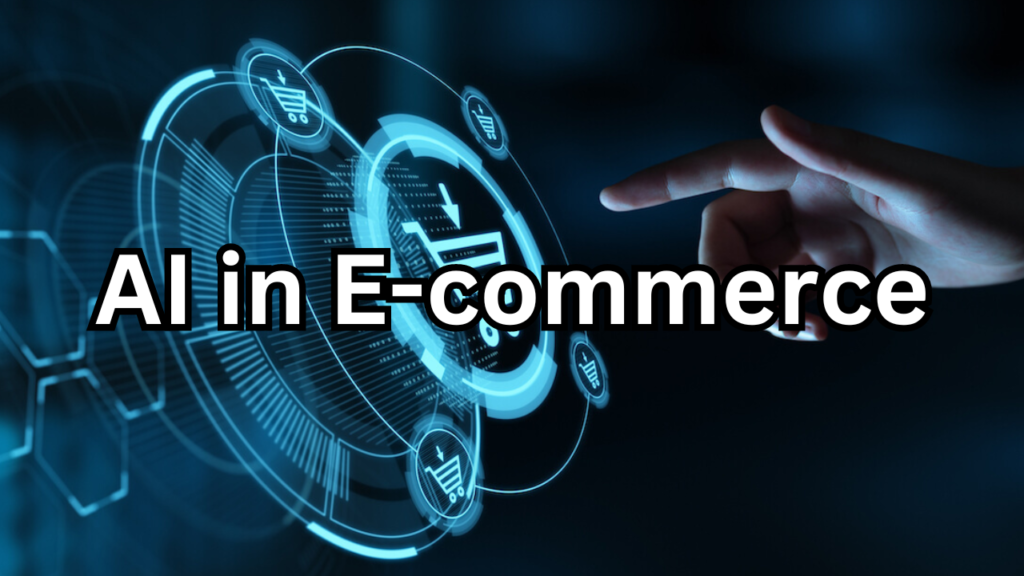
Table of Contents
Introduction to AI in E-commerce
Artificial Intelligence (AI) has emerged as a transformative force within the realm of e-commerce, revolutionizing how businesses interact with customers, manage operations, and drive growth. By leveraging advanced algorithms and machine learning techniques, AI enables e-commerce platforms to analyze vast amounts of data in real-time, offering unprecedented insights into consumer behavior and preferences. This technological innovation has not only enhanced the efficiency of online retail operations but has also personalized the shopping experience in ways previously unimaginable.
Definition and Scope
In the context of e-commerce, AI refers to the application of computational algorithms that simulate human intelligence processes such as learning, reasoning, and problem-solving. These algorithms allow e-commerce platforms to automate tasks, predict customer behavior, optimize pricing strategies, and personalize recommendations based on individual user preferences. The scope of AI in e-commerce extends across various domains, including customer service automation, supply chain management, marketing optimization, and fraud detection, thereby encompassing nearly every aspect of the online retail experience.
Importance of AI in Transforming E-commerce
The integration of AI into e-commerce operations is pivotal for businesses aiming to stay competitive in a rapidly evolving digital landscape. AI-driven technologies enable e-commerce enterprises to deliver seamless and personalized shopping experiences that foster customer loyalty and satisfaction. Moreover, AI enhances operational efficiency by streamlining processes, reducing costs, and improving decision-making through data-driven insights. As consumers increasingly expect personalized interactions and instant gratification, AI not only meets these expectations but also anticipates future trends and demands, positioning e-commerce businesses at the forefront of innovation and market leadership.
Foundational Concepts of AI
Machine Learning vs. AI
Machine Learning (ML) and Artificial Intelligence (AI) are closely related but distinct concepts within the realm of technology and computer science. AI is the broader field that encompasses any technique or system that enables machines to simulate human intelligence, such as reasoning, learning, problem-solving, and decision-making. Machine Learning, on the other hand, is a subset of AI focused on developing algorithms that allow computers to learn from and make predictions or decisions based on data. In essence, while AI aims to create intelligent systems that can mimic human cognitive functions across a wide range of tasks, Machine Learning provides the tools and techniques to achieve this by training models on large datasets.
Deep Learning and Neural Networks
Deep Learning is a specialized subset of Machine Learning that revolves around training artificial neural networks (ANNs) with many layers (hence “deep”) to learn from data representations. Neural Networks, inspired by the structure of the human brain, consist of interconnected nodes (neurons) organized in layers. Each layer processes information and passes it on to the next, with deeper layers capable of learning increasingly complex representations of data. Deep Learning techniques have revolutionized fields like image and speech recognition, natural language processing, and even e-commerce, where they enable more accurate predictions, personalized recommendations, and improved customer interactions based on vast amounts of data.
