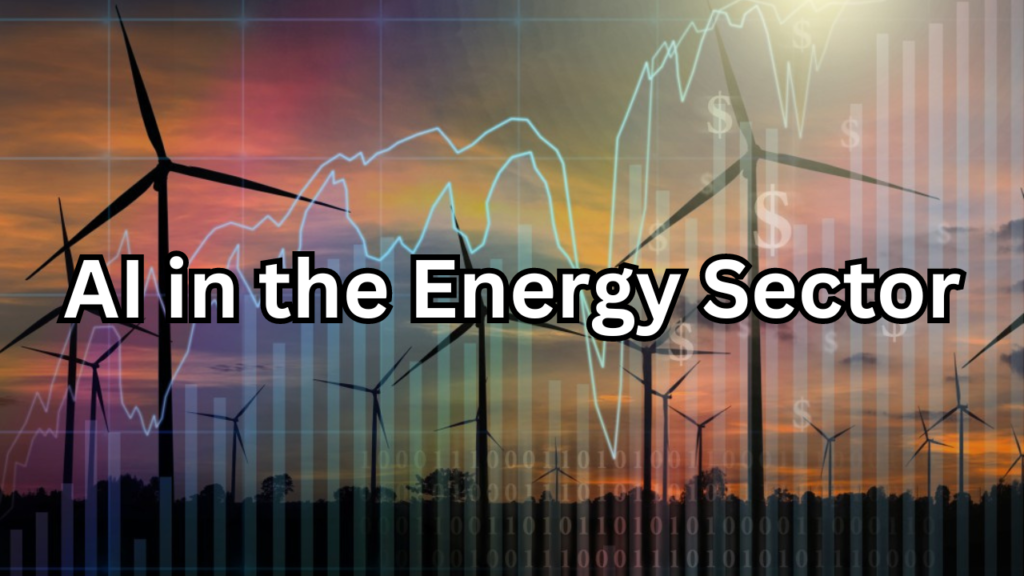
Table of Contents
Introduction
The energy sector is undergoing a transformative shift, driven by the integration of advanced technologies, among which Artificial Intelligence (AI) stands out prominently. As global energy demands rise and environmental concerns intensify, the need for efficient, sustainable, and innovative solutions has never been more critical. AI, with its vast capabilities in data analysis, predictive modeling, and automation, presents a powerful tool to revolutionize how energy is produced, distributed, stored, and consumed. This article delves into the profound impact of AI on the energy sector, exploring its applications, benefits, challenges, and future prospects.
Overview of AI and Its Relevance to the Energy Sector
Artificial Intelligence, at its core, refers to the simulation of human intelligence in machines that are programmed to think, learn, and make decisions. In the energy sector, AI’s relevance is multi-faceted. It enhances the efficiency of renewable energy sources by predicting weather patterns and optimizing energy production. AI algorithms can manage and distribute energy more effectively through smart grids, ensuring a stable and reliable power supply. In consumption, AI-driven systems help reduce energy waste and lower costs by optimizing usage patterns in homes and industries. Furthermore, AI’s ability to process vast amounts of data in real-time allows for predictive maintenance, reducing downtime and extending the lifespan of critical infrastructure. This transformative potential underscores AI’s critical role in advancing the energy sector towards a more sustainable and efficient future.
Historical Context: Evolution of AI in Energy
The evolution of AI in the energy sector has been a journey marked by significant milestones and technological advancements. In the early stages, AI applications were limited to basic automation and control systems. However, with the advent of machine learning and big data analytics in the late 20th and early 21st centuries, the scope of AI expanded dramatically. Early implementations in the energy sector focused on optimizing grid management and forecasting energy demand. Over time, as computational power and data availability increased, AI’s role grew to encompass more complex tasks, such as real-time energy trading, predictive maintenance of equipment, and integration of renewable energy sources into the grid. The continuous development of AI technologies, coupled with increasing environmental and economic pressures, has driven the energy sector to adopt AI-driven solutions more widely, heralding a new era of innovation and efficiency.
Understanding AI in Energy
Defining AI in the Context of Energy
Artificial Intelligence (AI) in the context of energy refers to the application of advanced computational techniques to solve complex problems related to the production, distribution, storage, and consumption of energy. AI encompasses a range of technologies, including machine learning, deep learning, neural networks, and natural language processing, which are used to analyze large datasets, predict outcomes, and automate decision-making processes. In the energy sector, AI enables the optimization of energy resources, enhances grid reliability, improves the efficiency of energy consumption, and facilitates the integration of renewable energy sources. By leveraging AI, energy companies can make data-driven decisions, forecast energy demand with greater accuracy, and implement more efficient and sustainable practices.
