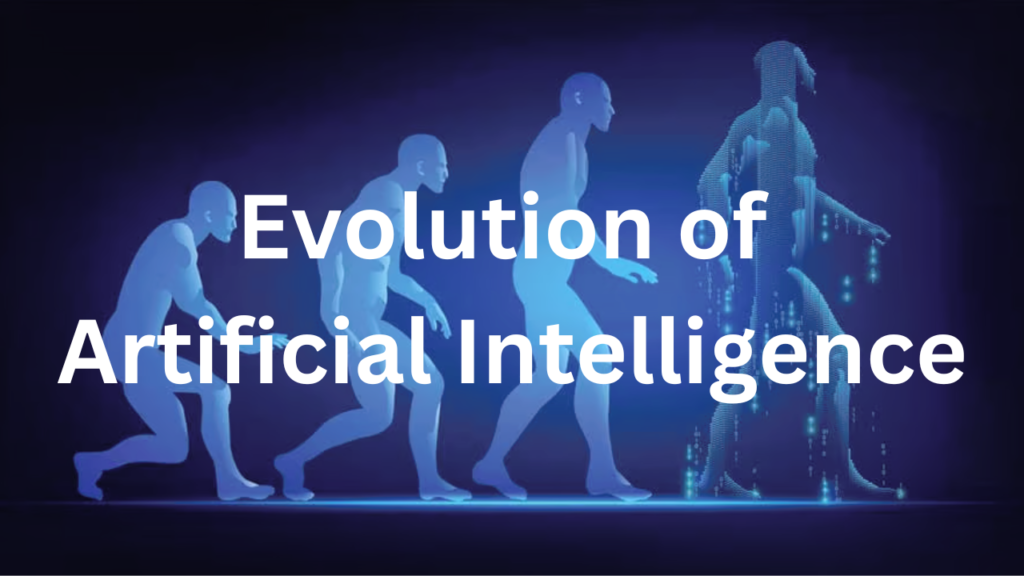
Table of Contents
Introduction
Definition of Artificial Intelligence (AI)
Artificial Intelligence (AI) refers to the simulation of human intelligence in machines that are designed to think and act like humans. These intelligent systems are capable of performing tasks that typically require human intelligence, such as learning, reasoning, problem-solving, perception, and language understanding. AI encompasses a variety of subfields, including machine learning, natural language processing, robotics, and computer vision. The goal of AI is to create systems that can function autonomously and adapt to new situations without human intervention.
Importance of Understanding Evolution of Artificial Intelligence
Understanding the evolution of Artificial Intelligence is crucial for several reasons:
- Technological Progress: AI has significantly evolved over the decades, leading to advancements in technology that impact various aspects of daily life. By understanding its evolution, we can appreciate the milestones that have shaped modern AI and anticipate future developments.
- Informed Decision-Making: Knowledge of AI’s history and progression helps policymakers, businesses, and individuals make informed decisions about the adoption and regulation of AI technologies. It allows for a better grasp of the potential benefits and risks associated with AI.
- Ethical Considerations: As AI systems become more integrated into society, ethical concerns arise regarding privacy, bias, and job displacement. Understanding AI’s evolution provides context for addressing these issues and developing frameworks for responsible AI use.
- Innovation and Research: A comprehensive understanding of AI’s past and present can inspire new research directions and innovative applications. It helps researchers build on existing knowledge and explore uncharted territories in AI.
- Public Awareness: Education the public about the Evolution of Artificial Intelligence can demystify the technology, reduce fear, and encourage a more nuanced view of its capabilities and limitations. This awareness is essential for fostering public trust and acceptance of AI systems.
By exploring the evolution of artificial intelligence, we gain valuable insights into how this transformative technology has developed and where it might be headed, enabling us to harness its potential responsibly and effectively.
Early Beginnings of AI
Conceptual Foundations
Ancient Myths and Mechanized Men
The concept of artificial beings and intelligent machines dates back to ancient civilizations. Mythologies from various cultures contain stories of mythical creatures crafted by gods or skilled artisans, such as Hephaestus’s automatons in Greek mythology or the Golem of Jewish folklore. These tales reflect humanity’s fascination with the idea of creating life-like entities endowed with intelligence and agency.
Throughout history, the notion of mechanized men, or automata, has captured the imagination of philosophers, inventors, and storytellers alike. Ancient civilizations, including ancient China, Egypt, and Greece, developed intricate mechanical devices that mimicked human or animal movements. These early automata, though rudimentary by today’s standards, laid the groundwork for later advancements in mechanical engineering and automation.
