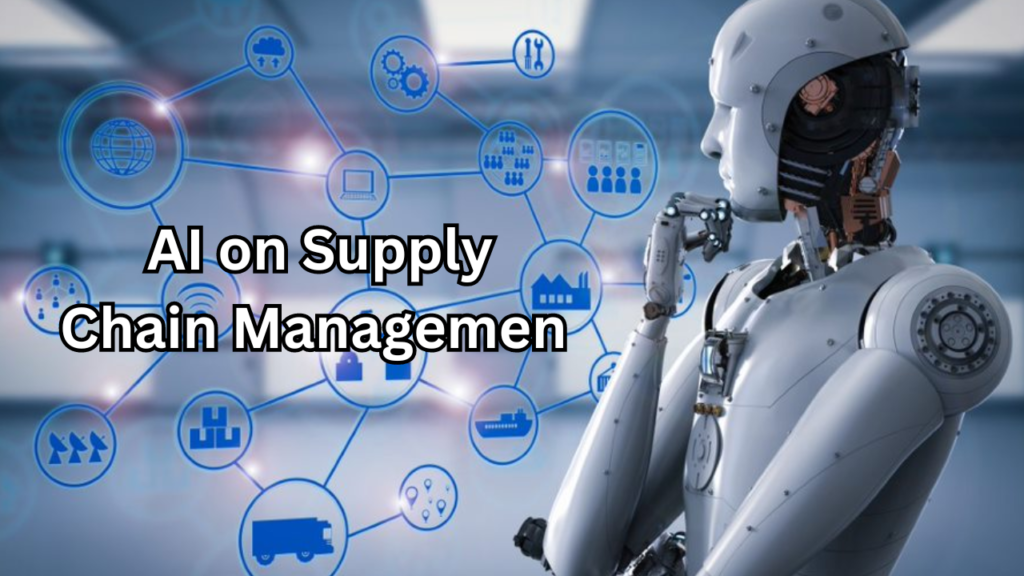
Table of Contents
Introduction
In today’s interconnected global economy, the effective management of supply chains plays a pivotal role in the success and competitiveness of businesses across industries. With the rapid advancements in technology, particularly in artificial intelligence (AI), the landscape of AI on supply chain management (SCM) is undergoing a transformative evolution. This evolution is not just about improving operational efficiency but also about redefining how businesses meet customer demands, manage resources, and mitigate risks.
Definition of AI on Supply Chain Management (SCM)
AI on supply chain management (SCM) encompasses the planning, execution, and control of all activities involved in sourcing, procurement, production, logistics, and distribution processes. It involves the coordination and integration of these activities across various entities, including suppliers, manufacturers, wholesalers, retailers, and end customers. The goal of SCM is to ensure that products or services are delivered to the right place, at the right time, and in the right quantity, while optimizing costs and maintaining quality standards throughout the entire supply chain network.
Importance of Optimizing SCM
Optimizing SCM is crucial for several reasons:
- Cost Efficiency: Efficient SCM helps in reducing operational costs by streamlining processes, minimizing waste, and optimizing inventory levels.
- Enhanced Customer Satisfaction: By ensuring timely delivery and availability of products, SCM contributes to better customer service and satisfaction.
- Risk Mitigation: Effective SCM strategies mitigate risks associated with supply chain disruptions, such as natural disasters, political instability, or unexpected demand fluctuations.
- Competitive Advantage: Organizations that can effectively manage their supply chains gain a competitive edge by responding quickly to market changes, innovating faster, and adapting to customer preferences.
- Sustainability: Optimized SCM practices can contribute to sustainability goals by reducing carbon footprints through efficient logistics and minimizing waste in the supply chain processes.
In essence, optimizing SCM through advanced technologies like AI not only enhances operational efficiencies but also empowers businesses to meet the dynamic demands of today’s marketplace effectively.
Role of AI in Supply Chain Management
Artificial Intelligence AI on supply chain management (SCM) by introducing advanced capabilities that enhance decision-making, optimize processes, and improve overall efficiency throughout the supply chain network. Key areas where AI is making a significant impact include data analytics, predictive modeling, demand forecasting, and inventory optimization.
