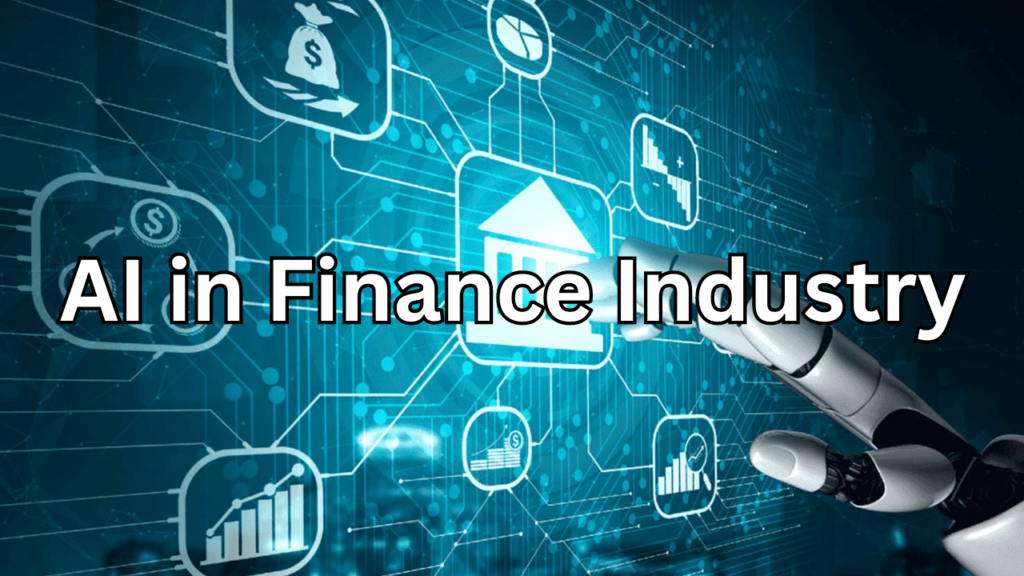
Table of Contents
Introduction
In today’s rapidly evolving financial landscape, the integration of artificial intelligence (AI) has become a game-changer. From revolutionizing customer service to enhancing decision-making processes, AI is reshaping the way the finance industry operates. Understanding the significance of AI in Finance Industry is crucial for both industry professionals and consumers alike.
Understanding the Significance of AI in Finance Industry
AI, with its ability to analyze vast amounts of data and derive actionable insights, holds immense significance in the finance sector. Traditionally, financial institutions relied heavily on manual processes and human expertise for tasks such as risk assessment, fraud detection, and investment analysis. However, the advent of AI technologies has ushered in a new era of efficiency, accuracy, and innovation.
Brief Overview of How AI is Transforming the Industry
AI is transforming the finance industry in multifaceted ways. One of its key contributions is in financial analysis, where AI-powered algorithms can crunch data, identify patterns, and predict market trends with unprecedented accuracy. This enables financial professionals to make informed investment decisions and mitigate risks more effectively.Moreover, AI is revolutionizing customer service in banking through the deployment of chatbots and virtual assistants. These AI-driven solutions provide personalized recommendations, streamline support operations, and enhance overall customer experience.
AI Applications in Financial Analysis
Utilizing AI for Market Trend Analysis
AI technologies are revolutionizing market trend analysis in the finance industry. By analyzing vast amounts of data from various sources such as social media, news articles, financial reports, and market transactions, AI algorithms can identify patterns and trends that human analysts might overlook. Through natural language processing (NLP) and sentiment analysis, AI can gauge market sentiment and investor behavior, providing valuable insights into market dynamics. Additionally, machine learning algorithms can detect correlations between different variables and predict future market movements with a high degree of accuracy. This enables financial professionals to stay ahead of market trends, identify lucrative investment opportunities, and make well-informed decisions.