Types of AI Technologies Used in Energy
The energy sector employs various AI technologies to enhance its operations and address its unique challenges. Machine learning, a subset of AI, involves training algorithms on large datasets to recognize patterns and make predictions. This technology is widely used for forecasting energy demand, optimizing the performance of renewable energy systems, and detecting anomalies in grid operations. Deep learning, another subset, utilizes neural networks with multiple layers to process complex data, enabling more precise predictions and advanced decision-making capabilities. Neural networks, which mimic the human brain’s structure and function, are employed in applications such as image recognition for monitoring infrastructure and assessing environmental impact. Additionally, natural language processing (NLP) allows AI systems to interpret and respond to human language, facilitating better customer service and more efficient communication within the industry. These AI technologies collectively contribute to a smarter, more resilient, and more efficient energy sector.
AI vs Traditional Energy Management Systems
AI-driven energy management systems differ significantly from traditional energy management systems in their approach and capabilities. Traditional systems rely on predefined rules and historical data to manage energy production and distribution, often resulting in reactive measures rather than proactive solutions. In contrast, AI systems utilize real-time data and advanced algorithms to predict and respond to changes dynamically. AI can optimize energy use by adjusting supply based on demand forecasts, identify potential issues before they become critical, and provide insights for continuous improvement. Furthermore, AI systems can handle vast amounts of data from various sources, offering a more comprehensive and integrated approach to energy management. This leads to increased efficiency, reduced operational costs, and enhanced sustainability compared to traditional methods. As a result, AI is rapidly becoming an indispensable tool for modern energy management, enabling a more agile and adaptive energy infrastructure.
AI in Energy Production
Optimizing Renewable Energy Sources
Optimizing renewable energy sources is a critical area where Artificial Intelligence (AI) is making a substantial impact. AI algorithms can predict weather patterns with high accuracy, which is crucial for renewable energy sources like solar and wind that are dependent on environmental conditions. By analyzing vast datasets from satellites, weather stations, and historical climate data, AI systems can forecast solar radiation levels and wind speeds, enabling better planning and utilization of these resources. Additionally, AI can optimize the operation of renewable energy systems by adjusting parameters in real-time to maximize efficiency. For instance, in solar farms, AI can control the angle of solar panels to capture the maximum amount of sunlight throughout the day. In wind farms, AI can adjust the orientation of turbines to align with changing wind directions. These optimizations not only enhance the energy output but also prolong the lifespan of the equipment and reduce maintenance costs. Furthermore, AI-driven energy management systems can seamlessly integrate renewable energy into the grid, balancing supply and demand to ensure a stable and reliable power supply.
Enhancing Fossil Fuel Extraction and Use
While the transition to renewable energy is accelerating, fossil fuels still play a significant role in the global energy landscape. AI is being used to enhance the efficiency and sustainability of fossil fuel extraction and use. In the extraction phase, AI technologies such as machine learning and neural networks analyze geological data to identify the most promising locations for drilling, minimizing the environmental impact and maximizing yield. AI can also predict equipment failures and optimize maintenance schedules, reducing downtime and operational costs. During the refining and processing stages, AI algorithms monitor and control various parameters to improve efficiency, reduce waste, and lower emissions. In the consumption phase, AI helps optimize the combustion process in power plants and industrial applications, ensuring more complete and efficient fuel use. Additionally, AI-driven systems can manage and reduce emissions by monitoring pollutants in real-time and adjusting processes to minimize their release. By leveraging AI, the fossil fuel industry can operate more efficiently, reduce its environmental footprint, and transition more smoothly towards a future dominated by renewable energy sources.
AI in Energy Distribution
Smart Grids and AI
Smart grids represent a significant advancement in energy distribution infrastructure, empowered by Artificial Intelligence (AI) technologies. AI enhances smart grids by enabling real-time monitoring, analysis, and control of energy flow. Through sensors and IoT devices, AI systems gather data on electricity usage patterns, grid conditions, and environmental factors. Machine learning algorithms process this data to optimize energy distribution, predict demand fluctuations, and detect anomalies or potential failures in the grid. AI also facilitates grid resilience by automatically rerouting power during outages or peak demand periods, thereby ensuring uninterrupted supply and minimizing downtime. Moreover, AI-driven smart grids support the integration of renewable energy sources by managing their variability and optimizing their contribution to the overall grid stability. Overall, AI transforms traditional grids into intelligent, adaptive networks capable of meeting the evolving energy demands of modern societies efficiently and sustainably.
