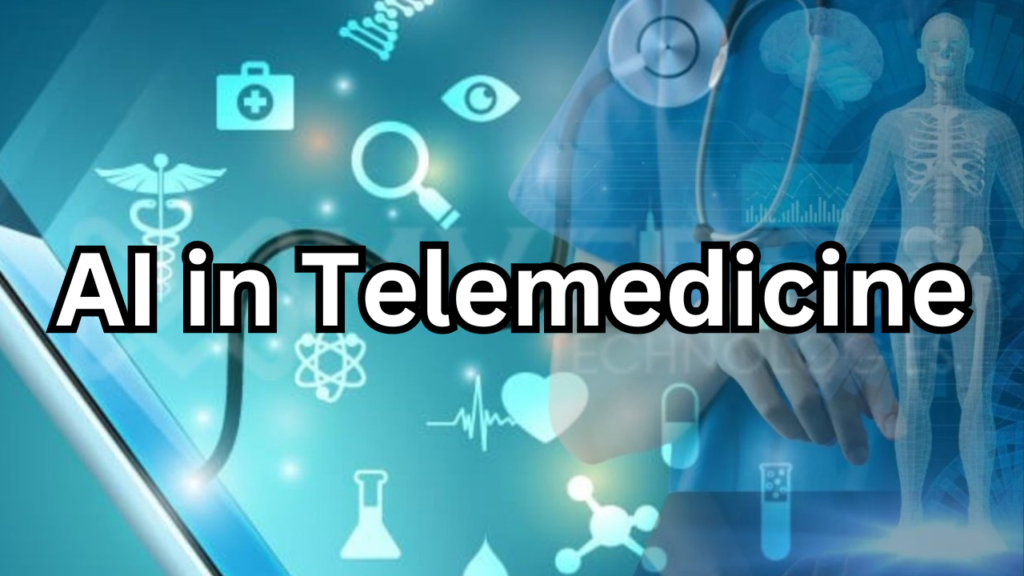
Table of Contents
Introduction
In an era defined by rapid technological advancements, the intersection of artificial intelligence (AI) and telemedicine stands at the forefront of revolutionizing healthcare delivery. This article delves into how AI is reshaping telemedicine, offering a comprehensive exploration of its applications, benefits, challenges, and future implications.
Definition of Telemedicine
Telemedicine refers to the remote diagnosis and treatment of patients using telecommunications technology. It encompasses a range of services, from consultations via video conferencing to monitoring chronic conditions through wearable devices, all conducted without the need for in-person visits to healthcare facilities.
Brief Overview of AI in Healthcare
Artificial intelligence in healthcare involves the use of sophisticated algorithms and machine learning techniques to analyze complex medical data. AI applications extend across various domains, including medical imaging interpretation, personalized treatment planning, predictive analytics, and virtual health assistants, significantly augmenting healthcare capabilities.
Importance of AI in Telemedicine
AI plays a pivotal role in enhancing telemedicine by enabling more accurate diagnostics, improving patient outcomes through personalized treatment plans, and facilitating remote patient monitoring. Its integration into telemedicine platforms not only expands access to healthcare services but also enhances efficiency and reduces costs, making quality healthcare more accessible globally.
Purpose and Scope of the Article
This article aims to provide a comprehensive overview of AI’s transformative impact on telemedicine. It explores the evolution of telemedicine, the specific applications of AI in remote healthcare delivery, benefits realized by patients and healthcare providers, as well as the ethical, legal, and technical challenges that accompany these advancements. By the end, readers will gain insights into the current landscape and future directions of AI in telemedicine, poised to shape the future of healthcare delivery worldwide.
The Evolution of Telemedicine
Historical Background of Telemedicine
The concept of telemedicine traces its roots back to the mid-20th century when advancements in telecommunications laid the groundwork for remote medical consultations. Early experiments in telemedicine involved transmitting electrocardiographic signals over telephone lines, pioneering the idea of diagnosing patients at a distance. Over subsequent decades, developments in satellite communication and video conferencing technology further expanded telemedicine capabilities, enabling real-time consultations between healthcare professionals and patients located miles apart. Today, telemedicine encompasses a diverse array of services, ranging from virtual doctor visits to remote monitoring of chronic conditions, marking a significant evolution in healthcare delivery.
Technological Advances Leading to Telemedicine
The evolution of telemedicine has been propelled by key technological advancements that have transformed healthcare accessibility and delivery. The advent of high-speed internet and mobile technology has revolutionized telecommunication capabilities, facilitating seamless transmission of medical data and enabling interactive video consultations. Innovations in wearable devices and remote monitoring technologies have empowered patients to actively participate in their healthcare management from the comfort of their homes. These technological strides have not only enhanced the efficiency of healthcare delivery but also addressed geographical barriers, particularly benefiting underserved populations and rural communities.