Predictive Analytics for Investment Decisions
Predictive analytics powered by AI is transforming the way investment decisions are made in the finance industry. By analyzing historical data, market trends, and macroeconomic indicators, AI algorithms can forecast the performance of stocks, bonds, commodities, and other financial instruments. These predictive models can assess the potential risks and returns associated with different investment options, helping investors to optimize their portfolios and maximize returns. Moreover, AI-driven predictive analytics can identify outliers and anomalies in financial data, enabling investors to detect opportunities or mitigate potential losses proactively. Overall, predictive analytics empowers investors with actionable insights, enhancing their decision-making processes and improving investment outcomes.
Risk Management Through AI Algorithms
AI algorithms are playing a crucial role in risk management within the finance industry. By analyzing large datasets and historical patterns, AI can identify potential risks across various aspects of financial operations, including credit risk, market risk, liquidity risk, and operational risk. Machine learning algorithms can assess the probability of adverse events occurring and their potential impact on financial institutions or investment portfolios. Moreover, AI-powered risk management systems can continuously monitor market conditions and adjust risk exposure in real-time, helping financial firms to mitigate losses and comply with regulatory requirements. Additionally, AI can enhance fraud detection capabilities by identifying suspicious patterns and anomalies in transaction data, thereby minimizing financial losses due to fraudulent activities. Overall, AI-driven risk management enables financial institutions to proactively identify and address risks, safeguarding their assets and maintaining financial stability.
AI-Powered Customer Service in Banking
Chatbots and Virtual Assistants Enhancing Customer Experience
In the finance industry, chatbots and virtual assistants are revolutionizing customer experience by providing instant and personalized support round the clock. These AI-driven tools are adept at handling a wide range of customer inquiries, from account balance inquiries to transaction histories and product information. By leveraging natural language processing (NLP) and machine learning algorithms, chatbots can engage in natural and meaningful conversations with customers, offering timely assistance and guidance. Moreover, chatbots can efficiently handle routine tasks such as account inquiries and password resets, freeing up human agents to focus on more complex issues. This seamless integration of chatbots and virtual assistants enhances customer satisfaction by providing quick, convenient, and personalized support anytime, anywhere.
Personalized Recommendations and Financial Advice
AI-powered personalized recommendations and financial advice are transforming the way individuals manage their finances. By analyzing customer data, spending patterns, investment preferences, and risk tolerance levels, AI algorithms can generate tailored recommendations and insights tailored to each customer’s unique needs and goals. Whether it’s suggesting personalized investment strategies, recommending suitable financial products, or optimizing savings plans, AI-driven systems can help individuals make informed decisions that align with their financial objectives. Moreover, AI can provide ongoing monitoring and feedback, adjusting recommendations based on changing market conditions or life events. This personalized approach to financial advice not only enhances customer satisfaction but also improves financial outcomes by empowering individuals to make smarter and more strategic choices.
Streamlining Customer Support Operations with AI
AI is streamlining customer support operations in the finance industry, making it more efficient, cost-effective, and scalable. By implementing AI-powered solutions such as intelligent routing, automated responses, and predictive analytics, financial institutions can optimize their customer support processes and deliver better outcomes for their customers. For instance, AI-driven systems can prioritize incoming inquiries based on urgency and complexity, routing them to the most appropriate agent or channel for resolution. Moreover, AI-powered chatbots can handle a significant portion of customer inquiries autonomously, reducing wait times and improving response rates. Additionally, AI-driven predictive analytics can anticipate customer needs and proactively address issues before they escalate, minimizing customer frustration and enhancing overall satisfaction. By streamlining customer support operations with AI, financial institutions can improve service quality, reduce costs, and build stronger relationships with their customers.
Fraud Detection and Security Measures
AI Algorithms for Detecting Fraudulent Transactions
In the realm of finance, AI algorithms are at the forefront of detecting fraudulent transactions, providing robust security measures for both businesses and consumers. These advanced algorithms leverage machine learning techniques to analyze vast amounts of transactional data in real-time, identifying patterns and anomalies indicative of fraudulent activities. By examining various parameters such as transaction amounts, frequency, location, and user behavior, AI algorithms can flag suspicious transactions for further investigation. Moreover, AI-driven fraud detection systems can continuously learn and adapt to new fraud patterns, enhancing their accuracy and effectiveness over time. This proactive approach to fraud detection helps financial institutions minimize losses, protect customer assets, and maintain trust in the integrity of the financial system.