AI in Load Forecasting
AI plays a pivotal role in load forecasting, a critical aspect of energy management that involves predicting future electricity demand. Traditional methods of load forecasting rely on historical data and statistical models, which may not capture the complexity and variability of modern energy consumption patterns. AI, particularly machine learning algorithms, excels in this domain by analyzing vast datasets that include weather patterns, economic indicators, and demographic trends. By identifying correlations and patterns in data, AI models can generate accurate predictions of electricity demand at various time scales—from hourly to seasonal forecasts. These predictions enable energy providers to optimize generation schedules, allocate resources effectively, and avoid under- or over-supply situations. AI-driven load forecasting also supports dynamic pricing strategies and demand response programs, empowering consumers to make informed choices about their energy usage and contributing to overall grid stability and efficiency.
Dynamic Energy Pricing and AI
Dynamic energy pricing, facilitated by AI, revolutionizes how consumers and businesses interact with the electricity market by adjusting prices based on real-time supply and demand conditions. AI algorithms analyze data from smart meters, weather forecasts, market trends, and consumer behavior to determine optimal pricing structures dynamically. This approach incentivizes consumers to shift their electricity usage to off-peak hours when prices are lower, reducing overall demand during peak periods and alleviating strain on the grid. Moreover, AI can personalize pricing strategies based on individual consumption patterns and preferences, enhancing customer engagement and satisfaction. For energy providers, dynamic pricing supported by AI improves revenue management, minimizes operational costs, and promotes efficient resource allocation. Overall, dynamic energy pricing with AI fosters a more responsive and resilient energy market, benefiting both consumers and utilities alike in terms of cost savings, sustainability, and reliability.
AI in Energy Storage
Optimizing Battery Storage Systems
Battery storage systems play a crucial role in modern energy infrastructure, enabling the efficient integration of renewable energy sources and enhancing grid stability. Artificial Intelligence (AI) is instrumental in optimizing battery storage systems to improve performance, longevity, and reliability. AI algorithms analyze real-time data from sensors embedded in batteries, such as temperature, voltage, and charge/discharge cycles. By processing this data, AI models can predict battery degradation, optimize charging and discharging cycles to extend lifespan, and enhance overall efficiency. For instance, AI can dynamically adjust charging rates based on energy demand forecasts and grid conditions, ensuring that batteries are ready to supply electricity when needed most effectively. Furthermore, AI-driven predictive analytics enable early detection of potential faults or malfunctions, allowing for proactive maintenance and minimizing downtime. By optimizing battery storage systems with AI, energy providers can maximize the value of their investments in renewable energy integration, improve energy resiliency, and contribute to a more sustainable energy future.
Predictive Maintenance for Energy Storage
Predictive maintenance is revolutionizing how energy storage systems are managed, leveraging Artificial Intelligence (AI) to predict and prevent equipment failures before they occur. Traditional maintenance approaches rely on scheduled inspections or reactive responses to breakdowns, which can be costly and disruptive. AI-based predictive maintenance, on the other hand, uses machine learning algorithms to analyze historical data, sensor readings, and operational parameters in real-time. By identifying patterns and anomalies indicative of potential failures, AI models can forecast the remaining useful life of energy storage components and recommend timely maintenance actions. This proactive approach not only reduces maintenance costs and extends equipment lifespan but also enhances system reliability and operational efficiency. For example, AI can detect abnormal temperature trends or voltage fluctuations in batteries, signaling the need for inspection or replacement before performance is compromised. By integrating predictive maintenance powered by AI, energy providers can optimize resource allocation, improve safety standards, and ensure continuous availability of energy storage systems to support grid stability and resilience.