Natural Language Processing (NLP) in E-commerce
Natural Language Processing (NLP) refers to the field of AI focused on enabling machines to understand, interpret, and generate human language in a way that is both meaningful and contextually appropriate. In e-commerce, NLP plays a crucial role in enhancing customer interactions and improving operational efficiencies. NLP algorithms power chatbots and virtual assistants that can understand customer queries, provide real-time support, and even facilitate transactions. Additionally, NLP enables sentiment analysis of customer reviews and social media interactions, allowing e-commerce platforms to gauge customer satisfaction and adapt marketing strategies accordingly. By leveraging NLP, e-commerce businesses can automate processes, personalize communication, and ultimately enhance the overall shopping experience for their customers.
AI Applications in E-commerce
Personalized Shopping Experience
A personalized shopping experience in e-commerce refers to tailoring the online shopping journey to meet the individual preferences and behaviors of each customer. AI plays a pivotal role in achieving personalization by analyzing vast amounts of data, including browsing history, purchase patterns, demographics, and even real-time interactions. By leveraging machine learning algorithms, e-commerce platforms can recommend products that are highly relevant to each customer’s interests, suggest personalized promotions, and create dynamic website experiences that adapt in real-time. This level of personalization not only enhances customer satisfaction but also increases engagement and conversion rates, as customers feel understood and valued by the brand.
Product Recommendations and Upselling
Product recommendations and upselling techniques powered by AI are crucial strategies for increasing average order value and driving revenue in e-commerce. AI algorithms analyze customer behavior and purchase history to suggest complementary products or upgrades that align with the customer’s preferences. These recommendations are often displayed prominently on product pages, in cart summaries, or through personalized email campaigns. By intelligently upselling related items or higher-priced alternatives, e-commerce businesses can capitalize on cross-selling opportunities and enhance the overall shopping experience by making relevant suggestions that are likely to resonate with the customer.
Customer Service Automation
Customer service automation using AI technologies like chatbots and virtual assistants has revolutionized how e-commerce businesses handle customer inquiries and support requests. AI-powered chatbots can provide instant responses to common questions, assist with order tracking, initiate returns or exchanges, and even recommend products based on customer queries. By automating routine customer service tasks, businesses can significantly reduce response times, operate more efficiently at scale, and improve customer satisfaction by providing round-the-clock support. Moreover, AI-driven sentiment analysis allows businesses to prioritize and escalate issues that require human intervention, ensuring that customers receive timely and effective assistance throughout their shopping journey.
AI-driven Customer Insights
Sentiment Analysis in Customer Reviews
Sentiment analysis, a key application of natural language processing (NLP) in e-commerce, involves extracting and categorizing opinions expressed in customer reviews or feedback into positive, negative, or neutral sentiments. AI-powered sentiment analysis algorithms analyze textual data to gauge customer satisfaction, identify emerging trends, and pinpoint areas needing improvement. By automating this process, e-commerce businesses can swiftly identify and respond to customer sentiments, improving product offerings, enhancing service quality, and maintaining a positive brand reputation. Moreover, sentiment analysis provides valuable insights for marketing strategies, enabling businesses to craft targeted campaigns that resonate with their audience’s emotions and preferences.
Predictive Analytics for Customer Behavior
Predictive analytics leverages AI and machine learning algorithms to forecast future customer behavior based on historical data, patterns, and trends. In e-commerce, predictive analytics enables businesses to anticipate customer actions such as purchasing decisions, browsing patterns, and even potential churn. By analyzing factors such as demographics, past purchases, website interactions, and external variables like economic conditions or seasonal trends, businesses can personalize marketing efforts, optimize inventory management, and implement proactive customer retention strategies. This predictive capability empowers e-commerce businesses to stay ahead of market dynamics and respond swiftly to changing consumer preferences, thereby driving revenue growth and fostering long-term customer loyalty.
Customer Lifetime Value (CLV) Prediction
Customer Lifetime Value (CLV) prediction involves estimating the total revenue a customer is likely to generate throughout their relationship with a business. AI algorithms analyze historical data on customer purchases, interactions, and behaviors to calculate CLV, taking into account factors such as purchase frequency, average order value, retention rates, and acquisition costs. By predicting CLV, e-commerce businesses can prioritize resources and marketing efforts towards high-value customers, personalize customer experiences based on their predicted lifetime value, and optimize customer acquisition strategies to maximize overall profitability. CLV prediction also aids in segmenting customers effectively, identifying loyal customers for special retention initiatives, and improving overall business profitability through strategic decision-making rooted in data-driven insights.
AI in Supply Chain Management
Inventory Optimization
Inventory optimization in e-commerce involves strategically managing stock levels to meet customer demand while minimizing carrying costs and stockouts. AI plays a crucial role in inventory optimization by analyzing historical sales data, seasonal trends, market demand fluctuations, and even external factors like weather or promotions. AI algorithms can forecast demand accurately, identify optimal reorder points and quantities, and dynamically adjust inventory levels in real-time. By leveraging predictive analytics and machine learning, e-commerce businesses can reduce excess inventory, improve inventory turnover rates, and ensure product availability, thus enhancing customer satisfaction and operational efficiency.