Enhancing Cybersecurity with AI-Driven Solutions
AI-driven solutions are playing a pivotal role in enhancing cybersecurity across the finance industry, safeguarding sensitive data and systems from cyber threats. These solutions leverage AI-powered algorithms to detect and mitigate various forms of cyber attacks, including malware, ransomware, phishing attempts, and insider threats. By analyzing network traffic, user behavior, and system logs, AI-driven cybersecurity systems can identify suspicious activities and potential vulnerabilities before they are exploited. Moreover, AI algorithms can autonomously respond to security incidents in real-time, mitigating the impact of cyber attacks and preventing data breaches. Additionally, AI-powered threat intelligence platforms can proactively identify emerging cyber threats and vulnerabilities, enabling financial institutions to stay one step ahead of cyber attackers. Overall, the integration of AI-driven solutions strengthens cybersecurity posture, ensuring the confidentiality, integrity, and availability of critical financial assets and information.
Preventing Identity Theft and Unauthorized Access
AI technologies are instrumental in preventing identity theft and unauthorized access to sensitive financial information, bolstering security measures and protecting customer identities. Through advanced biometric authentication techniques such as facial recognition, voice recognition, and fingerprint scanning, AI-driven systems can verify the identity of users with a high degree of accuracy. This helps prevent unauthorized individuals from gaining access to financial accounts or sensitive information, reducing the risk of identity theft and fraudulent activities. Moreover, AI-powered anomaly detection algorithms can monitor user behavior and flag suspicious activities that deviate from normal patterns, such as multiple login attempts from different locations or unusual transaction patterns. By proactively identifying and mitigating security threats, AI-driven solutions empower financial institutions to safeguard customer identities and uphold the trust and confidence of their clientele.
Automation of Financial Processes
Improving Efficiency through Robotic Process Automation (RPA)
Robotic Process Automation (RPA) is revolutionizing efficiency in financial operations by automating repetitive and rule-based tasks, thereby freeing up human resources to focus on more strategic activities. RPA involves deploying software robots or “bots” to mimic human actions and interact with various systems and applications to execute tasks such as data entry, invoice processing, reconciliation, and report generation. By streamlining workflows and eliminating manual intervention, RPA reduces processing times, enhances productivity, and lowers operational costs for financial institutions. Moreover, RPA can be easily scaled and integrated with existing systems, enabling organizations to adapt quickly to changing business requirements and market dynamics. Overall, RPA improves efficiency by automating mundane tasks, allowing employees to allocate their time and expertise towards value-added activities that drive business growth.
AI-Driven Automation of Routine Tasks
In the finance industry, AI-driven automation is transforming routine tasks across various functions, from customer service to risk management and compliance. AI-powered algorithms can analyze vast amounts of data and learn from patterns to perform tasks autonomously, without human intervention. For example, AI chatbots can handle routine customer inquiries, process transactions, and provide account information in real-time, enhancing customer service and satisfaction. Similarly, AI algorithms can automate routine risk assessment processes, such as credit scoring and fraud detection, by analyzing historical data and identifying anomalies or high-risk patterns. Additionally, AI-driven automation can streamline compliance processes by monitoring regulatory changes, analyzing documents, and generating reports, ensuring adherence to regulatory requirements. By automating routine tasks with AI, financial institutions can improve operational efficiency, reduce costs, and accelerate decision-making processes.
Reducing Errors and Enhancing Accuracy in Financial Operations
AI-driven automation not only improves efficiency but also reduces errors and enhances accuracy in financial operations. Manual processes are prone to human errors, such as data entry mistakes, calculation errors, and oversight errors, which can have significant implications for financial accuracy and compliance. By automating these processes with AI, financial institutions can minimize the risk of errors and ensure data integrity throughout the financial ecosystem. AI algorithms are capable of analyzing data with precision, detecting inconsistencies, and validating information against predefined rules and thresholds. Moreover, AI-powered automation systems can continuously learn and improve over time, refining processes and optimizing outcomes. By reducing errors and enhancing accuracy, AI-driven automation instills confidence in financial operations, strengthens regulatory compliance, and fosters trust among stakeholders.