Philosophical Underpinnings: Descartes and the Mind-Body Problem
René Descartes, a prominent philosopher of the 17th century, played a pivotal role in shaping modern conceptions of the mind and artificial intelligence. Descartes famously proposed the concept of dualism, which posits that the mind and body are distinct entities—an immaterial soul or mind (res cogitans) and a material body (res extensa). This philosophical framework raised profound questions about the nature of consciousness, free will, and the possibility of creating artificial minds.
Descartes’s musings on the mind-body problem laid the foundation for discussions about the potential existence of non-biological intelligence. His assertion that the mind is fundamentally different from the body sparked debates about whether machines could ever possess true intelligence or consciousness. Descartes’s views influenced subsequent philosophers, scientists, and inventors, shaping their inquiries into the nature of cognition and the feasibility of creating thinking machines.
By examining ancient myths and philosophical inquiries like those of Descartes, we gain insight into humanity’s enduring fascination with artificial intelligence and the complex interplay between culture, philosophy, and technology in shaping our understanding of intelligent beings.
Pioneers and Theories
Alan Turing and the Turing Test
Alan Turing, a British mathematician, logician, and computer scientist, is widely regarded as one of the founding fathers of modern computer science and artificial intelligence. In 1950, Turing proposed a seminal concept known as the Turing Test, which aimed to assess a machine’s ability to exhibit intelligent behavior indistinguishable from that of a human.
The Turing Test involves a human judge interacting with both a machine and another human through a text-based interface, without knowing which is which. If the judge cannot reliably distinguish between the responses of the machine and the human, then the machine is said to have passed the Turing Test, demonstrating a level of intelligence comparable to that of a human.
Turing’s groundbreaking idea sparked considerable debate and inspired generations of researchers to pursue the goal of creating machines capable of human-like intelligence. While the Turing Test remains a controversial benchmark for AI, it remains a foundational concept in the field, highlighting the importance of natural language processing and communication skills in assessing intelligence.
John McCarthy and the Dartmouth Conference
In 1956, John McCarthy, along with a group of fellow researchers including Marvin Minsky, Nathaniel Rochester, and Claude Shannon, organized the Dartmouth Conference, often referred to as the “birth of artificial intelligence.” Held at Dartmouth College in Hanover, New Hampshire, the conference marked the beginning of systematic AI research and formalized the field as a distinct discipline.
The Dartmouth Conference aimed to explore the potential of creating intelligent machines and to lay the groundwork for future AI research. Participants discussed a wide range of topics, including problem-solving, symbolic reasoning, and machine learning, setting the agenda for AI research in the years to come.
McCarthy’s vision for AI as a scientific discipline focused on developing computer programs capable of intelligent behavior, such as reasoning, learning, and problem-solving. His efforts at Dartmouth catalyzed AI research and led to significant advancements in areas such as expert systems, natural language processing, and robotics.
The contributions of Alan Turing and John McCarthy, as well as their pioneering theories and initiatives, played instrumental roles in shaping the field of artificial intelligence and laying the groundwork for the development of intelligent machines.
The Dawn of AI Research
1950s-1960s: The Birth of AI
The 1950s and 1960s marked a period of remarkable innovation and exploration in the field of artificial intelligence (AI). Building upon earlier theoretical work and conceptualizations of intelligent machines, researchers began to develop the first computer programs aimed at simulating human cognitive abilities.
Early Computer Programs: Logic Theorist and General Problem Solver
One of the earliest AI programs developed during this period was the Logic Theorist, created by Allen Newell, J.C.R. Licklider, and Herbert A. Simon in 1956. The Logic Theorist was designed to mimic human problem-solving skills by proving mathematical theorems using symbolic logic. It demonstrated the potential for computers to perform tasks traditionally associated with human intelligence, such as logical reasoning and problem-solving.
Another significant milestone in early AI research was the development of the General Problem Solver (GPS) by Newell and Simon in 1957. The GPS was an extension of the Logic Theorist that aimed to solve a wider range of problems by employing heuristic search techniques. By encoding problem-solving strategies into a computer program, the GPS demonstrated the feasibility of automated problem-solving and laid the groundwork for future AI systems.
Symbolic AI: The Era of Rule-Based Systems
During the 1950s and 1960s, symbolic AI, also known as “good old-fashioned AI” (GOFAI), emerged as a dominant approach to artificial intelligence. Symbolic AI focused on representing knowledge and reasoning using symbolic representations, such as logic and rules.
Rule-based systems became a hallmark of symbolic AI research during this era. These systems utilized sets of logical rules to manipulate symbols and make inferences about the world. Early examples included expert systems designed to emulate the decision-making processes of human experts in specific domains, such as medicine or finance.
