in treatment plans.
Examples: Chatbots and Predictive Analytics
- Chatbots:
- Functionality: AI-powered chatbots interact with users via text or voice interfaces to provide mental health support and assessments.
- Applications: They can conduct initial screenings, offer psychoeducation, and provide ongoing support by simulating human-like conversations.
- Benefits: Chatbots enhance accessibility to mental health resources, offer anonymity, and operate 24/7, providing immediate support during crises.
- Predictive Analytics:
- Usage: AI algorithms analyze historical data to predict mental health outcomes, such as risk of depression relapse or response to specific treatments.
- Applications: Clinicians can use predictive analytics to personalize treatment plans and allocate resources more efficiently.
- Benefits: Early intervention becomes possible by identifying individuals at higher risk of developing mental health disorders, thus improving outcomes and reducing healthcare costs.
Treatment and Therapy:
AI-Driven Platforms and Virtual Reality Interventions
AI-driven platforms and virtual reality (VR) interventions represent cutting-edge innovations in mental health treatment and therapy. These technologies harness artificial intelligence to provide personalized therapeutic experiences, offering new avenues for enhancing mental well-being and addressing various mental health disorders.
AI-Driven Therapy Platforms
AI-driven therapy platforms utilize machine learning algorithms to deliver therapeutic interventions and support. These platforms can:
- Personalize Treatment: AI analyzes patient data to tailor therapeutic interventions to individual needs and preferences.
- Provide Continuous Support: Virtual therapists and chatbots offer round-the-clock support, engaging with users in real-time to provide counseling and coping strategies.
- Monitor Progress: AI tracks patient progress over time, adjusting treatment plans based on ongoing data analysis and feedback.
AI-driven therapy platforms are particularly beneficial in:
- Accessibility: They overcome barriers to traditional therapy, such as geographical limitations and stigma, by offering remote access and anonymity.
- Scalability: AI enables these platforms to handle large volumes of users simultaneously, making mental health support more widely available.
Virtual Reality and AI Interventions
Virtual reality (VR) combined with AI represents a promising frontier in mental health interventions:
- Immersive Therapy: VR environments simulate real-world scenarios to expose individuals to anxiety-inducing situations in a controlled setting, aiding in desensitization and therapeutic exposure.
- Personalized Environments: AI tailors VR experiences based on patient preferences and therapeutic goals, enhancing engagement and effectiveness.
- Biofeedback Integration: VR platforms with AI can incorporate biofeedback mechanisms to monitor physiological responses (e.g., heart rate, skin conductance), facilitating real-time adjustments in therapy.
Challenges and Concerns
Ethical Issues in AI and Mental Health
The integration of artificial intelligence (AI) into mental health care raises significant ethical considerations that must be carefully addressed to ensure responsible and effective implementation. Two primary ethical concerns are privacy issues related to AI in mental health and biases inherent in AI algorithms.
Privacy Concerns with AI in Mental Health
- Data Security and Confidentiality:
- Sensitive Information: AI systems in mental health often require access to sensitive personal data, including medical records, therapy sessions, and behavioral data.
- Risk of Breaches: There is a heightened risk of data breaches and unauthorized access, potentially exposing individuals to privacy violations and identity theft.
- Informed Consent: Ensuring informed consent from patients regarding the collection, use, and storage of their data is crucial to maintaining trust and ethical standards.
- Anonymity and De-Identification:
- Protecting Identities: AI applications should anonymize patient data whenever possible to prevent the identification of individuals.
- Encryption and Security Measures: Implementing robust encryption and security measures can safeguard data during storage, transmission, and processing.
- Regulatory Compliance:
- Legal Frameworks: Adhering to existing privacy laws and regulations, such as HIPAA (Health Insurance Portability and Accountability Act) in the United States or GDPR (General Data Protection Regulation) in Europe, to protect patient privacy rights.
- Ethical Guidelines: Developing ethical guidelines specific to AI applications in mental health to guide practitioners and developers in ethical data handling practices.
Bias in AI Algorithms
- Algorithmic Bias:
- Data Bias: AI algorithms are trained on datasets that may reflect biases present in society, including racial, gender, or socioeconomic biases.
- Impact on Diagnoses: Biased algorithms can lead to disparities in diagnosis and treatment recommendations, potentially exacerbating existing inequalities in healthcare access and outcomes.