AI in Wealth Management
Portfolio Optimization using Machine Learning
Machine learning is revolutionizing portfolio optimization in the finance industry by leveraging advanced algorithms to maximize returns while minimizing risk. Traditional portfolio optimization methods often rely on historical data and statistical models to construct diversified portfolios. However, machine learning algorithms can analyze vast amounts of data, including market trends, asset correlations, economic indicators, and investor sentiment, to identify optimal investment strategies. By employing techniques such as mean-variance optimization, genetic algorithms, and reinforcement learning, machine learning algorithms can dynamically adjust portfolio allocations based on changing market conditions and investor objectives. This enables investors to achieve better risk-adjusted returns and navigate volatile market environments more effectively. Moreover, machine learning-driven portfolio optimization can uncover non-linear relationships and hidden patterns in data, leading to more robust and resilient investment strategies.
Customized Investment Strategies based on Individual Preferences
In today’s dynamic investment landscape, personalized investment strategies are gaining prominence as investors seek tailored solutions that align with their unique preferences, goals, and risk tolerance levels. Machine learning algorithms play a pivotal role in crafting customized investment strategies by analyzing individual investor profiles, financial goals, investment preferences, and risk appetite. These algorithms can segment investors into distinct clusters based on demographic, behavioral, and psychographic attributes, allowing financial advisors to offer personalized recommendations and advice. By leveraging techniques such as collaborative filtering, cluster analysis, and predictive modeling, machine learning algorithms can identify investment opportunities that resonate with each investor’s specific needs and preferences. This not only enhances investor satisfaction but also improves investment outcomes by aligning portfolios with individual goals and constraints.
Automated Wealth Management Platforms
Automated wealth management platforms, also known as robo-advisors, are disrupting the wealth management industry by offering algorithm-driven investment solutions tailored to individual investors’ needs. These platforms leverage machine learning algorithms to assess investors’ risk profiles, investment objectives, and time horizons, and then recommend diversified portfolios of exchange-traded funds (ETFs) or mutual funds. By automating the investment process, robo-advisors eliminate the need for human intermediaries, making wealth management more accessible, affordable, and efficient for investors of all sizes. Moreover, automated wealth management platforms offer features such as goal-based investing, tax optimization, and rebalancing, ensuring that portfolios remain aligned with investors’ objectives over time. Additionally, machine learning algorithms continuously analyze market data and investor behavior to optimize investment strategies and mitigate risks. As a result, automated wealth management platforms provide investors with a convenient and cost-effective way to grow their wealth while maintaining personalized investment strategies.
Regulatory Compliance and AI
Ensuring Compliance with AI-Powered Regulatory Technology (RegTech)
The integration of artificial intelligence (AI) in regulatory technology (RegTech) is revolutionizing compliance processes within the finance industry. AI-powered RegTech solutions enable financial institutions to streamline regulatory compliance efforts by automating tasks such as data analysis, risk assessment, and reporting. These advanced algorithms can parse through vast amounts of regulatory data, identify relevant requirements, and ensure adherence to complex regulatory frameworks in real-time. By leveraging machine learning algorithms, AI-powered RegTech platforms can adapt to evolving regulations, detect compliance gaps, and provide actionable insights to compliance officers. This proactive approach to compliance not only enhances regulatory efficiency but also reduces the risk of non-compliance penalties and reputational damage for financial institutions. Overall, AI-powered RegTech solutions empower organizations to navigate regulatory complexities more effectively, fostering a culture of compliance and trust in the financial ecosystem.
Monitoring and Reporting Regulatory Requirements with AI
AI-driven solutions are reshaping the way financial institutions monitor and report regulatory requirements, enhancing transparency, accuracy, and efficiency in compliance processes. By leveraging natural language processing (NLP) and machine learning algorithms, AI-powered platforms can analyze regulatory documents, legislative updates, and industry guidelines to identify relevant requirements applicable to the organization. These platforms can then automatically monitor changes in regulations, assess their impact on existing policies and procedures, and generate compliance reports in a timely manner. Moreover, AI algorithms can detect anomalies and inconsistencies in compliance data, flagging potential issues for further investigation. By automating monitoring and reporting tasks, AI streamlines compliance operations, reduces manual errors, and enables compliance officers to focus on higher-value activities such as risk management and strategic planning. Ultimately, AI-driven monitoring and reporting enhance regulatory compliance effectiveness and accountability, strengthening the overall governance framework of financial institutions.