Role of AI in Advancing Telemedicine
Artificial intelligence (AI) stands at the forefront of advancing telemedicine by leveraging machine learning algorithms to interpret vast amounts of medical data swiftly and accurately. AI-powered diagnostic tools, such as image recognition algorithms in medical imaging, enable healthcare providers to detect anomalies with high precision, leading to timely interventions and improved patient outcomes. AI-driven predictive analytics enhance decision-making by forecasting patient health trends and recommending personalized treatment plans based on individual medical histories. Moreover, AI chatbots and virtual health assistants facilitate patient interactions, providing timely medical advice and support, thereby augmenting the efficiency and accessibility of telemedicine services. As AI continues to evolve, its integration into telemedicine promises to redefine healthcare delivery, making quality medical care more accessible and personalized than ever before.
Key Components of AI in Telemedicine
AI Algorithms and Machine Learning
AI algorithms and machine learning form the backbone of artificial intelligence applications in healthcare. These algorithms are designed to analyze vast amounts of healthcare data, ranging from patient records to medical research papers, to extract meaningful insights. Supervised learning algorithms can be trained on labeled data to perform tasks such as medical image analysis, disease diagnosis, and treatment planning. Unsupervised learning algorithms, on the other hand, can discover patterns and associations within data sets, aiding in medical research and population health management. Reinforcement learning algorithms are also being explored to optimize treatment protocols and personalized medicine strategies. Overall, AI algorithms empower healthcare providers to make data-driven decisions, enhance diagnostic accuracy, and improve patient outcomes.
Natural Language Processing (NLP)
Natural Language Processing (NLP) is a branch of artificial intelligence that focuses on enabling computers to understand, interpret, and generate human language. In healthcare, NLP plays a crucial role in processing and extracting insights from unstructured clinical text, such as electronic health records (EHRs), medical notes, and research literature. NLP algorithms can analyze patient symptoms, extract key information from medical reports, and support clinical decision-making by summarizing patient histories and identifying critical information. Furthermore, NLP-powered chatbots and virtual assistants can engage in natural language conversations with patients, providing information, scheduling appointments, and even offering preliminary medical advice. NLP’s ability to handle and interpret human language data significantly enhances the efficiency and accuracy of healthcare information management and communication.
Computer Vision in Medical Imaging
Computer vision refers to the field of artificial intelligence that enables machines to interpret and analyze visual information from the world. In medical imaging, computer vision algorithms are trained to analyze images generated from various imaging modalities, such as X-rays, CT scans, MRIs, and histopathological slides. These algorithms can automatically detect abnormalities, segment organs and tissues, classify diseases, and quantify anatomical structures with high accuracy. Computer vision in medical imaging enhances diagnostic capabilities by providing radiologists and clinicians with precise and timely insights into patient conditions. Moreover, advancements in deep learning algorithms have enabled the development of AI-powered diagnostic tools that can outperform human experts in certain tasks, leading to faster diagnosis, reduced error rates, and improved patient outcomes.
Predictive Analytics
Predictive analytics harnesses the power of AI and machine learning to forecast future trends and behaviors based on historical data patterns. In healthcare, predictive analytics models analyze patient data, including demographics, medical history, genetic information, and lifestyle factors, to predict risks, outcomes, and disease progression. These models can identify individuals at high risk of developing chronic conditions, predict hospital readmissions, optimize treatment plans, and personalize preventive care strategies. By leveraging predictive analytics, healthcare providers can proactively manage patient health, allocate resources more efficiently, and improve population health outcomes. Additionally, predictive analytics empower healthcare organizations to enhance operational efficiency, optimize revenue cycles, and streamline resource allocation based on anticipated patient needs.
Applications of AI in Telemedicine
Remote Patient Monitoring
Remote patient monitoring (RPM) utilizes technology to collect patient data outside of traditional healthcare settings, such as in the patient’s home or through wearable devices. RPM allows healthcare providers to monitor vital signs, symptoms, and other health indicators in real-time, enabling early detection of health issues and proactive intervention. AI plays a crucial role in RPM by analyzing continuous streams of data, identifying trends, and alerting healthcare providers to deviations from normal parameters. This technology enhances patient care by facilitating timely interventions, reducing hospitalizations, and improving overall patient outcomes, particularly for individuals with chronic conditions.
Virtual Consultations
Virtual consultations enable patients to interact with healthcare providers remotely via video conferencing or telecommunication platforms. AI enhances virtual consultations by improving the quality and efficiency of patient interactions. AI-powered tools can assist in medical history review, symptom assessment, and preliminary diagnosis before or during the consultation. Natural language processing (NLP) enables virtual assistants to understand and respond to patient queries, schedule appointments, and provide educational information. Virtual consultations supported by AI offer patients greater convenience, reduce travel time and costs, and expand access to specialized healthcare services, especially in underserved or remote areas.