AI in Energy Consumption
Smart Homes and AI Integration
AI integration in smart homes is revolutionizing how individuals interact with and manage their energy consumption. By leveraging Artificial Intelligence (AI), smart home devices such as thermostats, lighting systems, and appliances can learn from user behaviors and preferences to optimize energy usage. AI algorithms analyze data from sensors, smart meters, and weather forecasts to make real-time adjustments in energy consumption patterns. For instance, AI can automatically adjust thermostat settings based on occupancy and external temperatures to minimize heating or cooling energy usage. Smart lighting systems equipped with AI can dim or turn off lights in unoccupied rooms, reducing unnecessary energy consumption. Moreover, AI enables predictive maintenance of household appliances, detecting anomalies early to prevent energy inefficiencies and breakdowns. Overall, AI integration in smart homes enhances energy efficiency, reduces utility bills, and promotes sustainable living by empowering homeowners to make informed decisions about their energy usage.
AI in Industrial Energy Management
In industrial settings, AI-driven energy management systems are transforming how energy resources are monitored, optimized, and utilized. AI plays a pivotal role in analyzing vast amounts of data from manufacturing processes, equipment performance, and energy consumption patterns to identify opportunities for efficiency improvements. Machine learning algorithms can detect patterns of energy waste or inefficiencies, optimizing production schedules and equipment operation to minimize energy usage while maintaining productivity. AI also facilitates predictive maintenance of industrial machinery by monitoring operational parameters and predicting potential failures before they occur, thereby reducing downtime and maintenance costs. Furthermore, AI-driven energy management systems enable dynamic optimization of energy-intensive processes based on real-time energy prices and grid conditions. By integrating AI into industrial energy management, companies can achieve significant cost savings, enhance operational reliability, and reduce their environmental footprint through more sustainable energy practices.
Reducing Energy Waste with AI
AI technologies are instrumental in reducing energy waste across various sectors by identifying inefficiencies and optimizing energy usage. In buildings and facilities, AI-powered systems analyze occupancy patterns, climate data, and building characteristics to adjust heating, ventilation, and air conditioning (HVAC) systems for maximum efficiency. Smart sensors and meters equipped with AI algorithms monitor energy consumption in real-time, detecting anomalies and inefficiencies that could lead to energy waste. AI can also optimize lighting systems by adjusting brightness levels based on natural light availability and occupancy, thereby reducing unnecessary energy usage. In manufacturing and production environments, AI-driven predictive analytics optimize energy-intensive processes, minimizing idle times and optimizing production schedules to align with energy demand and cost fluctuations. Moreover, AI enables utilities to implement demand response programs, incentivizing consumers to shift their energy usage to off-peak hours through dynamic pricing strategies. By leveraging AI to reduce energy waste, businesses and consumers alike can lower energy bills, enhance energy efficiency, and contribute to sustainability goals by conserving valuable resources.
AI for Energy Efficiency
AI-Driven Energy Audits
AI-driven energy audits revolutionize the process of assessing and improving energy efficiency in buildings and industrial facilities. Traditional energy audits involve manual inspections and data collection, which can be time-consuming and subjective. AI transforms this process by analyzing vast amounts of data from building systems, including HVAC (Heating, Ventilation, and Air Conditioning), lighting, insulation, and appliances. Machine learning algorithms identify patterns of energy usage, detect inefficiencies, and recommend tailored energy-saving measures. For example, AI can analyze historical energy consumption data to pinpoint areas where energy is being wasted, such as inefficient equipment or poorly insulated areas. AI-driven energy audits provide actionable insights that enable building owners and facility managers to prioritize energy-saving upgrades, optimize building performance, and reduce operational costs. By leveraging AI for energy audits, organizations can achieve significant energy savings, improve sustainability efforts, and enhance the comfort and productivity of building occupants.
AI in Building Management Systems
AI is transforming building management systems by optimizing operations, enhancing efficiency, and improving occupant comfort. AI-powered building management systems integrate with sensors, IoT devices, and smart meters to monitor and control various building functions in real-time. Machine learning algorithms analyze data streams from these devices to predict patterns of occupancy, temperature fluctuations, and energy demand. This predictive capability allows AI to automatically adjust HVAC settings, lighting levels, and other building parameters to optimize energy usage while maintaining indoor comfort conditions. AI also facilitates proactive maintenance by detecting equipment faults or performance anomalies early, preventing potential breakdowns and reducing maintenance costs. Additionally, AI-driven building management systems enable remote monitoring and control, providing facility managers with actionable insights and real-time alerts to address issues promptly. By enhancing operational efficiency and reducing energy consumption, AI in building management systems contributes to sustainable building practices and operational cost savings for owners and tenants alike.