Data Analytics and Predictive Modeling
AI enables robust data analytics and predictive modeling capabilities in SCM by processing vast amounts of data from various sources, including historical sales data, customer trends, market conditions, and even external factors like weather patterns and geopolitical events. This enables:
- Insight Generation: AI algorithms can uncover hidden patterns and correlations within data that human analysts may overlook, providing valuable insights into customer behavior, market trends, and supply chain performance.
- Predictive Analytics: By leveraging machine learning algorithms, AI can predict future demand patterns more accurately than traditional methods. This allows organizations to anticipate demand fluctuations, seasonal trends, and even unexpected changes, enabling proactive decision-making in inventory management and production planning.
Demand Forecasting
Demand forecasting is a critical aspect of SCM, as it directly impacts inventory levels, production schedules, and distribution strategies. AI enhances demand forecasting by:
- Real-time Analysis: AI algorithms can continuously analyze incoming data streams, such as point-of-sale data, social media trends, and economic indicators, to adjust forecasts in real-time.
- Accuracy Improvement: Machine learning algorithms improve forecast accuracy over time by learning from historical data and adjusting predictions based on changing market conditions and customer behavior.
- Scenario Planning: AI enables scenario-based forecasting, allowing organizations to simulate different market scenarios and assess the impact on demand, enabling better risk management and strategic decision-making.
Inventory Optimization
Optimizing inventory levels is crucial for balancing supply and demand, reducing carrying costs, and ensuring product availability. AI plays a pivotal role in inventory optimization by:
- Dynamic Inventory Management: AI algorithms can dynamically adjust inventory levels based on real-time demand signals, supplier lead times, and production capacities, optimizing stock levels to minimize stockouts and excess inventory.
- Supply Chain Visibility: AI-driven analytics provide real-time visibility into inventory across the supply chain, enabling organizations to synchronize inventory levels across warehouses and distribution centers, reducing fulfillment costs and improving order fulfillment rates.
- Supplier Collaboration: AI facilitates collaboration with suppliers by predicting demand patterns and sharing forecasts, enabling proactive supply chain management and reducing lead times.
In conclusion, AI-driven data analytics, predictive modeling, demand forecasting, and inventory optimization are transforming supply chain management by enabling organizations to operate more efficiently, respond quickly to market changes, and deliver superior customer experiences. As AI continues to evolve, its role in SCM will likely expand, offering new opportunities for innovation and competitive advantage in an increasingly complex global marketplace.
Automation and Robotics in Supply Chain Management
Automation and robotics are revolutionizing supply chain management (SCM) by introducing advanced technologies that streamline operations, improve efficiency, and enhance overall productivity across various stages of the supply chain network.
Warehouse Management
Warehouse management is a critical component of SCM, where automation and robotics play a transformative role:
- Automated Storage and Retrieval Systems (AS/RS): AS/RS systems use robotics to automatically store and retrieve goods from designated storage locations within warehouses. This technology reduces labor costs, minimizes errors, and optimizes space utilization.
- Pick-and-Place Robotics: Robotics are used for picking, packing, and sorting goods in warehouses. Automated guided vehicles (AGVs) and autonomous mobile robots (AMRs) navigate through warehouse aisles, transporting goods between storage areas and shipping docks efficiently.
- Inventory Tracking: RFID (Radio Frequency Identification) and barcode scanning technologies, often integrated with robotic systems, enable real-time inventory tracking and management, ensuring accurate stock levels and reducing the risk of stockouts.
Autonomous Vehicles
Autonomous vehicles are reshaping logistics and transportation within supply chains:
- Autonomous Trucks: Self-driving trucks equipped with AI and sensors can autonomously navigate highways and local roads, delivering goods between distribution centers and retail locations. These vehicles enhance delivery efficiency, reduce fuel consumption, and improve road safety.
- Drones: Unmanned aerial vehicles (drones) are used for inventory management, warehouse inspection, and last-mile delivery. Drones can access hard-to-reach areas in warehouses and deliver small parcels directly to customers’ doorsteps, reducing delivery times and operational costs.
- Autonomous Forklifts and AGVs: Within warehouses and manufacturing facilities, autonomous forklifts and AGVs transport materials and goods between production lines, storage areas, and shipping docks without human intervention. This automation increases operational efficiency and reduces manual labor requirements.