AI-Powered Chatbots
AI-powered chatbots are virtual agents that use artificial intelligence and natural language processing to simulate human conversation. In healthcare, AI chatbots interact with patients to provide information, answer queries, schedule appointments, and offer basic medical advice. These chatbots can triage patient symptoms, directing urgent cases to healthcare providers while providing reassurance and guidance to others. AI chatbots improve healthcare accessibility by offering round-the-clock support, reducing waiting times for non-urgent inquiries, and empowering patients to manage their health proactively. As AI continues to evolve, chatbots are becoming increasingly sophisticated, capable of understanding complex medical terminology and adapting responses based on individual patient data.
AI in Diagnostic Support
AI in diagnostic support involves the use of artificial intelligence and machine learning algorithms to assist healthcare providers in medical diagnosis and decision-making. AI algorithms analyze medical images, laboratory results, and patient data to detect abnormalities, identify patterns, and generate diagnostic insights with high accuracy. In radiology, AI-powered image analysis can expedite the interpretation of X-rays, CT scans, and MRIs, improving diagnostic speed and accuracy. AI algorithms can also integrate clinical data from electronic health records (EHRs) to support differential diagnosis and recommend personalized treatment options based on patient-specific factors. AI in diagnostic support enhances clinical efficiency, reduces diagnostic errors, and enhances patient care outcomes by providing timely and reliable diagnostic information.
Personalized Treatment Plans
Personalized treatment plans leverage AI and machine learning to tailor medical treatments to the individual characteristics and needs of each patient. AI analyzes vast amounts of patient data, including genetic information, biomarkers, medical history, lifestyle factors, and treatment outcomes, to predict optimal treatment strategies. AI algorithms can identify patient-specific biomarkers or genetic mutations that influence disease progression and response to therapy. This personalized approach enables healthcare providers to optimize treatment efficacy, minimize adverse effects, and improve patient adherence to treatment regimens. Personalized treatment plans supported by AI empower healthcare providers to deliver precision medicine, offering patients targeted therapies that maximize therapeutic benefit and enhance overall health outcomes.
Benefits of AI in Telemedicine
Increased Accessibility to Healthcare
AI technologies significantly enhance accessibility to healthcare by overcoming geographical barriers and improving service delivery. Telemedicine platforms supported by AI enable patients in remote or underserved areas to access specialist care without traveling long distances. Virtual consultations and AI-powered chatbots provide immediate medical advice and support, reducing wait times for appointments. Moreover, remote patient monitoring allows continuous health monitoring from home, particularly beneficial for elderly or chronically ill patients. AI-driven healthcare solutions bridge gaps in healthcare access, making medical expertise more available and accessible to diverse populations globally.
Cost-Effectiveness
AI in healthcare introduces cost-effective solutions by streamlining processes, reducing operational expenses, and optimizing resource allocation. Telemedicine reduces healthcare costs by minimizing in-person visits, lowering transportation expenses, and preventing unnecessary hospitalizations. AI-powered diagnostic tools enhance diagnostic accuracy, reducing repeat tests and avoiding unnecessary procedures, thus lowering overall healthcare expenditures. Predictive analytics in healthcare management predict patient needs, optimizing treatment plans and resource utilization. By enhancing efficiency and preventive care, AI contributes to cost savings while improving healthcare quality and patient satisfaction.
Improved Patient Outcomes
AI advancements in healthcare lead to improved patient outcomes through personalized treatment plans, early disease detection, and proactive interventions. AI algorithms analyze vast patient data to predict disease risks, customize treatment options, and optimize medication dosages based on individual responses. AI in diagnostic support enhances accuracy and speed of diagnoses, reducing diagnostic errors and improving treatment efficacy. Remote patient monitoring allows timely intervention and management of chronic conditions, preventing complications and improving patient health outcomes over time. By focusing on preventive care and personalized medicine, AI enhances overall patient well-being and quality of life.
Enhanced Efficiency for Healthcare Providers
AI technologies enhance efficiency for healthcare providers by automating routine tasks, optimizing workflows, and reducing administrative burdens. AI-driven algorithms streamline medical image analysis, allowing radiologists to interpret scans faster and prioritize urgent cases. Virtual health assistants powered by AI manage appointment scheduling, patient inquiries, and administrative tasks, freeing up healthcare professionals to focus on patient care. Predictive analytics identify high-risk patients and recommend preventive measures, allowing proactive care management and reducing hospital readmissions. AI enhances clinical decision-making by providing evidence-based insights and treatment recommendations, optimizing healthcare delivery and improving operational efficiency.