Addressing Challenges and Concerns Regarding AI in Compliance
While AI holds immense potential to revolutionize compliance processes, it also presents challenges and concerns that need to be addressed to ensure its responsible and ethical use. One of the key challenges is the interpretability of AI algorithms, as complex machine learning models may lack transparency in their decision-making processes, making it difficult to understand and explain their outputs. This raises concerns about accountability and the potential for biased or discriminatory outcomes in compliance decisions. Additionally, data privacy and security issues arise as AI systems require access to large volumes of sensitive data, raising concerns about data protection, confidentiality, and the risk of unauthorized access or misuse. Moreover, regulatory compliance in the context of AI itself is a complex and evolving field, as regulators strive to keep pace with technological advancements and address emerging risks and challenges. Financial institutions must navigate regulatory uncertainty and ensure that their AI-powered compliance solutions comply with relevant laws, regulations, and industry standards. Overall, addressing these challenges and concerns requires a collaborative effort between regulators, industry stakeholders, and technology providers to develop robust governance frameworks, ethical guidelines, and best practices for the responsible use of AI in compliance.
AI in Credit Scoring and Lending
Assessing Creditworthiness Using AI Algorithms
AI algorithms are revolutionizing the assessment of creditworthiness by providing more accurate and insightful analyses of borrower profiles and financial histories. Traditional credit scoring methods often rely on limited data points and simplistic models, leading to incomplete or biased assessments of credit risk. In contrast, AI algorithms can analyze a wide range of data sources, including transactional data, social media activity, and alternative credit data, to build comprehensive profiles of borrowers. By leveraging machine learning techniques such as predictive modeling and pattern recognition, AI algorithms can identify complex patterns and correlations in data that may not be apparent to human analysts. This enables financial institutions to make more informed decisions about lending, resulting in better outcomes for both lenders and borrowers. Moreover, AI-driven credit assessment systems can continuously learn and adapt to changing market conditions and borrower behaviors, improving their predictive accuracy over time. Overall, assessing creditworthiness using AI algorithms enhances the efficiency, accuracy, and fairness of lending processes, enabling financial institutions to expand access to credit while minimizing credit risk.
Streamlining Loan Approval Processes with Machine Learning
Machine learning is streamlining loan approval processes within the finance industry by automating and optimizing various stages of the lending lifecycle. Traditional loan approval processes often involve manual data entry, paper-based documentation, and lengthy decision-making timelines, resulting in inefficiencies and delays for both lenders and borrowers. Machine learning algorithms can automate data collection, verification, and analysis processes, reducing the time and effort required to process loan applications. By analyzing historical loan data, borrower profiles, and credit risk indicators, machine learning algorithms can predict the likelihood of loan default and assess the creditworthiness of applicants more accurately and efficiently. This enables financial institutions to make faster, more consistent, and data-driven lending decisions, improving the overall customer experience and reducing operational costs. Moreover, machine learning-driven loan approval systems can identify opportunities for process optimization and risk mitigation, enabling lenders to enhance their competitiveness and profitability in the lending market. Overall, streamlining loan approval processes with machine learning improves efficiency, transparency, and accessibility in lending, fostering financial inclusion and economic growth.
Reducing Bias in Credit Scoring through AI
AI is playing a crucial role in reducing bias in credit scoring by leveraging advanced algorithms and data-driven approaches to assess credit risk more objectively and fairly. Traditional credit scoring models may inadvertently perpetuate biases based on factors such as race, gender, or socioeconomic status, leading to unequal access to credit and financial services. AI algorithms can mitigate these biases by focusing on relevant and predictive factors of creditworthiness, rather than relying on potentially discriminatory variables. By analyzing a diverse set of data points and using techniques such as feature engineering and fairness-aware machine learning, AI algorithms can identify and mitigate biases in credit scoring models. For example, AI algorithms can adjust for historical disparities in credit access or incorporate alternative data sources that reflect an individual’s financial behavior more accurately. Moreover, transparency and explainability features in AI-driven credit scoring systems enable lenders to understand how decisions are made and identify and address any potential biases or disparities. Overall, reducing bias in credit scoring through AI promotes fairness, equality, and inclusion in the lending process, enabling financial institutions to serve a broader and more diverse customer base while minimizing credit risk.