Demand Forecasting using AI
Demand forecasting using AI enables e-commerce businesses to predict future customer demand for products or services with greater accuracy and reliability. AI algorithms analyze historical sales data, customer behavior patterns, market trends, and external factors to generate forecasts that guide inventory management, production planning, and marketing strategies. By leveraging machine learning models such as time series analysis, regression, and neural networks, businesses can anticipate seasonal spikes, respond to changing consumer preferences, and optimize pricing and promotional strategies accordingly. Accurate demand forecasting not only reduces stockouts and overstocking but also improves resource allocation, enhances supply chain efficiency, and ultimately boosts profitability in e-commerce operations.
Warehouse Automation
Warehouse automation refers to the use of AI-driven technologies and robotics to streamline and optimize warehouse operations in e-commerce fulfillment centers. AI-powered automation solutions encompass tasks such as inventory sorting, picking, packing, and shipping, significantly reducing manual labor and human error while improving speed and accuracy. AI algorithms can optimize warehouse layouts, route planning for goods movement, and even predict maintenance needs for machinery. By implementing warehouse automation, e-commerce businesses can achieve faster order fulfillment, reduce operational costs, enhance inventory management precision, and scale operations efficiently to meet growing customer demands. Moreover, warehouse automation improves workplace safety and allows human employees to focus on higher-value tasks such as quality control and customer service, thereby driving overall productivity and customer satisfaction.
AI-powered Marketing Strategies
Dynamic Pricing Algorithms
Dynamic pricing algorithms in e-commerce involve adjusting product prices in real-time based on various factors such as demand, competitor pricing, inventory levels, and customer behavior. AI-driven dynamic pricing algorithms use machine learning models to analyze large datasets and make pricing decisions that maximize revenue and profitability. These algorithms can automatically set prices, offer personalized discounts or promotions, and respond to market fluctuations instantly. By dynamically adjusting prices, e-commerce businesses can optimize sales performance, improve competitiveness, and capitalize on pricing opportunities, ultimately enhancing overall revenue and customer satisfaction.
Behavioral Targeting and Segmentation
Behavioral targeting and segmentation in e-commerce leverage AI and data analytics to categorize customers into segments based on their behaviors, preferences, and interactions with the website or app. AI algorithms analyze browsing history, purchase patterns, demographics, and psychographics to create detailed customer profiles. Behavioral targeting enables businesses to deliver personalized marketing messages, product recommendations, and offers tailored to each segment’s interests and needs. By understanding customer behavior and preferences through segmentation, e-commerce businesses can enhance engagement, increase conversion rates, and foster long-term customer loyalty by delivering relevant and timely content that resonates with each segment.
Ad Campaign Optimization
Ad campaign optimization in e-commerce involves using AI-powered tools and algorithms to maximize the effectiveness of digital advertising efforts across various channels such as social media, search engines, and display networks. AI analyzes campaign performance metrics, audience demographics, engagement data, and conversion rates to optimize ad targeting, bidding strategies, ad creatives, and budget allocation. Through machine learning techniques like predictive analytics and A/B testing, e-commerce businesses can refine their ad campaigns in real-time, identify high-performing audience segments, and allocate resources towards campaigns that deliver the highest return on investment (ROI). Ad campaign optimization not only increases ad efficiency and cost-effectiveness but also ensures that marketing efforts align with business objectives, driving traffic, sales, and brand awareness in a competitive digital landscape.
Enhancing User Experience with AI
Chatbots and Virtual Assistants
Chatbots and virtual assistants are AI-powered technologies that enable e-commerce businesses to provide automated customer support and enhance user interactions. Chatbots, deployed on websites or messaging platforms, use natural language processing (NLP) to understand and respond to customer inquiries in real-time. They can handle routine tasks such as answering FAQs, processing orders, providing product recommendations, and even initiating customer service requests. Virtual assistants, more advanced iterations of chatbots, can engage in more complex conversations and perform tasks across multiple channels, offering personalized assistance based on user preferences and historical interactions. By leveraging chatbots and virtual assistants, e-commerce businesses can improve customer satisfaction, reduce response times, and streamline operational efficiencies, ultimately enhancing the overall shopping experience.
Voice Commerce Integration
Voice commerce integration refers to the capability of e-commerce platforms to support transactions and interactions via voice-activated devices such as smart speakers and virtual assistants. AI technologies enable voice recognition, natural language understanding, and voice search capabilities, allowing customers to browse products, add items to their carts, and complete purchases using voice commands. Voice commerce leverages machine learning algorithms to understand and process spoken language, retrieve relevant product information, and provide personalized recommendations based on user preferences. By integrating voice commerce into their platforms, e-commerce businesses can cater to the growing trend of voice search and hands-free interactions, offering convenience and accessibility to customers while driving sales and enhancing brand loyalty.