The birth of AI in the 1950s and 1960s laid the foundation for subsequent advancements in the field, demonstrating the potential of computers to perform intelligent tasks and sparking widespread interest in AI research. The development of early computer programs like the Logic Theorist and General Problem Solver, as well as the rise of symbolic AI and rule-based systems, represented significant milestones in the quest to create intelligent machines.
1970s: AI Winter
The 1970s marked a challenging period for the field of artificial intelligence (AI), often referred to as the “AI Winter.” Following the initial excitement and optimism of the 1950s and 1960s, AI research faced a series of setbacks and challenges that led to a decline in funding and interest in the field.
Challenges and Limitations of Early AI
Despite early successes and promising developments, AI researchers encountered significant challenges and limitations during the 1970s that contributed to the onset of the AI Winter. Some of the key challenges included:
- Complexity of Intelligence: Early AI systems struggled to handle the complexity and ambiguity of real-world tasks. While they excelled in well-defined domains with clear rules, such as chess or theorem proving, they struggled with tasks requiring common-sense reasoning, natural language understanding, and context-dependent decision-making.
- Computational Resources: The computational resources available during the 1970s were limited compared to today’s standards. AI researchers faced constraints in terms of processing power, memory capacity, and storage, which restricted the complexity and scalability of AI systems.
- Knowledge Representation: Representing and encoding knowledge in AI systems posed significant challenges. Early AI approaches relied heavily on symbolic representations and rule-based systems, which struggled to handle the complexity and uncertainty inherent in real-world knowledge domains.
- Lack of Data: AI algorithms require large amounts of data to learn and generalize from examples. However, during the 1970s, access to high-quality datasets was limited, hindering the development of data-driven AI approaches such as machine learning.
Reduced Funding and Interest
As AI research encountered mounting challenges and limitations, funding for AI projects began to wane, and interest in the field declined. Government agencies and private organizations scaled back their investments in AI research, redirecting resources to other areas deemed more promising or practical.
The AI Winter of the 1970s was characterized by a sense of disillusionment and skepticism regarding the feasibility of creating truly intelligent machines. Many researchers and industry stakeholders became pessimistic about the prospects of AI and shifted their focus to other areas of computer science and technology.
Despite the challenges and setbacks of the AI Winter, the field of artificial intelligence continued to evolve and rebound in subsequent decades, fueled by advancements in computing technology, algorithms, and data availability. The lessons learned during this period laid the groundwork for the resurgence of AI research and the emergence of new paradigms and approaches in the field.
Renaissance and Growth
1980s: Expert Systems
The 1980s witnessed the rise of expert systems as a prominent paradigm in artificial intelligence (AI) research and application. Expert systems were designed to emulate the problem-solving abilities of human experts in specific domains by capturing and codifying their knowledge into a computer program.
Development and Implementation of Expert Systems
During the 1980s, significant progress was made in the development and implementation of expert systems across various domains. Expert systems typically consisted of three main components:
- Knowledge Base: The knowledge base contained a collection of domain-specific facts, rules, heuristics, and problem-solving strategies acquired from human experts. This knowledge was represented in a structured format that could be interpreted and used by the expert system.
- Inference Engine: The inference engine was responsible for reasoning and making inferences based on the information stored in the knowledge base. It employed various inference mechanisms, such as rule-based reasoning, forward and backward chaining, and uncertainty handling, to generate solutions to problems.
- User Interface: The user interface provided a means for users to interact with the expert system, input queries or problems, and receive responses or recommendations. User interfaces varied depending on the application and could range from text-based interfaces to graphical user interfaces (GUIs).
Expert systems were successfully deployed in a wide range of domains, including medicine, finance, engineering, and manufacturing. They demonstrated the ability to provide expert-level advice, diagnostic assistance, decision support, and troubleshooting in complex and specialized domains.
Commercial Applications and Success Stories
The commercialization of expert systems led to their widespread adoption in industry and the emergence of success stories across various sectors. Some notable examples of commercial applications of expert systems in the 1980s include:
- MYCIN: Developed in the 1970s but gaining prominence in the 1980s, MYCIN was an expert system designed to assist physicians in diagnosing bacterial infections and recommending antibiotic treatments. It demonstrated high levels of accuracy and reliability in its recommendations, showcasing the potential of expert systems in healthcare.
- Dendral: Dendral was one of the earliest expert systems developed in the late 1960s and 1970s for chemical analysis. In the 1980s, Dendral and its successor, Meta-Dendral, continued to advance the field of expert systems in chemistry, providing valuable insights and predictions for organic chemical structure determination.
- XCON: XCON was an expert system developed by Digital Equipment Corporation (DEC) in the 1980s to configure and customize computer systems based on customer requirements. XCON streamlined the configuration process, reducing lead times and improving customer satisfaction, leading to significant cost savings for DEC.