AI Ethics and Governance in Finance
Ensuring Transparency and Accountability in AI Applications
Transparency and accountability are paramount in ensuring the responsible and ethical use of AI applications in the finance industry. As AI algorithms become increasingly complex and pervasive, it is essential for financial institutions to provide transparency into how AI-driven decisions are made and ensure accountability for their outcomes. Transparency involves disclosing information about the data sources, algorithms, and decision-making processes used in AI applications, enabling stakeholders to understand and assess their reliability, fairness, and potential biases. Financial institutions must also establish mechanisms for accountability, including clear roles and responsibilities for AI developers, users, and decision-makers, as well as processes for monitoring, evaluating, and addressing the impact of AI-driven decisions on individuals, organizations, and society. By fostering transparency and accountability in AI applications, financial institutions can build trust, mitigate risks, and promote responsible innovation in the finance industry.
Ethical Considerations in AI-Driven Decision-Making
Ethical considerations play a critical role in guiding AI-driven decision-making processes in the finance industry, ensuring that decisions are made in a manner that is fair, unbiased, and respectful of individuals’ rights and interests. Financial institutions must consider ethical principles such as fairness, transparency, accountability, privacy, and consent when developing and deploying AI applications. For example, AI algorithms should be designed to avoid discrimination and promote equal opportunities for all individuals, regardless of their race, gender, or socioeconomic status. Additionally, financial institutions must be transparent about how AI-driven decisions are made and provide individuals with meaningful control over their personal data and preferences. Moreover, financial institutions must establish mechanisms for accountability and recourse in cases where AI-driven decisions result in adverse outcomes or harm. By embedding ethical considerations into AI-driven decision-making processes, financial institutions can uphold their moral and social responsibilities, build trust with stakeholders, and contribute to a more inclusive and equitable finance industry.
Establishing Regulatory Frameworks for AI in Finance Industry
Establishing regulatory frameworks for AI in Finance Industry is essential for promoting innovation, protecting consumers, and ensuring the responsible and ethical use of AI technologies. Regulatory frameworks provide clear guidelines and standards for the development, deployment, and use of AI applications in the finance industry, addressing key considerations such as data privacy, security, transparency, accountability, and fairness. Regulatory authorities must collaborate with industry stakeholders, technology experts, and policymakers to develop robust and flexible regulatory frameworks that accommodate the rapid pace of technological advancements and address emerging risks and challenges. Moreover, regulatory frameworks should strike a balance between promoting innovation and mitigating risks, fostering a regulatory environment that encourages responsible experimentation and learning while safeguarding against potential harms and abuses. By establishing regulatory frameworks for AI in finance, regulatory authorities can promote trust, stability, and confidence in the finance industry, enabling financial institutions to harness the full potential of AI technologies for the benefit of society while minimizing risks and ensuring compliance with legal and ethical standards.
Case Studies: Successful Implementation of AI in Finance Industry
Highlighting Real-World Examples of AI Adoption in Finance
Real-world examples of AI adoption in finance demonstrate the transformative power of AI technologies across various areas of the industry. For instance, JPMorgan Chase utilizes AI-powered algorithms to analyze legal documents and contracts, significantly reducing the time and resources required for manual review processes. Goldman Sachs leverages machine learning algorithms to automate trading strategies and optimize investment decisions, enabling traders to respond quickly to market trends and generate higher returns. Additionally, Capital One employs AI-driven chatbots to provide personalized customer support and assistance, enhancing the overall customer experience and reducing call center volumes. These examples illustrate how financial institutions are leveraging AI technologies to drive innovation, improve operational efficiency, and deliver value to customers and shareholders.