Visual Search Technology
Visual search technology utilizes AI and computer vision to enable users to search for products online using images rather than text-based queries. E-commerce platforms integrate visual search capabilities that allow customers to upload or take a photo of an item they are interested in, and the AI system identifies similar products from the inventory. AI algorithms analyze visual features such as colors, shapes, patterns, and textures to match the user’s image with relevant products. Visual search enhances the shopping experience by making product discovery more intuitive and efficient, especially for items that are difficult to describe in words. By leveraging visual search technology, e-commerce businesses can increase engagement, reduce bounce rates, and drive conversions by presenting visually similar products that meet customer preferences and style preferences accurately.
AI and Fraud Detection
Transaction Monitoring and Anomaly Detection
Transaction monitoring and anomaly detection in e-commerce involve leveraging AI-powered systems to detect suspicious or fraudulent activities in real-time. AI algorithms analyze transactional data, user behavior patterns, and historical trends to identify deviations from normal purchasing behavior or potential fraudulent transactions. These systems use machine learning techniques such as anomaly detection models, behavioral analytics, and pattern recognition to flag transactions that may indicate fraud, such as unusually large purchases, rapid successive transactions, or transactions from unfamiliar locations. By continuously monitoring transactions and detecting anomalies promptly, e-commerce businesses can mitigate risks, protect customer data, and maintain trust and security within their online payment systems.
Identity Verification Solutions
Identity verification solutions in e-commerce utilize AI technologies to authenticate and validate the identity of customers during account creation, login processes, and transactions. AI-driven identity verification systems employ biometric verification, document verification, and behavioral analysis to ensure that individuals accessing e-commerce platforms are who they claim to be. Biometric verification techniques include facial recognition, fingerprint scanning, and voice recognition, while document verification involves scanning and validating government-issued IDs or passports. Behavioral analysis examines user interaction patterns and device fingerprinting to detect suspicious activities or attempts at identity theft. By integrating robust identity verification solutions, e-commerce businesses can enhance security, prevent account takeover fraud, comply with regulatory requirements, and safeguard sensitive customer information effectively.
AI in Cybersecurity for E-commerce
AI plays a critical role in enhancing cybersecurity measures for e-commerce businesses by proactively identifying and mitigating potential threats and vulnerabilities. AI-powered cybersecurity solutions utilize machine learning algorithms to analyze vast amounts of data, including network traffic, user behaviors, and system logs, to detect and respond to cyber threats in real-time. AI algorithms can detect unusual patterns indicative of malware, phishing attacks, distributed denial-of-service (DDoS) attacks, and other cyber threats that target e-commerce platforms. Additionally, AI enhances threat intelligence gathering, automates incident response processes, and improves the accuracy of threat detection and mitigation efforts. By leveraging AI in cybersecurity, e-commerce businesses can strengthen their defenses, reduce the impact of cyber attacks, and ensure the continuous protection of sensitive customer data and transactional information.
Ethical Considerations in AI-driven E-commerce
Data Privacy and Security Concerns
Data privacy and security concerns are paramount in e-commerce, where personal and financial information is exchanged online. E-commerce businesses collect and store vast amounts of customer data, including payment details, browsing history, and personal preferences, making them attractive targets for cyber attacks and data breaches. AI technologies play a dual role in addressing these concerns by enhancing security measures and managing data privacy. AI-driven cybersecurity solutions employ advanced encryption, anomaly detection, and behavioral analysis to protect sensitive data and mitigate risks of unauthorized access or breaches. Moreover, businesses must adhere to regulatory frameworks such as GDPR (General Data Protection Regulation) and CCPA (California Consumer Privacy Act) to ensure the lawful collection, use, and retention of customer data, thus fostering trust and maintaining transparency in their data handling practices.
Bias and Fairness in AI Algorithms
Bias and fairness in AI algorithms are critical considerations in e-commerce applications, as AI systems make decisions that impact customers’ experiences and opportunities. AI algorithms can inadvertently perpetuate biases present in training data, resulting in unfair outcomes such as discriminatory pricing, biased product recommendations, or uneven access to services. To mitigate bias, e-commerce businesses must adopt strategies like dataset diversification, bias detection tools, and algorithmic auditing processes. Implementing diverse and representative training datasets and employing fairness-aware AI techniques can help mitigate bias and ensure equitable outcomes for all customers. Transparency in how AI systems operate and the factors influencing decision-making is crucial for building trust and accountability, promoting fairness in e-commerce environments.
Transparency and Accountability
Transparency and accountability are foundational principles in the ethical deployment of AI in e-commerce. E-commerce businesses using AI must be transparent about how AI systems operate, the data they collect, and how they use that data to make decisions that affect customers. Transparent communication regarding data privacy practices, AI algorithms, and the purposes for which customer data is used fosters trust and empowers customers to make informed choices. Moreover, accountability involves taking responsibility for the outcomes of AI-driven decisions, including addressing errors, biases, or unintended consequences. Implementing mechanisms for auditing AI systems, providing explanations for AI-generated decisions, and establishing clear lines of responsibility within organizations are essential steps towards ensuring accountability in e-commerce AI applications. By prioritizing transparency and accountability, e-commerce businesses can build stronger relationships with customers, enhance regulatory compliance, and uphold ethical standards in AI deployment.