Reduced Healthcare Disparities
AI in healthcare plays a pivotal role in reducing healthcare disparities by improving access, quality, and equity in healthcare delivery. Telemedicine and AI-powered diagnostics expand access to specialized care for underserved populations, rural communities, and patients in remote areas. AI-enabled language translation and cultural sensitivity tools enhance communication and understanding between healthcare providers and diverse patient populations. Personalized treatment plans tailored by AI consider individual patient factors, optimizing care outcomes across diverse demographic groups. By addressing barriers to healthcare access and providing equitable care solutions, AI contributes to narrowing healthcare disparities and promoting health equity globally.
AI and Remote Patient Monitoring
Overview of Remote Patient Monitoring
Remote patient monitoring (RPM) involves the use of technology to collect and transmit health data from patients located outside traditional healthcare settings to healthcare providers. This continuous monitoring enables healthcare professionals to track patient health metrics such as blood pressure, heart rate, glucose levels, and more, in real-time. RPM devices include wearable sensors, mobile apps, and remote monitoring platforms that transmit data securely to healthcare providers, facilitating timely intervention and proactive management of chronic conditions. RPM enhances patient care by detecting health changes early, reducing hospitalizations, improving patient adherence to treatment plans, and promoting patient engagement in self-care.
AI in Chronic Disease Management
AI plays a transformative role in chronic disease management by leveraging machine learning algorithms to analyze vast amounts of patient data and provide personalized care plans. AI algorithms can predict disease progression, identify high-risk patients, and recommend tailored interventions to manage chronic conditions such as diabetes, hypertension, cardiovascular diseases, and respiratory disorders. AI-powered tools monitor patient symptoms and health trends, optimize medication regimens, and promote lifestyle modifications based on individual patient data. By integrating AI into chronic disease management, healthcare providers can deliver proactive, patient-centered care that improves outcomes, enhances quality of life, and reduces healthcare costs associated with managing chronic conditions.
Real-Time Health Data Analysis
Real-time health data analysis involves the continuous monitoring and analysis of patient health data to provide actionable insights for healthcare decision-making. AI algorithms process real-time data streams from RPM devices, electronic health records (EHRs), and other sources to detect patterns, trends, and anomalies in patient health metrics. This analysis enables healthcare providers to make timely clinical decisions, adjust treatment plans, and intervene promptly in case of health emergencies. Real-time health data analysis enhances diagnostic accuracy, supports early disease detection, and facilitates personalized medicine by tailoring interventions based on current patient health status. This proactive approach to healthcare management improves patient outcomes and enhances healthcare delivery efficiency.
Case Studies and Success Stories
Case studies and success stories highlight the transformative impact of AI and remote patient monitoring in healthcare. For example, in chronic disease management, AI algorithms have helped reduce hospital readmissions by predicting exacerbations in conditions like heart failure or COPD. Real-time health data analysis has enabled early detection of deteriorating health conditions, prompting timely interventions that prevent complications and improve patient outcomes. Success stories demonstrate how RPM devices and AI-powered tools have empowered patients to actively participate in their healthcare, leading to better symptom management, increased treatment adherence, and enhanced quality of life. These examples underscore the potential of AI-driven healthcare innovations to revolutionize patient care and improve health outcomes across diverse populations.
Virtual Consultations and AI
AI in Video Consultations
AI enhances video consultations by improving the efficiency, accuracy, and overall experience for both healthcare providers and patients. During video consultations, AI-powered tools can assist healthcare professionals by summarizing patient medical records, highlighting relevant information, and providing real-time decision support based on patient data and symptoms. Natural language processing (NLP) algorithms enable AI to understand and interpret patient queries, facilitating more meaningful and productive conversations between doctors and patients. Additionally, AI can automate administrative tasks such as scheduling follow-up appointments and sending reminders, allowing healthcare providers to focus more on patient care during video consultations.
Enhancing Doctor-Patient Interaction with AI
AI technologies enhance doctor-patient interactions by personalizing the healthcare experience, improving communication, and optimizing care delivery. AI-powered virtual health assistants can engage in natural language conversations with patients, answering common medical questions, providing educational resources, and offering personalized health advice based on individual health data. AI-enhanced communication tools translate medical jargon into layman’s terms, ensuring patients understand their diagnoses, treatment options, and medication instructions. Moreover, AI analytics can analyze doctor-patient interactions to identify communication patterns and improve patient satisfaction by tailoring care plans to individual preferences and needs.
AI for Triage and Initial Assessments
AI plays a critical role in triage and initial assessments by efficiently categorizing patient symptoms, prioritizing cases based on urgency, and recommending appropriate interventions. AI algorithms analyze patient-reported symptoms, medical history, and vital signs to generate preliminary assessments, allowing healthcare providers to prioritize high-risk patients and allocate resources effectively. AI-powered triage systems can streamline emergency room workflows by expediting the evaluation process and reducing waiting times for critical patients. In non-emergency settings, AI facilitates remote triage through virtual assessments, enabling timely interventions and reducing unnecessary visits to healthcare facilities.