Benefits and Outcomes of Integrating AI Solutions
The integration of AI solutions in finance offers a wide range of benefits and outcomes for financial institutions, customers, and stakeholders alike. Firstly, AI-driven automation streamlines manual processes, reduces operational costs, and increases efficiency across various functions such as risk management, compliance, and customer service. Secondly, AI-powered predictive analytics enables financial institutions to make data-driven decisions, optimize resource allocation, and identify new business opportunities. Thirdly, AI-driven personalization enhances the customer experience by providing tailored recommendations, products, and services that meet individual needs and preferences. Additionally, AI solutions improve risk management capabilities by detecting fraudulent activities, identifying anomalies, and mitigating potential risks in real-time. Moreover, AI technologies enable financial institutions to stay competitive in a rapidly evolving industry landscape by fostering innovation, agility, and adaptability. Overall, integrating AI solutions in finance delivers tangible benefits such as cost savings, revenue growth, risk mitigation, and customer satisfaction, positioning financial institutions for long-term success and sustainability.
Lessons Learned from Leading Financial Institutions
Leading financial institutions have garnered valuable insights and lessons from their experiences with AI adoption, providing guidance for others embarking on similar journeys. Firstly, successful AI adoption requires strong leadership commitment, vision, and alignment with business objectives, ensuring that AI initiatives are strategically aligned with organizational priorities and goals. Secondly, collaboration and partnership with technology vendors, industry experts, and regulatory authorities are essential for overcoming challenges and driving innovation in AI adoption. Thirdly, investing in talent development and capability building is critical for building internal expertise and capacity to develop, deploy, and manage AI solutions effectively. Additionally, fostering a culture of experimentation, learning, and continuous improvement enables financial institutions to iterate, refine, and scale AI initiatives over time. Moreover, transparency, ethics, and trust are foundational principles for responsible AI adoption, fostering trust with stakeholders and promoting ethical practices in AI-driven decision-making. By embracing these lessons learned, financial institutions can navigate the complexities of AI adoption successfully and unlock the full potential of AI technologies to drive value and innovation in the finance industry.
Future Trends and Predictions
Emerging Technologies Shaping the Future of Finance
Several emerging technologies are poised to reshape the future of finance, driving innovation, efficiency, and customer-centricity across the industry. One such technology is blockchain, which offers decentralized and immutable ledgers for secure and transparent transactions, enabling faster and more cost-effective cross-border payments, smart contracts, and tokenization of assets. Another emerging technology is quantum computing, which promises to revolutionize data processing and analytics, enabling financial institutions to solve complex problems and optimize portfolios with unprecedented speed and accuracy. Additionally, Internet of Things (IoT) devices and sensors provide real-time data insights for risk management, fraud detection, and customer engagement, while augmented reality (AR) and virtual reality (VR) enhance the customer experience through immersive banking and investment experiences. These emerging technologies hold the potential to drive significant transformation and disruption in the finance industry, unlocking new business models, revenue streams, and opportunities for growth.
Predictions for the Evolution of AI in the Finance Industry
The evolution of AI in the finance industry is expected to continue at a rapid pace, with several key trends and developments shaping its trajectory. Firstly, AI-powered personalization will become more prevalent, as financial institutions leverage customer data and machine learning algorithms to deliver hyper-personalized products, services, and recommendations tailored to individual preferences and needs. Secondly, explainable AI will gain importance, as regulators and stakeholders seek transparency and accountability in AI-driven decision-making processes, ensuring that AI algorithms are fair, unbiased, and compliant with regulatory requirements. Thirdly, AI-driven automation will expand beyond routine tasks to more complex decision-making processes such as underwriting, risk assessment, and fraud detection, enabling financial institutions to streamline operations, reduce costs, and mitigate risks more effectively. Additionally, AI-driven predictive analytics will enable financial institutions to anticipate market trends, identify emerging risks, and capitalize on opportunities with greater precision and foresight. Overall, the evolution of AI in the finance industry promises to enhance efficiency, innovation, and customer experiences, driving value and competitive advantage for financial institutions in a rapidly changing landscape.