Benefits of Automation and Robotics
The integration of automation and robotics in SCM offers several benefits:
- Improved Efficiency: Automated processes reduce cycle times, increase throughput, and minimize errors, enhancing overall operational efficiency.
- Cost Savings: Reduced labor costs, optimized inventory management, and lower transportation expenses contribute to significant cost savings for businesses.
- Enhanced Safety: Robotics and autonomous vehicles improve workplace safety by minimizing the risks associated with manual handling and transportation of goods.
- Scalability: Scalable automation solutions allow businesses to adapt quickly to changing market demands and scale operations without significant infrastructure investments.
AI in Logistics and Transportation
Artificial Intelligence (AI) is reshaping logistics and transportation sectors by leveraging advanced algorithms and data analytics to optimize operations, improve efficiency, and enhance overall customer satisfaction.
Route Optimization
Route optimization is crucial for minimizing transportation costs, reducing delivery times, and maximizing vehicle utilization:
- AI Algorithms: AI algorithms analyze historical data, real-time traffic conditions, weather forecasts, and delivery constraints to generate optimized routes for vehicles. These algorithms continuously learn and adapt to changing conditions, ensuring efficient route planning.
- Dynamic Adjustments: AI enables real-time adjustments to routes based on traffic congestion, road closures, and other unforeseen events, ensuring on-time deliveries and reducing fuel consumption.
- Multi-Objective Optimization: AI can optimize routes based on multiple objectives, such as minimizing fuel costs, reducing carbon emissions, and maximizing delivery efficiency, while considering constraints like delivery windows and vehicle capacities.
Last-Mile Delivery
Last-mile delivery is the final and often most challenging leg of the supply chain, involving the transportation of goods from distribution centers to customers’ doorsteps:
- Predictive Analytics: AI-powered predictive analytics forecast demand patterns and consumer behavior, enabling companies to pre-position inventory closer to customers, reducing last-mile delivery times and costs.
- Delivery Route Optimization: AI optimizes last-mile delivery routes by considering factors such as traffic patterns, delivery windows, package sizes, and customer preferences, ensuring efficient and timely deliveries.
- Autonomous Vehicles and Drones: AI-driven autonomous vehicles and drones are increasingly used for last-mile delivery. These technologies navigate urban environments and deliver parcels directly to customers’ homes or designated pickup points, enhancing delivery speed and customer convenience.
Benefits of AI in Supply Chain Management (SCM)
Artificial Intelligence AI on supply chain management (SCM) by introducing advanced capabilities that enhance efficiency, decision-making processes, and risk management strategies.
Improved Efficiency and Cost Reduction
AI improves efficiency in SCM through several mechanisms:
- Automation of Routine Tasks: AI automates repetitive tasks such as data entry, inventory management, and order processing, reducing human error and accelerating operational workflows.
- Optimized Resource Allocation: AI algorithms analyze data to optimize resource allocation, including workforce scheduling, transportation routes, and inventory levels. This optimization minimizes idle resources and reduces overall operational costs.
- Predictive Maintenance: AI-powered predictive analytics anticipate equipment failures and maintenance needs, enabling proactive maintenance interventions that prevent costly downtime and disruptions in production.
Enhanced Decision Making
AI enhances decision-making processes in SCM by:
- Real-time Insights: AI analyzes large volumes of data in real-time to provide actionable insights into market trends, customer preferences, and supply chain performance metrics. This enables businesses to make informed decisions quickly and effectively.
- Scenario Analysis: AI models simulate various scenarios based on different variables and constraints, allowing organizations to evaluate the impact of decisions on operational outcomes before implementation.
- Prescriptive Analytics: AI-powered prescriptive analytics recommend optimal actions and strategies to achieve specific supply chain goals, such as minimizing costs, maximizing efficiency, or improving service levels.
Challenges and Limitations of AI in Supply Chain Management (SCM)
While AI offers substantial benefits in optimizing AI on supply chain management, its implementation is not without challenges and limitations that organizations must navigate.
Data Quality and Integration Issues
- Data Complexity: SCM generates vast amounts of data from various sources, including suppliers, manufacturers, logistics partners, and customers. Ensuring data accuracy, completeness, and consistency poses challenges due to disparate data formats and quality issues.