Success Stories in Virtual Consultations
Success stories in virtual consultations highlight the benefits of AI-enabled telemedicine in improving healthcare access, patient outcomes, and operational efficiency. For example, AI-driven diagnostic tools have enabled healthcare providers to diagnose and treat patients remotely with high accuracy, reducing the need for in-person visits and minimizing travel-related expenses for patients. Virtual consultations supported by AI have expanded access to specialist care for rural and underserved communities, bridging geographical barriers and ensuring timely medical advice and treatment. Moreover, AI-powered virtual health assistants have enhanced patient engagement and satisfaction by providing personalized care, continuous support, and proactive health management tips. These success stories underscore the transformative impact of AI in virtual consultations, paving the way for more accessible, efficient, and patient-centered healthcare delivery models.
AI-Powered Diagnostic Tools
AI in Medical Imaging and Radiology
AI has revolutionized medical imaging and radiology by enhancing diagnostic accuracy, speeding up image analysis, and improving patient outcomes. AI algorithms trained on vast datasets can analyze medical images such as X-rays, CT scans, MRIs, and mammograms with a level of precision that rivals or exceeds human capabilities. Deep learning models can detect abnormalities, tumors, fractures, and other medical conditions from images, aiding radiologists in making timely and accurate diagnoses. AI in medical imaging also includes image segmentation, where algorithms identify and outline anatomical structures and lesions, providing detailed insights for treatment planning and surgical interventions. Overall, AI in medical imaging transforms healthcare by optimizing workflow efficiency, reducing diagnostic errors, and ultimately improving patient care.
AI for Pathology and Lab Tests
AI applications in pathology and laboratory testing automate and enhance the analysis of tissue samples, blood tests, and other diagnostic specimens. AI-powered image analysis algorithms can analyze digital pathology slides, identifying cancerous cells, grading tumors, and predicting disease prognosis with high accuracy. Natural language processing (NLP) algorithms assist in interpreting and summarizing pathology reports, extracting key information for clinical decision-making. AI in laboratory testing accelerates test result analysis, detects anomalies in biochemical markers, and predicts patient responses to treatments based on genetic and molecular profiling. By augmenting pathologists’ capabilities and improving diagnostic precision, AI transforms pathology and laboratory medicine, enabling faster diagnoses and personalized treatment recommendations.
Predictive Diagnostics and Early Detection
AI-driven predictive diagnostics and early detection algorithms leverage machine learning to analyze patient data and identify patterns indicative of disease onset or progression. These algorithms integrate clinical data, genetic information, biomarkers, and patient history to predict disease risks and forecast health outcomes. For example, predictive models can assess cardiovascular risk factors, predict the onset of chronic diseases like diabetes or hypertension, and stratify patients based on their likelihood of developing complications. Early detection algorithms utilize AI to detect subtle changes in medical images, biomarkers, or patient symptoms that may indicate early stages of disease, enabling timely interventions and preventive measures. By focusing on proactive healthcare management, AI-powered predictive diagnostics improve patient outcomes, reduce healthcare costs, and enhance population health management strategies.
Case Studies of AI Diagnostic Tools
Case studies of AI diagnostic tools showcase their effectiveness in improving diagnostic accuracy, workflow efficiency, and patient care across various medical specialties. For instance, AI-powered algorithms for mammography have demonstrated superior performance in detecting breast cancer lesions compared to traditional screening methods, leading to earlier detection and improved survival rates. In neuroimaging, AI algorithms analyze MRI scans to detect brain tumors, hemorrhages, and neurological disorders with high sensitivity and specificity, facilitating prompt treatment planning and surgical interventions. In dermatology, AI-driven image analysis tools assist dermatologists in diagnosing skin conditions, distinguishing between benign and malignant lesions, and recommending appropriate treatments. These case studies illustrate how AI diagnostic tools enhance clinical decision-making, reduce diagnostic errors, and contribute to better health outcomes for patients worldwide.
Personalized Treatment Plans with AI
AI in Genomic Medicine
AI plays a pivotal role in advancing genomic medicine by analyzing vast amounts of genetic data to identify patterns, mutations, and biomarkers associated with disease susceptibility, progression, and treatment response. Genomic sequencing generates massive datasets that AI algorithms can analyze to uncover genetic variations relevant to individual health risks and conditions. AI-powered bioinformatics tools assist in interpreting genomic data, predicting disease risks, and recommending personalized treatment strategies based on genetic profiles. In cancer care, AI in genomic medicine identifies targetable mutations and informs precision therapies tailored to individual tumor characteristics. By integrating AI into genomic medicine, healthcare providers can deliver more precise diagnoses, optimize treatment selection, and improve outcomes for patients with genetic disorders and complex diseases.