Optimizing HVAC Systems with AI
AI plays a crucial role in optimizing HVAC (Heating, Ventilation, and Air Conditioning) systems to enhance energy efficiency, reduce operational costs, and improve indoor air quality. AI algorithms analyze data from sensors, weather forecasts, occupancy patterns, and building characteristics to dynamically adjust HVAC operations in real-time. For example, AI can optimize airflow and temperature settings based on occupancy levels and external weather conditions, ensuring that heating and cooling systems operate at peak efficiency. AI-driven predictive analytics anticipate HVAC maintenance needs by monitoring system performance metrics, detecting anomalies, and scheduling proactive maintenance to prevent equipment failures and minimize downtime. Furthermore, AI optimizes energy consumption by identifying opportunities for energy savings, such as adjusting setpoints during non-peak hours or implementing demand response strategies. By leveraging AI to optimize HVAC systems, building owners and operators can achieve significant energy savings, reduce carbon emissions, and create healthier indoor environments for occupants through enhanced comfort and air quality control.
AI in Energy Trading
AI for Predictive Market Analysis
AI has revolutionized predictive market analysis in energy markets by leveraging advanced algorithms to analyze vast amounts of data and predict future market trends with high accuracy. In energy markets, AI algorithms analyze historical market data, supply and demand dynamics, geopolitical factors, weather patterns, and regulatory changes to forecast price movements and market behavior. Machine learning models can identify patterns, correlations, and anomalies that human analysts may overlook, providing valuable insights for traders and investors. AI-powered predictive market analysis enables proactive decision-making, such as anticipating price fluctuations and optimizing trading strategies to capitalize on market opportunities. By enhancing forecasting accuracy and reducing uncertainty, AI contributes to more informed and profitable trading decisions in energy markets, ultimately improving market efficiency and liquidity.
Algorithmic Trading in Energy Markets
Algorithmic trading, facilitated by AI, has transformed energy markets by automating the execution of trading strategies based on predefined rules and algorithms. AI-driven trading systems analyze real-time market data, including price movements, trading volumes, and market liquidity, to execute trades at optimal times and prices. Machine learning algorithms can adapt and refine trading strategies based on market conditions, identifying arbitrage opportunities and responding to market signals with speed and precision beyond human capabilities. Algorithmic trading in energy markets enhances liquidity, reduces transaction costs, and improves market efficiency by increasing trade execution speed and reducing market impact. However, it also introduces challenges related to algorithmic stability, regulatory compliance, and market fairness. Overall, AI-powered algorithmic trading enhances market transparency and liquidity in energy markets while presenting opportunities for enhanced risk management and profitability.
Risk Management Using AI
AI-powered risk management is transforming how energy companies and traders assess, mitigate, and manage risks in volatile and complex markets. AI algorithms analyze diverse data sources, including market data, operational metrics, geopolitical events, and weather forecasts, to identify potential risks and predict their impact on business operations and financial performance. Machine learning models can quantify and prioritize risks, such as price volatility, supply chain disruptions, regulatory changes, and geopolitical instability, enabling proactive risk mitigation strategies. AI-driven risk management systems also facilitate scenario analysis and stress testing, simulating potential outcomes under different market conditions to evaluate resilience and preparedness. By providing real-time insights and predictive analytics, AI enhances decision-making processes, strengthens risk mitigation strategies, and improves overall risk-adjusted returns for energy companies and traders. Additionally, AI-driven risk management promotes regulatory compliance, operational efficiency, and financial stability in the dynamic and competitive energy markets.
AI and Environmental Impact
Reducing Carbon Footprint with AI
AI technologies are playing a pivotal role in reducing the carbon footprint of industries and energy production processes. By harnessing the power of Artificial Intelligence (AI), companies can optimize energy use, improve operational efficiency, and adopt more sustainable practices. AI-driven systems analyze large datasets to identify opportunities for energy conservation and emission reductions across various sectors. For example, AI algorithms can optimize the operation of renewable energy sources such as solar and wind farms by predicting weather patterns and adjusting energy production accordingly. In manufacturing and industrial processes, AI monitors and optimizes energy-intensive operations to minimize waste and emissions. Furthermore, AI facilitates the development of smart grids and energy management systems that integrate renewable energy sources seamlessly, reducing reliance on fossil fuels and enhancing grid stability. By leveraging AI for energy efficiency and sustainability initiatives, businesses can achieve significant reductions in their carbon footprint while contributing to global efforts to combat climate change.