Opportunities for Innovation and Growth
The finance industry is ripe with opportunities for innovation and growth, fueled by emerging technologies, changing customer expectations, and evolving regulatory landscapes. One opportunity lies in digital transformation, as financial institutions embrace technology-driven strategies to modernize legacy systems, digitize processes, and enhance customer experiences across channels. Another opportunity lies in data-driven insights, as financial institutions leverage big data analytics, AI, and machine learning to extract actionable insights from vast amounts of data, enabling more informed decision-making, risk management, and product development. Additionally, partnerships and collaborations with fintech startups, technology vendors, and ecosystem players offer opportunities for financial institutions to access new markets, diversify revenue streams, and experiment with innovative business models. Moreover, regulatory sandboxes and innovation hubs provide platforms for financial institutions to test and scale innovative solutions in a controlled environment, fostering experimentation, learning, and collaboration. Overall, the opportunities for innovation and growth in the finance industry are vast and diverse, enabling financial institutions to stay ahead of the curve, drive value for stakeholders, and shape the future of finance in a dynamic and competitive landscape.
Frequently Asked Questions (FAQs)
What is artificial intelligence (AI) in the context of finance?
Artificial intelligence (AI) refers to the simulation of human intelligence processes by machines, particularly computer systems. In the context of finance, AI encompasses various technologies such as machine learning, natural language processing (NLP), and robotic process automation (RPA) to analyze data, make predictions, and automate tasks. AI enables financial institutions to leverage data-driven insights, improve decision-making processes, and enhance operational efficiency across various functions such as customer service, risk management, and investment management.
How is AI transforming financial analysis and investment decisions?
AI is transforming financial analysis and investment decisions by enabling more accurate, timely, and data-driven insights. AI algorithms can analyze vast amounts of financial data from diverse sources such as market trends, economic indicators, and company reports to identify patterns, trends, and anomalies that may impact investment opportunities. By leveraging machine learning techniques, AI can generate predictive models that forecast market movements, assess risk factors, and optimize investment strategies. This enables investors to make more informed decisions, mitigate risks, and maximize returns in a dynamic and competitive market environment.
What are the benefits of using AI-powered customer service in banking?
AI-powered customer service offers several benefits for banking institutions and their customers. Firstly, AI-driven chatbots and virtual assistants provide immediate and personalized assistance to customers, enhancing the overall customer experience and satisfaction. Secondly, AI-powered customer service solutions can handle a wide range of inquiries and tasks, such as account inquiries, transaction requests, and product recommendations, reducing the burden on human agents and increasing operational efficiency. Thirdly, AI-driven customer service solutions can operate 24/7, enabling banks to provide round-the-clock support to customers across different time zones and channels. Moreover, AI-powered customer service solutions can analyze customer interactions and feedback to identify areas for improvement and optimize service delivery over time.
How does AI contribute to fraud detection and security in finance?
AI contributes to fraud detection and security in finance by analyzing patterns, anomalies, and deviations in transactional data to identify suspicious activities and potential threats. AI algorithms can detect unusual spending patterns, unauthorized access attempts, and fraudulent transactions in real-time, enabling financial institutions to respond quickly and mitigate risks. Additionally, AI-powered fraud detection systems can continuously learn and adapt to new fraud tactics and techniques, improving their accuracy and effectiveness over time. By leveraging AI technologies, financial institutions can enhance their fraud detection capabilities, protect customer assets, and maintain trust in the integrity of the financial system.
What financial processes can be automated using AI?
AI can automate various financial processes across different functions and departments within financial institutions. Some examples include:
- Automating data entry and reconciliation tasks in accounting and finance departments.
- Streamlining loan approval processes through AI-driven credit scoring and risk assessment models.
- Optimizing investment strategies and portfolio management through AI-driven predictive analytics.
- Enhancing compliance monitoring and reporting with AI-powered regulatory technology (RegTech).
- Improving customer service and engagement through AI-driven chatbots and virtual assistants. Overall, AI automation enables financial institutions to streamline operations, reduce costs, and enhance productivity while delivering better outcomes for customers and stakeholders.