Future Trends in AI and E-commerce
AI and Augmented Reality (AR)
AI and augmented reality (AR) are converging to revolutionize the e-commerce experience by enhancing interactivity and personalization. AI-powered algorithms analyze user preferences, browsing behavior, and contextual data to deliver personalized AR experiences. AR overlays digital information onto the physical world, allowing customers to visualize products in their environment before making a purchase. AI enhances AR by improving object recognition, spatial mapping, and gesture recognition capabilities, thereby creating more immersive and accurate AR simulations. E-commerce businesses leverage AI-driven AR applications for virtual try-ons, interactive product demonstrations, and customized visualizations, enhancing customer engagement and reducing return rates. The combination of AI and AR not only transforms how consumers shop online but also empowers businesses to create innovative and memorable shopping experiences that drive sales and brand loyalty in a competitive market.
Blockchain Integration with AI
Blockchain integration with AI in e-commerce represents a powerful synergy that enhances trust, security, and transparency across transactions and data management processes. Blockchain technology provides a decentralized and immutable ledger that records transactions securely, eliminating the need for intermediaries and reducing the risk of fraud or data tampering. AI complements blockchain by enhancing data analytics, fraud detection, and decision-making processes through advanced algorithms and machine learning models. AI algorithms can analyze blockchain data to identify patterns, predict market trends, and optimize supply chain operations. Moreover, blockchain enhances AI-driven solutions by providing a transparent and auditable record of data provenance and ensuring compliance with data privacy regulations. By integrating blockchain with AI, e-commerce businesses can foster trust with customers, streamline operations, and unlock new opportunities for innovation in secure and efficient online transactions.
Predictive Customer Service
Predictive customer service leverages AI and machine learning algorithms to anticipate and address customer needs proactively, enhancing satisfaction and loyalty. AI analyzes historical customer interactions, purchase behaviors, and demographic data to predict future service requirements and preferences. Predictive models can forecast customer inquiries, predict potential issues, and recommend personalized solutions in real-time. E-commerce businesses use AI-powered predictive customer service to automate responses, prioritize support tickets, and route inquiries to the most appropriate channels or personnel. By anticipating customer needs and delivering timely, relevant assistance, businesses can improve first-contact resolution rates, reduce wait times, and enhance overall service efficiency. Predictive customer service not only enhances the customer experience but also enables e-commerce businesses to optimize resource allocation, strengthen customer relationships, and differentiate themselves in a competitive market landscape dominated by personalized service and responsiveness.
Case Studies of Successful AI Implementations
Amazon: Leveraging AI for Product Recommendations
Amazon, a pioneer in e-commerce, extensively employs AI to enhance the shopping experience through personalized product recommendations. Using machine learning algorithms, Amazon analyzes vast amounts of customer data, including purchase history, browsing behavior, and demographic information, to predict and suggest products that match individual preferences. These AI-driven recommendations are prominently featured on Amazon’s website and app, offering customers tailored suggestions that increase the likelihood of purchases and improve overall user engagement. By continuously refining its recommendation engine, Amazon not only boosts sales but also cultivates customer loyalty by delivering relevant and timely suggestions that cater to diverse interests and shopping patterns.
Alibaba: AI in Supply Chain and Logistics
Alibaba, a global leader in e-commerce and technology, leverages AI to optimize supply chain and logistics operations on a massive scale. AI algorithms analyze real-time data from Alibaba’s extensive network of suppliers, warehouses, and delivery routes to forecast demand, optimize inventory levels, and improve shipping efficiency. Alibaba uses predictive analytics and machine learning to anticipate market trends, manage inventory dynamically, and reduce logistics costs while ensuring timely delivery to customers worldwide. By integrating AI into its supply chain management systems, Alibaba enhances operational agility, minimizes risks of overstocking or stockouts, and maintains high service levels that contribute to its competitive advantage in the global e-commerce landscape.
Netflix: Personalization through AI-driven Content Curation
Netflix, a leading streaming platform, relies heavily on AI to personalize content recommendations and enhance user satisfaction. Using advanced machine learning algorithms, Netflix analyzes viewing behaviors, viewing history, genre preferences, and even contextual data such as time of day to curate personalized content suggestions for each user. AI-powered recommendation systems on Netflix continuously learn and adapt based on user interactions, improving the accuracy and relevance of content suggestions over time. This personalization strategy not only increases viewer engagement and retention but also drives content discovery by introducing users to new movies and shows tailored to their individual tastes. By harnessing AI for content curation, Netflix shapes the viewing experience, promotes content diversity, and maintains its position as a leader in the competitive streaming industry.