AI for Monitoring Environmental Impact of Energy Production
AI is revolutionizing the monitoring and management of environmental impact associated with energy production activities. Traditional methods of environmental monitoring often rely on manual data collection and analysis, which can be labor-intensive and limited in scope. AI technologies, on the other hand, enable real-time monitoring and analysis of environmental parameters such as air and water quality, habitat disturbance, and biodiversity impacts. AI algorithms process data from sensors, satellite imagery, and remote sensing technologies to detect changes in environmental conditions and assess the ecological footprint of energy production facilities. For instance, AI can analyze satellite data to monitor deforestation caused by logging activities near energy infrastructure or detect pollutants in water bodies resulting from industrial operations. By providing timely and accurate insights, AI enhances environmental compliance efforts, facilitates early detection of environmental risks, and supports proactive mitigation strategies. Moreover, AI-driven environmental monitoring promotes transparency and accountability in the energy sector, enabling stakeholders to make informed decisions that prioritize environmental sustainability and ecosystem conservation.
Challenges and Risks of AI in the Energy Sector
Data Privacy and Security Concerns
The integration of Artificial Intelligence (AI) in the energy sector brings significant advancements, but it also raises critical data privacy and security concerns. AI systems rely on vast amounts of data to function effectively, including sensitive information about energy consumption patterns, grid operations, and personal data from smart home devices. The collection, storage, and processing of this data pose potential risks related to unauthorized access, data breaches, and misuse of personal information. Ensuring robust cybersecurity measures is paramount to protecting the integrity and confidentiality of the data. This includes implementing encryption, secure data storage solutions, and access controls to safeguard against cyber threats. Additionally, it is essential to establish transparent data governance policies that clearly define how data is collected, used, and shared, ensuring compliance with data protection regulations such as the General Data Protection Regulation (GDPR) and other relevant laws. By addressing data privacy and security concerns, the energy sector can build trust with consumers and stakeholders, fostering a safer and more resilient AI-driven infrastructure.
Ethical Considerations
The deployment of AI in the energy sector also necessitates careful consideration of ethical issues to ensure that the technology is used responsibly and fairly. One primary concern is the potential for bias in AI algorithms, which can lead to unequal treatment of different communities or regions in energy distribution and access. Ensuring that AI systems are designed and trained with diverse and representative data sets is crucial to mitigate bias and promote equity. Furthermore, the automation of decision-making processes in energy management raises questions about accountability and transparency. It is important to establish clear guidelines and ethical standards for AI development and deployment, ensuring that decisions made by AI systems can be audited and understood by humans. Another ethical consideration is the impact of AI on employment within the energy sector. While AI can improve efficiency and reduce costs, it may also lead to job displacement. Addressing these challenges requires a balanced approach that includes workforce retraining and support for affected employees. By proactively addressing ethical considerations, the energy sector can harness the benefits of AI while promoting fairness, transparency, and social responsibility.
Regulatory and Compliance Issues
The rapid adoption of AI in the energy sector presents regulatory and compliance challenges that must be addressed to ensure safe and effective implementation. Existing regulations may not fully account for the capabilities and implications of AI technologies, necessitating updates and new frameworks to govern their use. Regulators need to establish clear guidelines for AI development, deployment, and monitoring to ensure that AI systems comply with safety, reliability, and environmental standards. This includes defining requirements for data privacy, cybersecurity, and ethical considerations in AI applications. Additionally, compliance with international standards and regulations is essential for organizations operating in multiple jurisdictions, requiring a comprehensive understanding of different legal requirements. Collaborative efforts between industry stakeholders, policymakers, and regulatory bodies are crucial to developing a cohesive and adaptive regulatory environment that fosters innovation while safeguarding public interests. By addressing regulatory and compliance issues, the energy sector can navigate the complexities of AI integration and ensure that technological advancements contribute to sustainable and ethical energy practices.
Case Studies and Real-World Applications
AI in Renewable Energy Optimization
Artificial Intelligence (AI) is transforming the renewable energy sector by optimizing the performance and integration of renewable energy sources such as solar, wind, and hydroelectric power. AI algorithms analyze vast amounts of data from weather forecasts, historical energy production, and real-time operational metrics to predict and enhance the output of renewable energy systems. For instance, in solar power, AI can forecast sunlight patterns and adjust the orientation of solar panels to maximize energy capture. In wind energy, AI models predict wind speeds and directions to optimize turbine positioning and operation, ensuring the most efficient energy generation. Additionally, AI helps manage the variability of renewable energy sources by forecasting supply and demand, allowing grid operators to balance load and prevent disruptions. By leveraging AI, renewable energy systems can achieve higher efficiency, lower operational costs, and increased reliability, ultimately accelerating the transition to a more sustainable energy landscape.