- Integration Challenges: Integrating data from legacy systems, IoT devices, and external sources into AI platforms requires robust data integration frameworks and interoperability standards. Poor data integration can lead to inaccurate insights and decision-making.
- Data Privacy and Security: Maintaining data privacy and security standards while sharing sensitive supply chain data with AI systems poses regulatory compliance challenges and risks of data breaches.
Implementation Costs and Complexity
- Infrastructure Investment: Deploying AI technologies in SCM requires significant upfront investments in hardware, software, and IT infrastructure capable of supporting advanced analytics, AI algorithms, and cloud computing resources.
- Skill Gap: Acquiring and retaining talent with expertise in AI, data science, and SCM is a challenge. Organizations may face difficulties in finding skilled professionals capable of developing, implementing, and maintaining AI solutions.
- Complexity in Deployment: Integrating AI systems into existing SCM processes and workflows involves complex project management, change management, and stakeholder alignment efforts. Overcoming organizational inertia and resistance to change can delay implementation timelines.
Workforce Adaptation
- Skill Development: AI adoption in SCM necessitates reskilling and upskilling the workforce to leverage AI tools effectively. Employees need training in data analysis, AI-driven decision-making, and collaboration with automated systems.
- Job Displacement Concerns: Automation and AI-driven efficiencies may lead to workforce displacement in traditional SCM roles, such as manual labor in warehouses or routine administrative tasks. Organizations must manage workforce transitions through retraining programs and job redeployment initiatives.
- Cultural Shift: Embracing AI in SCM requires a cultural shift towards data-driven decision-making, agility, and continuous learning within the organization. Building a culture of innovation and embracing technological change is essential for successful AI adoption.
Case Studies and Examples of AI in Supply Chain Management
AI has been instrumental in transforming supply chain operations for major corporations like Amazon and Walmart, showcasing innovative applications that enhance efficiency, reduce costs, and improve customer satisfaction.
Amazon’s Use of AI in Fulfillment Centers
Amazon, a pioneer in e-commerce and logistics, leverages AI extensively in its fulfillment centers to streamline operations and meet growing customer demands:
- Robotics and Automation: Amazon utilizes robots powered by AI to automate order picking, packing, and sorting processes in fulfillment centers. These robots navigate autonomously through warehouses, retrieving items and delivering them to human workers for packaging.
- Predictive Analytics: Amazon employs AI-driven predictive analytics to forecast customer demand with high accuracy. By analyzing historical sales data, seasonal trends, and other variables, Amazon optimizes inventory management, ensuring products are stocked efficiently to meet customer orders without overstocking.
- Delivery Optimization: AI algorithms optimize delivery routes for Amazon’s logistics network, minimizing delivery times and costs. This includes leveraging real-time traffic data, weather conditions, and historical delivery patterns to determine the most efficient routes for package delivery.
- Customer Personalization: AI algorithms analyze customer preferences, browsing history, and purchase behavior to personalize recommendations and promotions. This enhances the customer shopping experience and increases order fulfillment accuracy.
AI-driven Supply Chain Optimization by Walmart
Walmart, one of the world’s largest retailers, harnesses AI to optimize its supply chain and logistics operations:
- Inventory Management: Walmart uses AI-powered algorithms to manage inventory across its network of stores and distribution centers. By analyzing sales data, supplier lead times, and seasonal demand fluctuations, Walmart optimizes inventory levels to prevent stockouts while minimizing excess inventory.
- Supply Chain Visibility: AI enhances Walmart’s supply chain visibility by tracking inventory in real-time and identifying potential disruptions early. This allows Walmart to proactively manage supplier relationships and mitigate risks associated with supply chain disruptions.
- Demand Forecasting: Walmart employs AI-driven demand forecasting models to predict customer demand at different store locations. These models consider factors such as local economic conditions, demographic trends, and promotional activities to optimize stocking levels and improve sales forecasting accuracy.
- Sustainability Initiatives: AI helps Walmart in its sustainability efforts by optimizing transportation routes to reduce fuel consumption and carbon emissions. This contributes to Walmart’s goal of operating a more sustainable supply chain.