The success of expert systems in the 1980s demonstrated the practical utility and potential of AI technologies in solving real-world problems and enhancing productivity across diverse industries. Expert systems paved the way for subsequent advancements in AI research and the development of more sophisticated and versatile AI applications.
1990s: Machine Learning Emergence
The 1990s witnessed a significant emergence of machine learning as a dominant paradigm within the field of artificial intelligence (AI). Machine learning algorithms, which enable computers to learn from data and improve their performance over time without explicit programming, gained widespread attention and adoption during this decade.
Introduction to Machine Learning
Machine learning is a subfield of AI that focuses on developing algorithms and techniques that enable computers to learn patterns and relationships from data and make predictions or decisions based on that knowledge. Unlike traditional rule-based programming, where explicit instructions are provided to perform tasks, machine learning algorithms learn from examples and experience, iteratively refining their models through training and feedback.
The rise of machine learning in the 1990s was fueled by advancements in computational power, data availability, and algorithmic innovation. Researchers and practitioners recognized the potential of machine learning to tackle complex problems across diverse domains, including pattern recognition, natural language processing, computer vision, and predictive analytics.
Key Algorithms: Decision Trees, Neural Networks
Two key machine learning algorithms that gained prominence during the 1990s were decision trees and neural networks.
- Decision Trees: Decision trees are a versatile and intuitive machine learning algorithm used for classification and regression tasks. Decision trees recursively partition the feature space into subsets based on the values of input features, ultimately leading to a tree-like structure where each leaf node represents a class label or regression value. Decision trees are easy to interpret and can handle both categorical and numerical data, making them suitable for a wide range of applications.
- Neural Networks: Neural networks, inspired by the structure and function of the human brain, are a class of algorithms capable of learning complex patterns and relationships from data. In the 1990s, neural networks experienced a resurgence of interest, fueled by advancements in training algorithms, network architectures, and computational resources. Multi-layer perceptrons (MLPs) and backpropagation became popular techniques for training neural networks, enabling them to learn hierarchical representations of data and achieve state-of-the-art performance in tasks such as image recognition, speech recognition, and natural language processing.
The emergence of machine learning in the 1990s paved the way for a new era of AI research and applications, where data-driven approaches and statistical learning techniques played a central role in solving real-world problems. Decision trees and neural networks were among the pioneering algorithms that laid the foundation for the modern machine learning landscape, setting the stage for further advancements in the years to come.
The Rise of Modern AI
Early 2000s: Big Data and Advanced Algorithms
The early 2000s marked a transformative period for artificial intelligence (AI), characterized by the convergence of big data and advanced algorithms. Rapid advancements in computing technology, coupled with the proliferation of digital data sources, led to the emergence of new opportunities and challenges in AI research and development.
Impact of Big Data on AI Development
The advent of big data revolutionized the field of AI by providing unprecedented access to vast and diverse datasets. Big data refers to large volumes of structured and unstructured data generated from various sources, including sensors, social media, mobile devices, and the internet. This abundance of data enabled AI researchers to train more complex and accurate models, uncover hidden patterns and insights, and make more informed decisions across a wide range of applications.
Big data had a profound impact on AI development in several key areas:
- Training Data: Big data provided AI algorithms with access to massive amounts of training data, allowing them to learn from examples and improve their performance over time. This data-driven approach enabled AI systems to achieve state-of-the-art results in tasks such as image recognition, speech recognition, and natural language processing.
- Scalability: The scalability of AI algorithms became increasingly important in the era of big data. Researchers developed distributed computing frameworks and parallel processing techniques to train and deploy AI models on large-scale datasets efficiently. These advancements facilitated the development of AI systems capable of handling the velocity, volume, and variety of big data.
- Personalization: Big data fueled the rise of personalized AI applications tailored to individual preferences and behaviors. By analyzing vast amounts of user data, AI systems could deliver personalized recommendations, content, and services in areas such as e-commerce, entertainment, and social media.
Advances in Natural Language Processing (NLP)
Natural language processing (NLP) saw significant advancements in the early 2000s, driven by the availability of large text corpora and breakthroughs in machine learning algorithms. NLP refers to the branch of AI that focuses on understanding, interpreting, and generating human language in a meaningful way.
Key advancements in NLP during this period included:
- Statistical Models: The adoption of statistical models and probabilistic methods revolutionized NLP, enabling AI systems to learn the structure and semantics of natural language from data. Techniques such as hidden Markov models (HMMs), conditional random fields (CRFs), and probabilistic graphical models (PGMs) became standard tools for NLP tasks such as part-of-speech tagging, named entity recognition, and syntactic parsing.