Smart Grids and AI Implementation
The implementation of AI in smart grids represents a significant advancement in modern energy distribution and management. Smart grids equipped with AI technologies can monitor and analyze data from millions of sensors and devices in real-time, enhancing the efficiency, reliability, and flexibility of the electrical grid. AI algorithms detect patterns and predict energy demand, enabling grid operators to optimize energy distribution, reduce peak loads, and prevent outages. For example, AI can forecast energy consumption based on historical data and real-time inputs, allowing for more accurate energy allocation and load balancing. Additionally, AI facilitates the integration of distributed energy resources (DERs) such as rooftop solar panels and electric vehicles, coordinating their contributions to the grid. AI also plays a crucial role in grid maintenance by identifying potential failures and vulnerabilities, enabling proactive repairs and reducing downtime. Overall, AI implementation in smart grids enhances grid performance, supports renewable energy integration, and ensures a resilient and adaptive energy infrastructure.
AI in Energy Storage Solutions
AI is revolutionizing energy storage solutions, making them more efficient, reliable, and cost-effective. Energy storage systems, such as batteries, are critical for balancing supply and demand, especially with the increasing integration of intermittent renewable energy sources. AI algorithms optimize the charging and discharging cycles of batteries by analyzing real-time data on energy demand, grid conditions, and market prices. This optimization maximizes the lifespan and efficiency of energy storage systems while ensuring they are available when needed most. AI also enhances predictive maintenance of energy storage solutions by monitoring performance metrics and identifying early signs of degradation or faults. By predicting and preventing potential issues, AI reduces maintenance costs and improves system reliability. Furthermore, AI enables advanced energy management strategies, such as peak shaving and load shifting, by forecasting demand and strategically deploying stored energy. By leveraging AI, energy storage solutions can play a pivotal role in stabilizing the grid, supporting renewable energy integration, and enhancing overall energy system resilience and sustainability.
Future Trends in AI and Energy
The Role of AI in Achieving Sustainable Energy Goals
Artificial Intelligence (AI) is pivotal in achieving sustainable energy goals by enhancing efficiency, reducing emissions, and promoting the integration of renewable energy sources. AI technologies enable precise forecasting and optimization of energy production and consumption, ensuring that energy resources are utilized in the most efficient manner possible. For instance, AI-driven predictive analytics can forecast energy demand with high accuracy, allowing energy providers to adjust production levels and reduce waste. In renewable energy, AI optimizes the operation of solar panels and wind turbines by predicting weather patterns and adjusting settings to maximize energy capture. AI also plays a crucial role in energy storage, managing the charge and discharge cycles of batteries to ensure stability and availability. Furthermore, AI-powered smart grids facilitate the seamless integration of distributed energy resources, balancing supply and demand to prevent outages and minimize reliance on fossil fuels. By leveraging AI, the energy sector can significantly reduce its carbon footprint, enhance energy efficiency, and accelerate the transition to a sustainable energy future.
Emerging Technologies in AI and Energy
Emerging technologies in AI are driving significant advancements in the energy sector, enabling smarter, more efficient, and sustainable energy systems. One such technology is machine learning, which is being used to analyze vast datasets from energy production and consumption, optimizing everything from grid management to renewable energy forecasting. Deep learning, a subset of machine learning, enhances predictive maintenance by accurately predicting equipment failures and optimizing maintenance schedules, thereby reducing downtime and operational costs. Another emerging technology is AI-powered IoT (Internet of Things), which connects various energy devices and systems, enabling real-time data collection and analysis for improved energy management and efficiency.