Tailoring Treatments Based on Patient Data
Tailoring treatments based on patient data involves using AI and machine learning to analyze diverse patient factors, including genetic profiles, biomarkers, medical history, lifestyle data, and treatment responses. AI algorithms integrate multidimensional data to generate personalized treatment plans that optimize therapeutic efficacy, minimize adverse effects, and improve patient outcomes. Personalized treatment approaches may involve selecting targeted therapies, adjusting medication dosages, and implementing lifestyle interventions tailored to individual patient needs. AI-driven predictive models predict patient responses to treatments, identify optimal drug combinations, and optimize treatment schedules based on real-time health data. By tailoring treatments to patient-specific characteristics, healthcare providers can achieve better treatment outcomes, enhance patient satisfaction, and promote precision medicine practices.
Benefits of Personalized Medicine
Personalized medicine offers several benefits that revolutionize healthcare delivery and improve patient care outcomes. Firstly, personalized medicine tailors treatment plans to individual patient characteristics, maximizing therapeutic efficacy and minimizing adverse effects. By considering genetic factors, biomarkers, and lifestyle data, personalized medicine optimizes treatment selection and dosing, leading to improved patient adherence and treatment outcomes. Secondly, personalized medicine fosters preventive care strategies by identifying individuals at high risk of developing certain diseases and implementing targeted interventions to reduce risks and promote wellness. Thirdly, personalized medicine enhances healthcare efficiency by reducing unnecessary treatments and hospitalizations, optimizing resource allocation, and lowering overall healthcare costs. Ultimately, personalized medicine shifts healthcare paradigms from reactive to proactive, empowering patients with tailored healthcare solutions that prioritize individual health needs and improve quality of life.
Success Stories of Personalized Treatment Plans
Success stories of personalized treatment plans highlight the transformative impact of personalized medicine in improving patient outcomes and quality of life. For instance, in oncology, personalized treatment plans based on genetic testing and tumor profiling have resulted in better responses to targeted therapies, extended survival rates, and improved overall survival for cancer patients. In cardiology, personalized treatment plans tailored to cardiovascular risk profiles and genetic predispositions have reduced cardiovascular events and improved heart health outcomes. Success stories also demonstrate the effectiveness of personalized medicine in managing chronic diseases like diabetes, autoimmune disorders, and rare genetic conditions, where individualized treatment strategies optimize disease management and enhance patient well-being. These case studies underscore the potential of personalized medicine to revolutionize healthcare delivery, paving the way for more precise, effective, and patient-centered treatment approaches across diverse medical specialties.
AI in Emergency Telemedicine
Role of AI in Emergency Situations
AI plays a crucial role in emergency situations by enhancing response times, improving decision-making, and optimizing resource allocation in high-pressure environments. In emergency medicine, AI algorithms analyze real-time data from multiple sources, including patient vital signs, medical history, and environmental factors, to prioritize cases based on severity and urgency. AI-powered predictive models can forecast patient outcomes, anticipate complications, and recommend appropriate interventions to healthcare providers in emergency settings. AI-enhanced decision support systems aid in rapid diagnosis, treatment planning, and triage, ensuring timely interventions for critical patients. By leveraging AI technologies, emergency medical teams can improve response efficiency, reduce mortality rates, and enhance overall patient care outcomes during critical incidents and mass casualty events.
AI-Powered Triage Systems
AI-powered triage systems automate and optimize the triage process by rapidly assessing patient symptoms, prioritizing cases based on severity, and recommending appropriate levels of care. These systems use machine learning algorithms to analyze patient-reported symptoms, vital signs, and medical history, enabling healthcare providers to make informed triage decisions quickly and accurately. AI triage tools can classify patients into categories such as emergent, urgent, or non-urgent, facilitating resource allocation and workflow management in emergency departments. Virtual triage platforms supported by AI enhance healthcare access by remotely assessing patient conditions and directing individuals to appropriate care settings, reducing overcrowding in emergency rooms and improving patient flow. AI-powered triage systems streamline emergency response operations, optimize clinical outcomes, and ensure that critical patients receive timely medical attention in urgent situations.
Case Studies in Emergency Telemedicine
Case studies in emergency telemedicine demonstrate the effectiveness of telehealth technologies and AI-driven solutions in improving emergency medical care delivery and patient outcomes. For example, in stroke care, telemedicine platforms equipped with AI-powered imaging analysis tools enable remote neurologists to quickly assess brain scans, diagnose strokes, and recommend time-sensitive treatments like thrombolytic therapy. In trauma care, teleconsultation with trauma specialists via video conferencing facilitates rapid assessment, triage decisions, and treatment planning for critically injured patients in remote or underserved areas. Virtual emergency telemedicine services supported by AI enhance access to specialized care, reduce time to treatment, and improve survival rates for patients experiencing acute medical emergencies. These case studies highlight the transformative impact of emergency telemedicine and AI technologies in enhancing emergency response capabilities, optimizing patient care delivery, and saving lives in critical situations.