- Machine Translation: Machine translation systems experienced significant improvements in accuracy and fluency, thanks to advances in statistical machine translation (SMT) and neural machine translation (NMT). These approaches leveraged large bilingual corpora and neural network architectures to achieve human-level performance in translating text between different languages.
- Question Answering and Information Retrieval: NLP techniques were applied to develop question answering systems and information retrieval engines capable of extracting relevant information from large text collections. These systems enabled users to pose queries in natural language and receive accurate and concise answers from unstructured text data.
The early 2000s marked a pivotal moment in AI history, characterized by the transformative impact of big data and advanced algorithms on AI development. The synergy between big data and AI, coupled with breakthroughs in NLP, paved the way for a new era of intelligent systems capable of understanding and processing human language at scale.
2010s: Deep Learning Revolution
The 2010s witnessed a groundbreaking revolution in artificial intelligence (AI) driven by the widespread adoption of deep learning techniques. Deep learning, a subset of machine learning inspired by the structure and function of the human brain, leverages neural networks with multiple layers of interconnected nodes to learn hierarchical representations of data. This decade saw significant advancements in deep learning algorithms, fueled by the availability of large-scale datasets, powerful computing infrastructure, and breakthroughs in hardware acceleration.
Convolutional Neural Networks (CNNs) and Image Recognition
Convolutional Neural Networks (CNNs) emerged as a cornerstone of the deep learning revolution, revolutionizing the field of image recognition and computer vision. CNNs are specialized neural network architectures designed to process visual data by hierarchically extracting features from raw pixel inputs. They consist of multiple layers, including convolutional layers, pooling layers, and fully connected layers, which enable them to learn spatial hierarchies of features from images.
CNNs have achieved remarkable success in various image-related tasks, including:
- Image Classification: CNNs can classify images into predefined categories with unprecedented accuracy, surpassing human performance in some cases. Models like AlexNet, VGGNet, and ResNet have demonstrated state-of-the-art results on benchmark image classification datasets such as ImageNet.
- Object Detection: CNNs enable the detection and localization of objects within images by predicting bounding boxes and class labels for each object instance. Frameworks like Faster R-CNN, YOLO (You Only Look Once), and SSD (Single Shot MultiBox Detector) have significantly advanced object detection capabilities in real-world applications.
- Semantic Segmentation: CNNs can segment images into pixel-level regions corresponding to different object classes or semantic categories. Techniques such as Fully Convolutional Networks (FCNs) and U-Net have been instrumental in tasks such as medical image analysis, autonomous driving, and scene understanding.
Recurrent Neural Networks (RNNs) and Language Models
Recurrent Neural Networks (RNNs) emerged as another critical component of the deep learning revolution, revolutionizing the field of natural language processing (NLP) and sequential data analysis. RNNs are specialized neural network architectures designed to process sequential data by maintaining internal state or memory across time steps. They are well-suited for tasks involving sequential dependencies and temporal dynamics.
RNNs have been applied to various language-related tasks, including:
- Language Modeling: RNNs can learn the underlying structure and patterns of natural language by predicting the probability distribution of the next word in a sequence given previous words. Language models based on RNNs, such as Long Short-Term Memory (LSTM) networks and Gated Recurrent Units (GRUs), have achieved state-of-the-art performance in tasks such as text generation, machine translation, and speech recognition.
- Sequence-to-Sequence Learning: RNNs enable the mapping of input sequences to output sequences of variable lengths, making them well-suited for tasks such as machine translation, speech recognition, and summarization. Sequence-to-sequence models based on RNNs, such as Encoder-Decoder architectures, have become the de facto approach for many NLP applications.
- Sentiment Analysis and Text Classification: RNNs can analyze and classify textual data based on sentiment or topic, enabling applications such as sentiment analysis, document classification, and spam detection. RNN-based models have been widely used in social media analytics, customer feedback analysis, and content moderation.
The deep learning revolution of the 2010s, driven by breakthroughs in CNNs and RNNs, transformed the landscape of artificial intelligence and catalyzed unprecedented advancements in computer vision, natural language processing, and many other fields. These revolutionary techniques have paved the way for a new era of intelligent systems capable of understanding and processing complex data modalities with human-like accuracy and efficiency.
Contemporary AI Applications
AI in Healthcare
Artificial intelligence (AI) is revolutionizing the healthcare industry by offering innovative solutions to complex challenges, ranging from diagnosis and treatment to drug discovery and personalized medicine. The integration of AI technologies into healthcare systems holds the promise of improving patient outcomes, reducing healthcare costs, and enhancing overall efficiency.