Challenges in Implementing AI in E-commerce
Integration with Legacy Systems
Integration with legacy systems refers to the process of incorporating new technologies, such as AI and advanced analytics, into existing IT infrastructures that may be outdated or not originally designed to support modern digital innovations. Legacy systems often present challenges such as compatibility issues, data silos, and rigid architectures that can hinder seamless integration with newer technologies. However, with careful planning and implementation strategies, businesses can leverage tools like APIs, middleware, and hybrid cloud solutions to bridge the gap between legacy systems and modern technologies. AI plays a crucial role in facilitating this integration by offering solutions that can interact with and extract value from legacy data sources, automate repetitive tasks, and enhance decision-making processes. By successfully integrating AI with legacy systems, businesses can unlock operational efficiencies, improve data accessibility, and accelerate digital transformation initiatives while preserving existing investments in IT infrastructure.
Skill Gap and Workforce Training
The integration of AI and advanced technologies into business operations often highlights the need for upskilling or reskilling the workforce to effectively leverage these innovations. The rapid evolution of AI requires employees to acquire new skills in data science, machine learning, programming languages, and AI-specific technologies to harness the full potential of these tools. Addressing the skill gap through comprehensive workforce training programs is essential for maximizing ROI and ensuring successful implementation of AI initiatives. Businesses can invest in training programs, workshops, online courses, and certifications to empower employees with the knowledge and skills needed to operate AI systems, interpret data insights, and apply AI-driven solutions to real-world business challenges. By prioritizing workforce training, businesses not only enhance employee productivity and job satisfaction but also foster a culture of continuous learning and innovation that drives long-term success in the digital age.
ROI Measurement and Implementation Costs
ROI measurement and implementation costs are critical considerations for businesses adopting AI and other advanced technologies. Determining the return on investment (ROI) of AI initiatives involves evaluating both the tangible benefits, such as cost savings, revenue growth, and productivity gains, as well as the intangible benefits, such as improved customer satisfaction and competitive advantage. Businesses must establish clear metrics and KPIs aligned with their strategic objectives to assess the impact of AI implementations accurately. Additionally, measuring ROI requires consideration of implementation costs, including expenses related to software licenses, hardware infrastructure, data integration, and workforce training. While initial implementation costs can be significant, businesses can mitigate expenses through careful budgeting, phased deployment strategies, and leveraging cloud-based AI solutions that offer scalability and flexibility. By conducting thorough ROI assessments and managing implementation costs effectively, businesses can make informed decisions, justify AI investments, and achieve sustainable growth and profitability in an increasingly competitive marketplace.
Regulatory Landscape for AI in E-commerce
GDPR and Data Protection Regulations
The General Data Protection Regulation (GDPR), enacted by the European Union (EU), sets stringent standards for data protection and privacy rights of individuals within the EU and European Economic Area (EEA). GDPR imposes obligations on businesses that collect, process, or store personal data, including customer information in e-commerce contexts. Key principles of GDPR include transparency, lawful processing, purpose limitation, data minimization, accuracy, storage limitation, integrity, and confidentiality. E-commerce businesses must comply with GDPR by obtaining explicit consent for data processing, providing individuals with rights to access, rectify, and erase their personal data upon request, and implementing measures to secure personal data against breaches or unauthorized access. Non-compliance with GDPR can result in significant fines and penalties, underscoring the importance of robust data protection practices and adherence to regulatory requirements to maintain customer trust and avoid legal consequences.
Compliance with AI Ethics Guidelines
Compliance with AI ethics guidelines is essential for ensuring responsible and ethical deployment of AI technologies in e-commerce and beyond. Various organizations and institutions, including governments, industry bodies, and advocacy groups, have developed AI ethics guidelines to address concerns such as fairness, transparency, accountability, privacy, bias mitigation, and societal impact. E-commerce businesses adopting AI must adhere to these guidelines to mitigate risks associated with AI-driven decisions and ensure that AI systems operate ethically and responsibly. Implementing ethical AI principles involves conducting ethical impact assessments, designing AI systems that prioritize human values and rights, fostering transparency in AI decision-making processes, and establishing mechanisms for accountability and oversight. By embracing ethical AI practices, e-commerce businesses can build trust with customers, uphold ethical standards, and contribute positively to societal well-being while leveraging AI’s transformative potential.
Global Perspectives on AI Regulations
AI regulations vary significantly across different countries and regions, reflecting diverse cultural, legal, and technological landscapes. While some countries have enacted comprehensive AI strategies and regulations to foster innovation while addressing ethical concerns and risks, others are in the process of developing regulatory frameworks to govern AI applications. For example, countries like the United States and European Union have initiated discussions and proposals for AI regulations that encompass areas such as data privacy, algorithmic transparency, cybersecurity, and accountability. In contrast, countries like China have prioritized AI development through national strategies and regulations aimed at promoting AI innovation and deployment across various sectors, including e-commerce. Global perspectives on AI regulations highlight the need for international collaboration, harmonization of standards, and shared governance principles to address cross-border challenges, ensure regulatory compliance, and promote responsible AI adoption on a global scale. As AI continues to evolve, navigating regulatory landscapes requires businesses to stay informed about emerging regulations, adapt their AI strategies accordingly, and engage in constructive dialogue with stakeholders to shape the future of AI governance and regulation worldwide.