Future Trends in AI and Telemedicine
Emerging Technologies in AI and Telemedicine
Emerging technologies in AI and telemedicine represent a dynamic frontier in healthcare innovation, promising to reshape the delivery of medical services worldwide. AI-powered telemedicine platforms leverage advanced algorithms to enable remote consultations, diagnosis, and treatment planning, transcending geographical barriers and expanding access to specialized healthcare expertise. These technologies include AI-driven diagnostic tools that analyze medical images with unprecedented accuracy, virtual health assistants capable of natural language processing for patient interactions, and remote monitoring devices that track vital signs and health metrics in real-time. By integrating AI into telemedicine, healthcare providers can deliver personalized care, enhance diagnostic precision, and improve patient outcomes while optimizing healthcare delivery efficiency. As these technologies evolve, they hold the potential to revolutionize healthcare access, quality, and affordability on a global scale, ushering in a new era of patient-centered, technology-enabled healthcare delivery.
Future Research Directions
Future research in AI and telemedicine is poised to explore transformative possibilities across various domains of healthcare. Key research directions include advancing AI algorithms for predictive analytics and early disease detection, enhancing interoperability and security standards in telemedicine platforms, and optimizing AI-driven decision support systems for clinical decision-making. Researchers are also focusing on integrating AI with emerging technologies such as wearable devices, internet of medical things (IoMT), and blockchain to enable seamless data exchange, improve patient monitoring capabilities, and ensure data privacy and security. Moreover, future research will delve into ethical considerations, regulatory frameworks, and societal impacts of AI in telemedicine to foster responsible innovation and equitable healthcare access. By addressing these research priorities, healthcare stakeholders can harness the full potential of AI and telemedicine to address healthcare challenges, improve patient outcomes, and transform healthcare delivery models worldwide.
Potential Impact on Global Healthcare Systems
The potential impact of AI and telemedicine on global healthcare systems is profound, offering transformative solutions to address healthcare disparities, improve quality of care, and optimize resource utilization. AI-powered telemedicine has the capacity to expand access to healthcare services in underserved and remote regions, enabling timely diagnosis, treatment, and management of diseases without geographic constraints. By facilitating remote consultations, AI enhances healthcare delivery efficiency, reduces healthcare costs associated with unnecessary hospital visits, and alleviates strain on healthcare infrastructure. Moreover, AI-driven predictive analytics and personalized medicine approaches can optimize treatment outcomes, minimize adverse effects, and tailor interventions based on individual patient characteristics, thereby enhancing patient satisfaction and healthcare provider productivity. However, realizing the full potential of AI in telemedicine requires addressing challenges such as regulatory frameworks, data interoperability, workforce readiness, and ensuring equitable access to technology-enabled healthcare solutions. As healthcare systems embrace AI and telemedicine innovations, they have the opportunity to foster sustainable healthcare practices, improve population health outcomes, and promote global health equity in an increasingly interconnected world.
Ethical and Legal Considerations
Ethical Implications of AI in Telemedicine
The integration of AI into telemedicine raises several ethical considerations that warrant careful scrutiny. Firstly, patient privacy and data security are paramount concerns, as AI systems require access to sensitive medical information to operate effectively. Ensuring robust data encryption, secure storage practices, and informed patient consent procedures are essential to safeguard patient confidentiality and trust. Secondly, AI algorithms must prioritize patient welfare and safety, maintaining transparency in their decision-making processes to ensure healthcare providers understand and can justify treatment recommendations. Ethical guidelines must govern the use of AI to avoid over-reliance on technology and preserve the human-centered aspect of healthcare interactions.
Legal Framework and Compliance Issues
The deployment of AI in telemedicine necessitates a robust legal framework to address regulatory compliance, liability, and patient rights. Legal considerations encompass data protection regulations such as the Health Insurance Portability and Accountability Act (HIPAA) in the United States or the General Data Protection Regulation (GDPR) in Europe, which govern the collection, storage, and use of patient health information. Healthcare providers and AI developers must adhere to these regulations to safeguard patient privacy and avoid legal repercussions.
Addressing Bias in AI Algorithms
Addressing bias in AI algorithms used in telemedicine is critical to ensure fairness, accuracy, and equitable healthcare outcomes for diverse patient populations. Bias can arise from various sources, including imbalanced training data, algorithm design flaws, and implicit biases embedded in decision-making processes. Biased AI algorithms may lead to disparities in diagnosis, treatment recommendations, and patient outcomes, particularly affecting minority groups and underrepresented populations.
The Role of Big Data in AI and Telemedicine
Importance of Big Data in AI Applications
Big data plays a crucial role in advancing AI applications by providing vast and diverse datasets that fuel machine learning algorithms and enhance predictive analytics. In healthcare, big data encompasses large volumes of patient health records, medical images, genomic sequences, wearable device data, and population health statistics. AI algorithms leverage big data to identify patterns, correlations, and insights that inform clinical decision-making, personalized treatment plans, and public health interventions. For example, in telemedicine, big data analytics enable healthcare providers to analyze patient trends, predict disease outbreaks, and optimize healthcare resource allocation based on real-time data. By harnessing big data, AI applications in healthcare can improve diagnostic accuracy, enhance treatment outcomes, and drive innovation in healthcare delivery models.