- Mitigation Strategies: Implementing bias detection tools, diversifying training datasets, and regular auditing of AI systems can mitigate algorithmic biases.
- Transparency and Accountability:
- Explainability: Ensuring AI systems are transparent in their decision-making processes, allowing clinicians and patients to understand how conclusions are reached.
- Human Oversight: Maintaining human oversight in AI-driven decisions to override biases and ensure decisions align with ethical standards and patient preferences.
- Fairness and Equity:
- Equitable Access: Designing AI systems that promote fairness and equity in healthcare delivery, ensuring all individuals receive appropriate and unbiased care.
- Ethical Evaluation: Conducting ethical evaluations of AI systems before deployment to identify and mitigate potential biases that could harm patient welfare.
Human vs. AI Interaction in Therapy
The integration of artificial intelligence (AI) into therapy raises important considerations regarding the roles of healthcare professionals, as well as the trust and acceptance of AI-driven technologies in therapeutic settings.
Role of Healthcare Professionals
- Collaborative Approach:
- Complementary Roles: AI can augment the capabilities of healthcare professionals by providing data-driven insights, automating routine tasks, and enhancing diagnostic accuracy.
- Clinical Judgment: Healthcare professionals retain a critical role in interpreting AI-generated insights, making informed decisions, and providing personalized care based on patient preferences and context.
- Patient-Centered Care:
- Empathy and Emotional Support: Human clinicians provide emotional support, empathy, and therapeutic rapport that are essential for building trust and fostering meaningful therapeutic relationships.
- Holistic Assessment: Professionals integrate AI-generated data with comprehensive patient assessments, considering psychosocial factors, cultural backgrounds, and individual experiences.
Trust and Acceptance of AI in Therapy
- Building Trust:
- Transparency: Ensuring transparency in AI algorithms and decision-making processes to clarify how recommendations are generated and to maintain patient trust.
- Evidence-Based Practice: Demonstrating the effectiveness and safety of AI-driven therapies through rigorous research and clinical trials to gain professional and public trust.
- Patient Acceptance:
- User Experience: Designing AI interfaces that are intuitive, user-friendly, and accessible to patients, promoting acceptance and engagement in therapy sessions.
- Education and Awareness: Educating patients about the capabilities and limitations of AI in therapy, addressing concerns about privacy, confidentiality, and the role of human oversight.
Future Directions
Advancements in AI and Mental Health
The field of artificial intelligence (AI) continues to advance rapidly, offering promising innovations that have the potential to revolutionize personalized treatment approaches in mental health care.
Current Landscape of AI Advancements
- AI for Diagnosis and Assessment:
- Enhanced Accuracy: AI algorithms are becoming increasingly adept at analyzing complex datasets to improve diagnostic accuracy for various mental health conditions, such as depression, anxiety disorders, and schizophrenia.
- Predictive Analytics: Machine learning models can predict treatment outcomes and identify individuals at higher risk of developing mental health disorders, enabling early intervention strategies.
- AI-Driven Therapy Platforms:
- Personalized Interventions: AI-powered therapy platforms tailor treatment plans based on individual patient data, preferences, and responses, optimizing therapeutic outcomes.
- Continuous Monitoring: Virtual therapists and chatbots provide real-time support and monitoring, enhancing accessibility and consistency of care.
Potential Future Technologies
- AI for Personalized Treatment:
- Precision Medicine: AI can analyze genetic, environmental, and behavioral data to personalize treatment approaches, matching interventions to the unique biological and psychological profiles of patients.
- Adaptive Therapies: AI algorithms can dynamically adjust therapy sessions based on real-time patient feedback and physiological responses, optimizing treatment effectiveness.
- Neuroscience and AI Integration:
- Brain-Computer Interfaces (BCIs): AI-powered BCIs can decode neural signals and facilitate direct communication between the brain and external devices, potentially aiding in the treatment of psychiatric disorders.
- Neurofeedback: AI algorithms can interpret brain activity patterns to provide real-time feedback during therapeutic exercises, promoting self-regulation and mental resilience.
- Virtual Reality (VR) and AI Combined:
- Immersive Therapy Environments: AI-enhanced VR simulations can create realistic and customizable environments for exposure therapy, cognitive-behavioral interventions, and relaxation techniques.
- Avatar-Assisted Therapy: AI-driven virtual avatars can simulate therapeutic interactions, offering personalized coaching and emotional support in a virtual setting.