Here you can read more about How AI is Transforming Healthcare
Diagnostic Systems and Personalized Medicine
AI-powered diagnostic systems are transforming the way diseases are detected and diagnosed. These systems leverage machine learning algorithms to analyze medical images, electronic health records (EHRs), genomic data, and other patient information to assist healthcare professionals in making accurate and timely diagnoses.
Personalized medicine, enabled by AI, aims to tailor medical treatments and interventions to individual patients based on their unique genetic makeup, medical history, and lifestyle factors. AI algorithms analyze vast amounts of patient data to identify patterns and correlations that can inform personalized treatment plans, drug prescriptions, and disease management strategies. By providing targeted therapies and interventions, personalized medicine maximizes treatment efficacy while minimizing adverse effects, leading to better patient outcomes.
AI in Drug Discovery and Development
AI is revolutionizing the drug discovery and development process by accelerating the identification, design, and optimization of novel therapeutic compounds. AI algorithms analyze biological data, chemical structures, and clinical trial data to predict drug candidates with the highest likelihood of success. These algorithms can identify potential drug targets, predict drug-drug interactions, and optimize drug formulations, significantly reducing the time and cost associated with traditional drug discovery methods.
Furthermore, AI enables the repurposing of existing drugs for new indications and the development of precision therapies for rare diseases and unmet medical needs. By leveraging AI-driven approaches such as virtual screening, molecular docking, and deep learning-based drug design, researchers can expedite the development of breakthrough treatments for a wide range of diseases, including cancer, neurodegenerative disorders, and infectious diseases.
AI in Finance
Artificial intelligence (AI) is revolutionizing the finance industry by providing advanced solutions for algorithmic trading, risk management, fraud detection, and more. The integration of AI technologies into financial systems has led to increased efficiency, improved decision-making, and enhanced security.
Algorithmic Trading and Risk Management
AI-powered algorithmic trading systems leverage machine learning algorithms to analyze vast amounts of financial data, identify patterns, and execute trades with speed and precision. These systems can automatically execute trades based on predefined strategies, taking into account market conditions, historical data, and risk factors.
Algorithmic trading algorithms can adapt to changing market conditions in real-time, allowing financial institutions to capitalize on market opportunities and mitigate risks more effectively. By automating trading processes and optimizing portfolio management strategies, AI enables financial firms to achieve higher returns while minimizing transaction costs and market volatility.
Risk management is another area where AI plays a crucial role in the finance industry. AI-powered risk management systems analyze complex financial data to assess and mitigate various types of risks, including market risk, credit risk, operational risk, and liquidity risk. These systems employ sophisticated algorithms to monitor financial markets, identify potential threats, and implement risk mitigation strategies in real-time.
Fraud Detection and Prevention
AI-powered fraud detection systems are instrumental in protecting financial institutions and consumers from fraudulent activities and cyber threats. These systems leverage machine learning algorithms to analyze transaction data, user behavior patterns, and other indicators of fraudulent activity.
By continuously monitoring financial transactions and detecting suspicious patterns or anomalies, AI-powered fraud detection systems can identify potential fraudsters and fraudulent transactions in real-time. These systems employ advanced techniques such as anomaly detection, pattern recognition, and predictive modeling to distinguish legitimate transactions from fraudulent ones.
Furthermore, AI enables financial institutions to implement proactive measures for fraud prevention, such as biometric authentication, multi-factor authentication, and real-time transaction monitoring. By leveraging AI-driven solutions, financial institutions can detect and prevent fraud more effectively, safeguarding their assets and maintaining trust with customers.
Here You can Read More about AI in Finance Industry
AI in Transportation
Artificial intelligence (AI) is revolutionizing the transportation industry by offering innovative solutions to enhance safety, efficiency, and sustainability. From autonomous vehicles and navigation systems to traffic management and smart cities, AI technologies are driving significant advancements in how people and goods are transported.
Autonomous Vehicles and Navigation Systems
Autonomous vehicles represent one of the most transformative applications of AI in transportation. These self-driving cars, trucks, and drones leverage AI algorithms, such as machine learning and computer vision, to perceive their surroundings, interpret traffic conditions, and make real-time driving decisions without human intervention.
AI-powered navigation systems play a crucial role in enabling autonomous vehicles to navigate complex environments and reach their destinations safely and efficiently. These systems integrate data from various sensors, including cameras, LiDAR, and radar, to create detailed maps, localize the vehicle within its environment, and plan optimal routes based on traffic conditions and user preferences.
Traffic Management and Smart Cities
AI technologies are also revolutionizing traffic management and urban mobility by optimizing traffic flow, reducing congestion, and improving overall transportation efficiency. AI-powered traffic management systems analyze real-time traffic data from sensors, cameras, and GPS devices to predict traffic patterns, identify congestion hotspots, and dynamically adjust traffic signals and routes to alleviate congestion and improve traffic flow.