AI Tools and Technologies for E-commerce Businesses
Open-source AI Frameworks
Open-source AI frameworks are software platforms that provide tools, libraries, and resources for developing and deploying AI applications. These frameworks are publicly accessible and allow developers to collaborate, modify, and redistribute code freely, fostering innovation and community-driven advancements in AI. Popular open-source AI frameworks include TensorFlow, PyTorch, scikit-learn, and Apache MXNet, each offering capabilities such as machine learning algorithms, neural network architectures, and data processing tools. By leveraging open-source AI frameworks, businesses benefit from cost-effective solutions, accelerated development cycles, and access to a vibrant community of developers contributing to continuous improvements and updates. Moreover, open-source frameworks promote transparency, flexibility, and customization, enabling businesses to tailor AI solutions to their specific needs while adhering to licensing terms that support commercial use and application deployment.
Cloud-based AI Solutions
Cloud-based AI solutions leverage cloud computing infrastructure to deliver scalable, accessible, and cost-effective AI capabilities over the internet. Cloud providers offer AI services such as machine learning models, natural language processing (NLP), computer vision, and data analytics through platforms like Amazon Web Services (AWS), Microsoft Azure, and Google Cloud Platform (GCP). These platforms provide on-demand access to compute resources, storage, and AI tools without the need for businesses to invest in and manage their own infrastructure. Cloud-based AI solutions enable businesses to deploy AI applications quickly, scale operations seamlessly, and integrate AI functionalities into existing workflows. Additionally, cloud providers offer managed AI services that handle tasks like model training, deployment, and monitoring, reducing the complexity and overhead associated with AI development. By embracing cloud-based AI solutions, businesses can innovate rapidly, leverage advanced AI capabilities, and harness the scalability and reliability of cloud computing to drive business growth and competitive advantage in the digital economy.
Custom AI Applications Development
Custom AI applications development involves designing, building, and deploying AI solutions tailored to meet specific business objectives, challenges, and industry requirements. Unlike off-the-shelf AI solutions, custom applications are developed from scratch or adapted from existing frameworks to address unique use cases and organizational needs. Businesses collaborate with AI developers, data scientists, and domain experts to define requirements, gather and preprocess data, select appropriate algorithms, and integrate AI functionalities into existing IT infrastructures. Custom AI applications span various domains, including predictive analytics, recommendation systems, image recognition, natural language processing (NLP), and robotic process automation (RPA), among others. By investing in custom AI development, businesses can achieve precise problem-solving capabilities, gain competitive differentiation, and capitalize on AI-driven insights to optimize operations, enhance customer experiences, and drive innovation across diverse industries. Custom AI applications empower businesses to harness the full potential of artificial intelligence tailored to their specific requirements, delivering measurable value and sustainable growth in an increasingly AI-driven marketplace.
Evaluating AI Solutions for E-commerce
Key Metrics for AI Performance
Key metrics for evaluating AI performance encompass various dimensions that measure the effectiveness, efficiency, and impact of AI systems deployed in different applications. These metrics include accuracy, precision, recall, and F1-score for classification tasks, which assess the model’s ability to correctly classify data points. For regression tasks, metrics like mean squared error (MSE) or mean absolute error (MAE) quantify the model’s predictive accuracy. Other essential metrics include computational efficiency metrics such as training time and inference latency, which determine how quickly models can process data. Additionally, metrics like scalability, robustness, and interpretability assess broader aspects of AI performance, ensuring that models can handle large datasets, maintain performance under varying conditions, and provide explanations for their decisions. Businesses utilize these metrics to evaluate AI models, optimize performance, and make informed decisions about model deployment and improvement strategies.
Vendor Selection Criteria
Selecting the right AI vendor involves evaluating several criteria to ensure compatibility, reliability, and alignment with business objectives. Key criteria include the vendor’s expertise and experience in AI technologies, including specific domains such as machine learning, natural language processing, computer vision, or robotics. Businesses assess vendor capabilities through case studies, client testimonials, and technical assessments to validate expertise and track record. Additionally, evaluating the scalability and flexibility of AI solutions offered by vendors is crucial to accommodate future growth and evolving business needs. Other considerations include data security measures, compliance with regulatory standards such as GDPR, and integration capabilities with existing IT infrastructure. Furthermore, evaluating vendor pricing models, service-level agreements (SLAs), and ongoing support options ensures transparency and aligns with budgetary and operational requirements. By thoroughly assessing these criteria, businesses can select AI vendors that deliver value, innovation, and sustainable partnerships to drive successful AI implementations.
Case Studies of AI Implementation Failures
AI implementation failures highlight challenges and pitfalls encountered by businesses in deploying AI technologies effectively. Common reasons for AI implementation failures include inadequate data quality and quantity, which can lead to biased or inaccurate models. Poorly defined business objectives and use cases, coupled with unrealistic expectations about AI capabilities, often result in mismatched outcomes and missed opportunities. Insufficient infrastructure and resource allocation for AI initiatives, including inadequate training data, limited computational resources, and lack of skilled personnel, hinder successful deployment and scalability of AI solutions. Additionally, ethical considerations such as transparency, fairness, and accountability in AI decision-making processes can impact user trust and regulatory compliance, contributing to implementation setbacks. Case studies underscore the importance of comprehensive planning, stakeholder engagement, continuous evaluation, and adaptive strategies in mitigating risks and maximizing the potential of AI technologies to achieve desired business outcomes.