Conclusion
The integration of Artificial Intelligence (AI) in the energy sector marks a transformative era characterized by enhanced efficiency, sustainability, and innovation. AI technologies are reshaping how energy is produced, distributed, and consumed, leading to significant advancements across various domains including renewable energy optimization, smart grid implementation, and energy storage solutions. By leveraging AI, the energy sector can achieve its sustainability goals, reduce carbon footprints, and ensure a resilient and reliable energy infrastructure. As the energy landscape continues to evolve, the role of AI will become increasingly critical, driving the sector towards a more sustainable and efficient future. AI has made profound impacts on the energy sector, revolutionizing traditional practices and introducing innovative solutions. AI-driven predictive analytics enhance load forecasting and energy demand management, allowing for more accurate and efficient energy distribution. In renewable energy, AI optimizes the operation of solar and wind farms, improving energy capture and reducing reliance on fossil fuels. Smart grids, empowered by AI, offer real-time monitoring and control, ensuring stability and reliability in energy supply. Furthermore, AI optimizes energy storage systems, extending battery life and enhancing their performance. AI also facilitates predictive maintenance, reducing downtime and operational costs. Overall, AI’s impact on the energy sector is substantial, leading to greater efficiency, sustainability, and resilience.
FAQs
What is the role of AI in energy efficiency?
AI plays a crucial role in enhancing energy efficiency by optimizing energy consumption and improving operational processes. AI algorithms analyze real-time data from various sources, such as smart meters, sensors, and historical usage patterns, to identify inefficiencies and recommend adjustments. For instance, AI can optimize heating, ventilation, and air conditioning (HVAC) systems by adjusting settings based on occupancy and weather forecasts, significantly reducing energy waste. Additionally, AI enables predictive maintenance of equipment, preventing energy loss due to faulty operations and ensuring optimal performance. By leveraging AI, energy providers and consumers can achieve substantial energy savings and reduce their overall energy footprint.
How does AI contribute to renewable energy production?
AI significantly enhances renewable energy production by optimizing the performance and integration of renewable energy sources. AI algorithms predict weather patterns and adjust the operations of solar panels and wind turbines to maximize energy capture. For example, AI can determine the optimal angles for solar panels based on sunlight forecasts or adjust wind turbine blades to harness the most wind energy. AI also aids in the integration of renewable energy into the grid by forecasting energy supply and demand, allowing for better load balancing and storage management. These capabilities ensure that renewable energy sources operate at peak efficiency and reliability, facilitating a more sustainable energy landscape.
What are the main challenges of implementing AI in the energy sector?
Implementing AI in the energy sector faces several challenges, including:
- Data Privacy and Security: The vast amounts of data required for AI applications raise concerns about data protection and cybersecurity. Ensuring the secure collection, storage, and processing of sensitive information is critical.
- Integration with Existing Systems: Many energy infrastructures rely on legacy systems, which can be challenging to integrate with modern AI technologies without significant upgrades or modifications.
- Cost and Investment: Developing and deploying AI solutions can be costly, requiring substantial investment in technology, infrastructure, and skilled personnel.
- Regulatory Compliance: Navigating the complex regulatory environment and ensuring compliance with various local, national, and international regulations can be daunting.
- Ethical Considerations: Addressing ethical issues such as bias in AI algorithms, transparency in decision-making, and the potential impact on employment is essential for responsible AI deployment.
Can AI help in reducing the environmental impact of energy production?
Yes, AI can significantly reduce the environmental impact of energy production by optimizing energy use, enhancing renewable energy integration, and improving efficiency across the energy sector. AI-driven predictive maintenance reduces emissions by ensuring equipment operates efficiently and preventing energy loss. In renewable energy, AI maximizes the output of solar and wind power systems, reducing reliance on fossil fuels. AI also aids in energy storage and grid management, ensuring that energy is used and distributed more efficiently, minimizing waste. By leveraging AI, the energy sector can lower its carbon footprint, reduce greenhouse gas emissions, and promote sustainable practices, contributing to global environmental conservation efforts.
What are the future trends of AI in the energy sector?
Future trends of AI in the energy sector include:
- Enhanced Smart Grids: AI will continue to improve the functionality and efficiency of smart grids, enabling better integration of distributed energy resources, real-time monitoring, and dynamic load balancing.
- Advanced Energy Storage: AI-driven advancements in energy storage technologies will improve battery performance, optimize charge-discharge cycles, and enhance grid stability.
- AI-Integrated Renewable Energy Systems: Continued integration of AI in renewable energy systems will lead to more efficient and reliable operations, maximizing energy production from solar, wind, and other renewable sources.
- Blockchain and AI Collaboration: Combining AI with blockchain technology will enhance transparency, security, and efficiency in energy transactions, facilitating peer-to-peer energy trading and more decentralized energy systems.
- Sustainable and Ethical AI: The focus will increasingly be on developing sustainable and ethical AI solutions that address data privacy, bias, and regulatory compliance while promoting social and environmental responsibility.