Smart cities leverage AI and Internet of Things (IoT) technologies to create interconnected transportation systems that enhance mobility, sustainability, and quality of life for residents. AI-enabled transportation solutions, such as intelligent traffic lights, dynamic routing algorithms, and predictive maintenance systems, enable cities to optimize transportation networks, reduce environmental impact, and enhance the overall urban experience.
Ethical and Societal Implications
Ethical Considerations
As artificial intelligence (AI) continues to permeate various aspects of society, it is essential to address the ethical considerations associated with its development and deployment. Ethical considerations in AI encompass a broad range of issues, including bias and fairness, privacy concerns, data security, accountability, transparency, and societal impact.
AI Bias and Fairness
One of the most significant ethical challenges in AI is the presence of bias and fairness issues in AI systems. AI algorithms learn from historical data, which may reflect societal biases and inequalities. If not properly addressed, these biases can perpetuate discrimination and injustice, leading to unfair treatment and outcomes for certain individuals or groups.
Addressing AI bias and promoting fairness requires careful consideration and proactive measures at every stage of the AI lifecycle, from data collection and preprocessing to algorithm development and deployment. Strategies for mitigating bias include diverse and representative dataset collection, fairness-aware algorithm design, bias detection and monitoring, and stakeholder engagement and oversight.
Privacy Concerns and Data Security
Privacy concerns and data security represent another critical ethical consideration in AI. AI systems often rely on large amounts of personal data to train models and make predictions, raising concerns about data privacy, consent, and the potential for misuse or unauthorized access.
Protecting privacy and ensuring data security in AI systems requires robust data governance frameworks, encryption techniques, access controls, and compliance with relevant regulations such as the General Data Protection Regulation (GDPR) and the Health Insurance Portability and Accountability Act (HIPAA). Additionally, transparency and accountability mechanisms, such as data anonymization, privacy impact assessments, and algorithm explainability, can help build trust and confidence in AI systems among users and stakeholders.
Societal Impact
The widespread adoption of artificial intelligence (AI) is transforming society in profound and multifaceted ways, shaping how we work, communicate, learn, and interact with the world around us. As AI technologies continue to evolve and permeate various sectors, it is crucial to consider their societal impact and implications for individuals, communities, and societies at large.
Employment and Job Displacement
One of the most pressing societal impacts of AI is its potential to disrupt labor markets and lead to job displacement. AI technologies have the capacity to automate repetitive tasks, augment human capabilities, and even perform complex cognitive functions traditionally performed by humans. While AI has the potential to create new job opportunities and enhance productivity, it also raises concerns about the future of work and the displacement of certain jobs and industries.
Addressing the challenges of AI-induced job displacement requires proactive measures to reskill and upskill the workforce, promote lifelong learning and adaptability, and ensure inclusive and equitable access to opportunities in the digital economy. Additionally, policies and initiatives aimed at fostering job creation, supporting affected workers, and promoting economic resilience are essential for mitigating the negative effects of AI on employment and ensuring a smooth transition to the future of work.
The Future of Human-AI Collaboration
Despite concerns about job displacement, AI also offers significant opportunities for human-AI collaboration and augmentation. Rather than replacing humans, AI technologies have the potential to complement and enhance human capabilities, enabling us to tackle complex challenges, make more informed decisions, and achieve greater levels of productivity and innovation.
The future of human-AI collaboration lies in leveraging the unique strengths of both humans and machines to achieve shared goals and outcomes. AI technologies can assist humans in performing tasks more efficiently, providing valuable insights, and automating routine processes, while humans contribute creativity, critical thinking, emotional intelligence, and ethical judgment to AI systems.
By fostering a culture of collaboration, trust, and partnership between humans and AI, we can harness the full potential of AI technologies to address pressing societal challenges, drive economic growth, and improve quality of life for individuals and communities. Embracing human-AI collaboration as a cornerstone of our approach to AI development and deployment can help us navigate the complexities of the digital age and shape a future where humans and machines work together synergistically to create a more prosperous and inclusive society.
The Future of AI
Emerging Trends
As artificial intelligence (AI) continues to evolve and mature, several emerging trends are shaping the trajectory of AI research and development. These trends reflect new opportunities, challenges, and advancements in AI technologies and applications, paving the way for transformative changes in various industries and domains.
Explainable AI and Transparency
Explainable AI (XAI) has emerged as a critical trend in AI research and development, driven by the growing demand for transparency, accountability, and trustworthiness in AI systems. XAI aims to enhance the interpretability and explainability of AI models and decisions, enabling users to understand how AI systems arrive at their predictions or recommendations.