AI and Customer Retention Strategies
Predictive Churn Analysis
Predictive churn analysis utilizes AI and machine learning techniques to forecast which customers are likely to churn or discontinue their relationship with a business. By analyzing historical customer data such as purchase behavior, engagement metrics, service usage patterns, and demographics, predictive models can identify indicators or “churn signals” that precede customer attrition. These models predict the probability of churn for individual customers, enabling businesses to proactively intervene with targeted retention strategies. Predictive churn analysis empowers businesses to segment customers based on their likelihood to churn, prioritize retention efforts, and personalize interventions such as special offers, loyalty rewards, or proactive customer support. By leveraging predictive insights, businesses can reduce churn rates, enhance customer retention, and optimize revenue growth by focusing resources on retaining valuable customers who are at risk of leaving.
Loyalty Program Optimization
Loyalty program optimization involves leveraging AI and data analytics to enhance the effectiveness and impact of customer loyalty initiatives. AI-powered algorithms analyze customer behavior, transaction histories, preferences, and engagement patterns to identify opportunities for optimizing loyalty program design and rewards structures. By segmenting customers based on their value, loyalty, and purchase frequency, businesses can tailor rewards and incentives that resonate with each segment’s preferences and motivations. AI enables dynamic adjustments to loyalty program strategies in real-time based on changing customer behaviors and market conditions. Additionally, predictive analytics forecast the potential ROI of loyalty program investments, guiding decisions on resource allocation and program enhancements. Optimizing loyalty programs with AI fosters stronger customer relationships, increases repeat purchases, and drives higher customer lifetime value, ultimately enhancing brand loyalty and competitive advantage in the marketplace.
Personalized Re-engagement Campaigns
Personalized re-engagement campaigns leverage AI-driven insights to reconnect with inactive or dormant customers and rekindle their interest in products or services. AI algorithms analyze customer data, including past interactions, purchase history, preferences, and browsing behavior, to identify segments of inactive customers who may be receptive to targeted re-engagement efforts. Personalized campaigns tailor messaging, offers, and content to align with individual customer preferences and motivations, increasing the likelihood of re-engagement and conversion. AI enables predictive modeling to anticipate the most effective timing, channel, and content for re-engagement efforts, optimizing campaign performance and ROI. By delivering personalized and relevant communications, businesses can revive customer interest, drive repeat purchases, and strengthen customer loyalty over time. Personalized re-engagement campaigns powered by AI enhance customer engagement strategies, improve campaign effectiveness, and maximize the impact of marketing investments in retaining and reactivating valuable customers.
Summary
In summary, AI has profoundly transformed the landscape of e-commerce, revolutionizing how businesses operate, engage customers, and drive growth. Key takeaways include the widespread adoption of AI technologies such as machine learning, natural language processing, and computer vision to enhance personalized shopping experiences, optimize operations, and predict consumer behavior. AI-powered solutions have enabled e-commerce businesses to streamline supply chain management, improve inventory management, and automate customer service, thereby boosting efficiency and reducing costs. Moreover, AI has facilitated the development of sophisticated recommendation systems, personalized marketing campaigns, and predictive analytics that drive sales and enhance customer satisfaction. As businesses continue to integrate AI into their operations, understanding and leveraging AI’s capabilities will be crucial for staying competitive and meeting evolving consumer expectations in the digital age.
FAQs on AI in E-commerce
What is AI in e-commerce?
AI in e-commerce refers to the application of artificial intelligence technologies such as machine learning, natural language processing (NLP), and computer vision to enhance various aspects of online shopping experiences. It includes personalized recommendations, predictive analytics, virtual assistants, and automated customer service, among others.
How does AI impact online shopping?
AI enhances online shopping by providing personalized product recommendations, improving search accuracy, optimizing pricing strategies, streamlining logistics and supply chain operations, and automating customer interactions. These advancements aim to increase customer satisfaction, conversion rates, and operational efficiency for e-commerce businesses.
What are the ethical considerations of AI in e-commerce?
Ethical considerations in AI for e-commerce include issues related to data privacy, algorithmic bias, transparency in decision-making processes, and the impact of automation on jobs. It’s crucial for businesses to adopt responsible AI practices, adhere to regulatory requirements, and ensure fairness and accountability in their use of AI technologies.
How can businesses integrate AI into their e-commerce operations?
Businesses can integrate AI into e-commerce operations by leveraging AI-powered tools and platforms for customer insights, marketing automation, personalized recommendations, supply chain optimization, and customer service automation. Implementation involves data preparation, model development, testing, and continuous monitoring and improvement.