Future Trends in AI in Supply Chain Management
The future of AI in supply chain management (SCM) holds promising advancements that will continue to reshape operations, enhance efficiency, and address emerging challenges in global supply chains.
Advancements in AI and Machine Learning
- Advanced Predictive Analytics: Future AI systems will leverage more sophisticated machine learning algorithms to improve predictive accuracy in demand forecasting, inventory management, and supply chain optimization. This includes the use of deep learning techniques for pattern recognition and anomaly detection.
- Autonomous Decision-Making: AI-driven autonomous decision-making systems will enable SCM processes to operate more autonomously, from autonomous vehicles in transportation to automated inventory replenishment and supply chain orchestration.
- Natural Language Processing (NLP) and AI Assistants: AI-powered chatbots and virtual assistants equipped with NLP capabilities will enhance communication and collaboration across supply chain stakeholders, improving responsiveness and operational agility.
Integration with IoT and Blockchain
- IoT-enabled Supply Chains: Integration of AI with IoT devices will enable real-time tracking of assets, monitoring of environmental conditions (e.g., temperature, humidity), and predictive maintenance of machinery and equipment within the supply chain.
- Blockchain for Transparency and Traceability: AI and blockchain integration will enhance transparency and traceability across supply chains by providing immutable records of transactions, provenance of goods, and compliance with ethical and regulatory standards. This can improve trust among stakeholders and mitigate risks related to counterfeit products and supply chain fraud.
Ethical and Regulatory Considerations
- Data Privacy and Security: As AI systems rely on vast amounts of data, ensuring data privacy and security remains a critical concern. Regulations such as GDPR (General Data Protection Regulation) in Europe and similar laws globally will influence how AI-driven SCM systems handle personal and sensitive data.
- Algorithmic Bias: AI algorithms can inadvertently perpetuate biases present in training data, affecting decisions related to pricing, hiring, and supplier selection. Addressing algorithmic bias requires careful data curation, algorithm transparency, and ethical guidelines in AI development.
- Labor Displacement and Workforce Impact: The adoption of AI in SCM may lead to job displacement in traditional roles, necessitating reskilling and upskilling initiatives for the workforce. Ethical considerations include ensuring fair treatment of workers affected by automation and promoting inclusive growth in the digital economy.
Conclusion
In conclusion, the transformative impact of Artificial Intelligence AI on supply chain management (SCM) has been profound and continues to reshape how businesses operate within global supply chains. AI technologies, including machine learning, predictive analytics, and automation, have revolutionized traditional SCM practices by enhancing efficiency, improving decision-making capabilities, and mitigating risks. From optimizing inventory management and streamlining logistics to enabling real-time data-driven insights, AI has empowered organizations to achieve greater operational agility and responsiveness to market dynamics.
Recap of AI’s Transformative Impact on SCM
AI has fundamentally altered SCM by optimizing processes that were previously labor-intensive and error-prone. Through advanced data analytics, AI systems can now forecast demand with unprecedented accuracy, leading to optimized inventory levels and reduced costs. Automation powered by AI has transformed warehouse operations and logistics, making them more efficient and adaptable to changing customer demands. Moreover, AI-driven decision-making has enabled organizations to make informed choices swiftly, enhancing overall supply chain resilience and customer satisfaction. These advancements underscore AI’s role as a catalyst for innovation in SCM, enabling businesses to navigate complexities and achieve sustainable growth in a competitive global marketplace.
Future Outlook and Recommendations
Looking ahead, the future of AI in SCM holds promising opportunities for further innovation and strategic integration. Organizations are encouraged to continue investing in AI technologies, such as IoT and blockchain, to enhance supply chain visibility, traceability, and sustainability. Embracing AI-driven predictive capabilities will enable proactive risk management and adaptive supply chain strategies. Furthermore, fostering a culture of continuous learning and digital transformation will be essential for equipping the workforce with the skills needed to leverage AI effectively in SCM. Collaborating with technology partners and regulatory bodies to address ethical considerations and compliance requirements will also be critical. By embracing these recommendations and leveraging AI’s transformative potential, organizations can position themselves for continued success and leadership in the dynamic landscape of modern supply chain management.