By providing insights into the inner workings of AI algorithms and the factors influencing their outputs, XAI techniques help stakeholders, including end-users, regulators, and domain experts, make informed decisions, diagnose errors or biases, and identify opportunities for improvement. XAI encompasses a variety of approaches, including model-agnostic methods, feature importance analysis, attention mechanisms, and counterfactual explanations, tailored to different AI applications and use cases.
AI in Quantum Computing
The intersection of AI and quantum computing represents another emerging trend with significant implications for the future of AI research and applications. Quantum computing, a revolutionary paradigm of computation leveraging the principles of quantum mechanics, offers unprecedented computational power and capabilities, surpassing the limitations of classical computing.
AI in quantum computing explores the synergy between AI algorithms and quantum computing hardware, aiming to accelerate AI computations, optimize AI models, and tackle computationally intensive tasks beyond the capabilities of classical computers. Quantum machine learning, quantum neural networks, and quantum optimization algorithms are among the key areas of research in AI and quantum computing, with potential applications in fields such as drug discovery, materials science, cryptography, and optimization problems.
As researchers continue to explore the potential of AI in quantum computing, collaborations between AI and quantum computing experts are expected to drive innovation and unlock new frontiers in AI research and applications. By harnessing the power of quantum computing to enhance AI capabilities, we can address complex challenges, solve previously intractable problems, and unlock new opportunities for scientific discovery and technological advancement.
Challenges Ahead
As artificial intelligence (AI) technologies continue to advance and proliferate across various domains, several challenges lie ahead that must be addressed to realize the full potential of AI while mitigating risks and ensuring responsible development and deployment.
Regulatory and Policy Challenges
One of the foremost challenges facing the AI landscape is the development of comprehensive regulatory frameworks and policies to govern the ethical use and deployment of AI technologies. As AI applications become increasingly integrated into critical systems and decision-making processes, there is a pressing need for regulations that address issues such as data privacy, algorithmic transparency, accountability, bias mitigation, and societal impact.
Effective AI regulations and policies require interdisciplinary collaboration among policymakers, technologists, ethicists, and stakeholders from industry, academia, and civil society. Regulatory approaches should strike a balance between fostering innovation and ensuring public trust and safety, taking into account the unique characteristics and challenges of AI technologies and applications.
Ensuring Ethical AI Development
Ethical considerations are central to the responsible development and deployment of AI technologies. Ensuring that AI systems are designed, implemented, and used in accordance with ethical principles and values is essential for safeguarding individual rights, promoting fairness and equity, and preventing harm.
Key ethical considerations in AI development include transparency, accountability, fairness, privacy, and societal impact. Developers and stakeholders must prioritize ethical considerations throughout the AI lifecycle, from data collection and algorithm design to deployment and evaluation. This requires adopting ethical AI frameworks, conducting ethical impact assessments, promoting diversity and inclusivity in AI development teams, and engaging with stakeholders to understand and address ethical concerns.
Summary
Artificial intelligence (AI) has undergone a remarkable evolution since its inception, transforming from a theoretical concept to a powerful force driving innovation across diverse industries and domains. The journey of AI has been characterized by breakthroughs, challenges, and paradigm shifts, shaping the way we live, work, and interact with technology.
Recap of Evolution of Artificial Intelligence
Evolution of artificial intelligence can be traced back to its conceptual foundations in ancient myths and philosophical inquiries, through the pioneering work of visionaries such as Alan Turing and John McCarthy in the mid-20th century. The birth of AI in the 1950s and 1960s marked the advent of early computer programs and symbolic AI systems, followed by the AI Winter of the 1970s, which brought challenges and setbacks to the field.
The emergence of expert systems in the 1980s laid the groundwork for the AI boom of the 1990s, characterized by the rise of machine learning and neural networks. The early 2000s saw the convergence of big data and advanced algorithms, leading to breakthroughs in natural language processing and image recognition. The 2010s witnessed the deep learning revolution, fueled by advancements in convolutional neural networks (CNNs) and recurrent neural networks (RNNs), as well as the proliferation of AI in various sectors, including healthcare, finance, transportation, and more.
The Continuing Journey of AI
As we look to the future, the journey of AI continues, with new opportunities, challenges, and frontiers awaiting exploration. Emerging trends such as explainable AI, quantum computing, and human-AI collaboration offer promise for further advancements in AI research and applications. However, challenges such as regulatory and policy considerations, ethical dilemmas, and societal impacts must be addressed to ensure the responsible and ethical development and deployment of AI technologies.
The continuing journey of AI requires collaboration, innovation, and a commitment to ethical principles and values. By working together to navigate the complexities of AI, we can unlock its full potential to drive positive change, solve pressing challenges, and create a better future for humanity.