Integration of AI into Healthcare
The integration of artificial intelligence (AI) into healthcare systems represents a transformative shift in how medical services, including mental health care, are delivered and managed. However, this integration necessitates careful consideration of policies, regulations, and adoption strategies to ensure ethical standards, patient safety, and equitable access to AI-driven innovations.
Policies and Regulations
- Regulatory Frameworks:
- Data Privacy and Security: Establishing regulations to protect patient data privacy and ensure secure handling of sensitive health information, aligning with standards such as GDPR (in Europe) and HIPAA (in the United States).
- Ethical Guidelines: Developing ethical guidelines for AI applications in healthcare, addressing issues such as transparency, bias mitigation, informed consent, and the responsible use of AI technologies.
- Safety and Efficacy:
- Clinical Validation: Requiring rigorous testing and clinical validation of AI algorithms and systems to demonstrate safety, efficacy, and reliability before widespread adoption.
- Post-Market Surveillance: Implementing mechanisms for continuous monitoring and evaluation of AI technologies post-deployment to identify and mitigate potential risks or issues.
Adoption in Different Healthcare Systems Globally
- Challenges and Opportunities:
- Infrastructure: Variations in healthcare infrastructure and technological readiness across different countries and regions may influence the adoption and integration of AI.
- Cultural and Regulatory Variations: Adapting AI solutions to comply with local regulatory frameworks and cultural norms regarding healthcare practices and patient interactions.
- Global Collaboration:
- Knowledge Sharing: Facilitating international collaboration and knowledge sharing among healthcare professionals, researchers, and policymakers to accelerate the adoption of AI technologies and best practices.
- Capacity Building: Supporting capacity building efforts in developing countries to enhance technical expertise and infrastructure readiness for integrating AI into healthcare delivery.
Conclusion
Summary of AI’s Current Role in Mental Health
Artificial intelligence (AI) has emerged as a pivotal tool in transforming mental health care, offering innovative solutions across diagnosis, treatment, and patient support:
- Diagnostic Advancements:
- AI algorithms analyze vast datasets to enhance diagnostic accuracy for various mental health conditions, aiding in early detection and personalized treatment planning.
- Tools such as AI-powered chatbots conduct initial screenings, provide symptom assessments, and offer continuous monitoring, improving accessibility to mental health services.
- Therapeutic Interventions:
- AI-driven therapy platforms deliver personalized interventions based on individual patient data and preferences, augmenting traditional therapeutic approaches.
- Virtual reality (VR) combined with AI facilitates immersive therapy environments for exposure therapy, cognitive-behavioral interventions, and stress management.
- Predictive Analytics:
- AI predicts treatment outcomes, identifies at-risk populations, and optimizes resource allocation, enabling proactive interventions and improving overall care delivery efficiency.
- Enhanced Access and Efficiency:
- AI technologies enhance the scalability and accessibility of mental health services, particularly in underserved communities or regions with limited healthcare resources.
- Integration with telemedicine platforms expands remote access to mental health care, overcoming geographical barriers and reducing stigma associated with seeking help.
Future Prospects and Challenges
- Advancing Personalized Treatment:
- Future AI developments will focus on leveraging big data and AI to tailor treatment plans based on individual genetic, behavioral, and environmental factors, optimizing therapeutic outcomes.
- Precision medicine approaches, combining AI with genomic research, promise to revolutionize mental health care by targeting treatments to the unique needs of each patient.
- Ethical and Regulatory Considerations:
- Addressing privacy concerns and ensuring data security in AI-driven mental health interventions remain paramount.
- Developing robust ethical guidelines and regulatory frameworks to govern AI use, including transparency, bias mitigation, informed consent, and patient autonomy.
- Integration into Healthcare Systems:
- Overcoming challenges related to healthcare system readiness, interoperability with existing technologies, and adapting AI solutions to diverse cultural and socioeconomic contexts.
- Promoting education and training for healthcare professionals to effectively integrate AI tools into clinical practice while maintaining patient-centered care and human oversight.
- Research and Innovation:
- Continued research into AI’s applications in understanding brain function, predicting treatment responses, and developing novel therapeutic interventions.
- Collaboration between academia, industry, and healthcare providers to drive innovation, validate AI technologies through rigorous clinical trials, and ensure evidence-based adoption in mental health care.