Data Collection and Analysis
Effective data collection and analysis are essential components of leveraging big data for AI applications in healthcare and telemedicine. Data collection involves acquiring structured and unstructured data from electronic health records (EHRs), medical imaging systems, wearable devices, and patient-reported outcomes. Quality data collection practices ensure data integrity, completeness, and compliance with privacy regulations such as HIPAA or GDPR to protect patient confidentiality. Data analysis techniques, including statistical modeling, machine learning algorithms, and natural language processing (NLP), extract actionable insights from big data sets, uncovering hidden patterns, and predicting healthcare outcomes. In telemedicine, data analysis facilitates real-time monitoring of patient health metrics, personalized treatment recommendations, and proactive interventions based on predictive analytics. By integrating robust data collection and analysis strategies, healthcare providers can harness the power of big data to enhance clinical decision-making, improve patient care quality, and optimize healthcare system performance.
Enhancing Telemedicine with Big Data Insights
Big data insights enhance telemedicine by enabling data-driven decision-making, personalized patient care, and operational efficiency improvements. AI algorithms analyze large-scale data sets from telemedicine platforms, patient health records, and remote monitoring devices to optimize telehealth services. For instance, predictive analytics in telemedicine leverage big data to forecast patient health trends, identify high-risk individuals, and tailor preventive care interventions. Real-time data analytics enable healthcare providers to monitor patient progress, adjust treatment plans promptly, and intervene proactively in case of health emergencies.
Conclusion
In conclusion, the integration of AI into telemedicine represents a transformative shift in healthcare delivery, offering unprecedented opportunities to enhance patient care, improve diagnostic accuracy, and optimize healthcare resources. Throughout this exploration, we’ve highlighted the pivotal role of AI in telemedicine, from its ability to facilitate remote consultations and personalized treatment plans to its impact on emergency response and population health management. Key to its success is the utilization of big data for predictive analytics, ensuring informed decision-making and proactive healthcare interventions. As AI continues to evolve, ethical considerations such as patient privacy, bias mitigation, and regulatory compliance remain crucial for maintaining trust and maximizing its benefits. Looking ahead, the future of AI in telemedicine holds promise for advancing healthcare access, reducing disparities, and fostering a more resilient healthcare ecosystem globally.
(FAQs)
What is telemedicine, and how does AI enhance it?
Telemedicine refers to the remote delivery of healthcare services using telecommunications technology. AI enhances telemedicine by improving diagnostic accuracy through image analysis, facilitating virtual consultations with AI-powered chatbots, and enabling predictive analytics for personalized treatment plans. AI also enhances remote monitoring of patient health metrics, ensuring timely interventions and proactive healthcare management.
What are the main benefits of AI in telemedicine?
The main benefits of AI in telemedicine include improved access to healthcare services, especially in underserved areas; enhanced diagnostic accuracy through AI-driven image analysis and diagnostic tools; personalized treatment plans based on patient data and predictive analytics; efficiency gains in healthcare delivery, reducing waiting times and optimizing resource allocation; and the ability to monitor patient health remotely, leading to early intervention and improved patient outcomes.
What challenges exist in implementing AI in telemedicine?
Challenges in implementing AI in telemedicine include ensuring data privacy and security, navigating regulatory compliance, addressing biases in AI algorithms that may affect healthcare outcomes, integrating AI seamlessly into existing healthcare workflows, ensuring interoperability of telemedicine platforms, and overcoming barriers related to technological infrastructure and digital literacy among patients and healthcare providers.
How does AI improve remote patient monitoring?
AI improves remote patient monitoring by analyzing continuous streams of patient health data, such as vital signs, activity levels, and medication adherence. AI algorithms detect anomalies, predict health deteriorations, and alert healthcare providers to intervene promptly. This real-time monitoring enhances patient safety, reduces hospital readmissions, and supports chronic disease management by enabling proactive adjustments to treatment plans based on individual patient responses.
Are there ethical concerns with AI in telemedicine?
Yes, ethical concerns with AI in telemedicine include patient privacy and data security risks associated with storing and transmitting sensitive health information. There are also concerns about the potential for biases in AI algorithms, which could lead to unequal treatment or diagnostic errors. Ethical considerations include ensuring informed consent for AI-driven healthcare interventions, maintaining transparency in AI decision-making processes, and addressing the impact of AI on the doctor-patient relationship and healthcare provider accountability. Balancing technological advancements with ethical standards is crucial to realizing the full potential of AI in telemedicine while upholding patient trust and welfare.