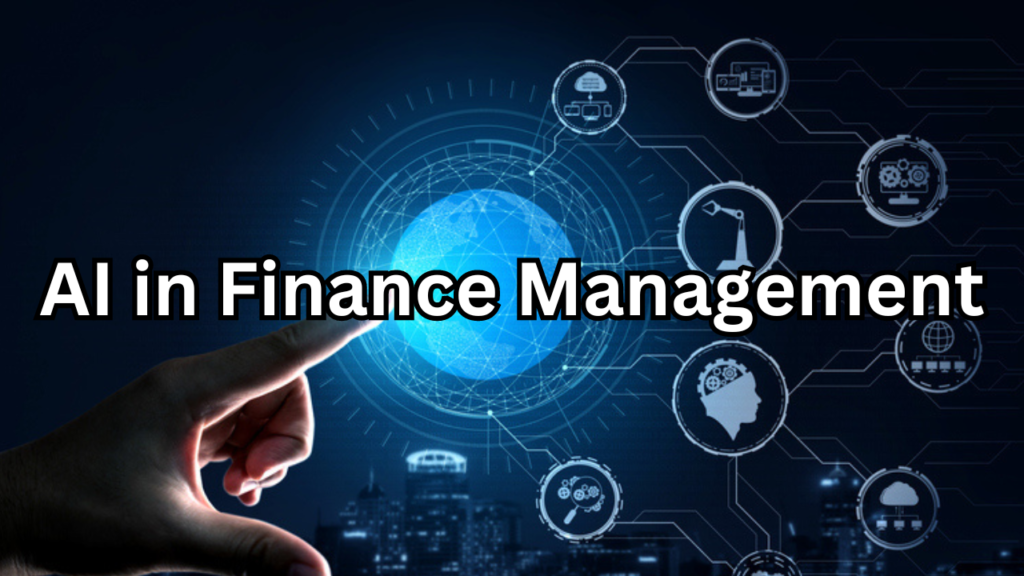
Table of Contents
Introduction
Overview of AI in Finance Management
Artificial Intelligence (AI) has revolutionized numerous industries, and finance management is no exception. AI in finance management encompasses a range of technologies, including machine learning, natural language processing, and robotics process automation. These technologies work together to analyze massive amounts of data, identify patterns, and make informed decisions with minimal human intervention. From automating mundane tasks to offering predictive analytics and personalized financial advice, AI is reshaping the way financial services are delivered and consumed.
In the realm of finance, AI applications are vast and varied. They include fraud detection, customer service automation, risk management, investment strategies, personal finance management, corporate finance, and more. The integration of AI in financial operations not only enhances efficiency but also provides deeper insights and fosters innovative solutions to complex financial challenges.
Importance of AI in the Modern Financial Landscape
The importance of AI in the modern financial landscape cannot be overstated. As the financial industry grapples with increasing volumes of data and a demand for real-time decision-making, AI emerges as a crucial tool. Here are several reasons why AI is indispensable in today’s financial environment:
- Efficiency and Automation: AI automates repetitive and time-consuming tasks, such as data entry, transaction processing, and compliance checks. This leads to significant cost savings and allows human workers to focus on more strategic activities.
- Enhanced Accuracy: AI algorithms reduce human error by performing precise calculations and analysis. This is particularly vital in areas like risk assessment and fraud detection, where accuracy is paramount.
- Predictive Analytics: AI’s ability to analyze historical data and predict future trends is invaluable for financial planning and investment strategies. It helps institutions anticipate market movements, optimize portfolios, and make data-driven decisions.
- Improved Customer Experience: AI-powered chatbots and virtual assistants provide 24/7 customer support, answering queries, and offering personalized financial advice. This enhances customer satisfaction and loyalty.
- Risk Management: AI tools can identify potential risks by analyzing patterns and anomalies in data. This proactive approach to risk management helps financial institutions mitigate threats before they escalate.
- Regulatory Compliance: Financial institutions face stringent regulatory requirements. AI helps in ensuring compliance by continuously monitoring transactions and activities for suspicious behavior, thereby reducing the risk of regulatory breaches.
- Scalability: AI systems can easily scale to handle increasing amounts of data and transactions, making them suitable for growing financial institutions.
The Evolution of AI in Finance
Historical Perspective
The integration of artificial intelligence (AI) in finance management has a rich history that dates back several decades. The journey began in the mid-20th century with the advent of computers, which laid the groundwork for automating basic financial tasks. Early applications of AI in finance emerged in the 1980s and 1990s, primarily focusing on algorithmic trading and fraud detection. These initial implementations were relatively rudimentary, relying on simple rule-based systems and basic statistical models. However, as computational power and data availability increased, so did the sophistication of AI applications. The late 20th and early 21st centuries saw the rise of machine learning techniques, which enabled more complex and accurate financial predictions and analyses. This period marked the beginning of a transformative era where AI began to play a more central role in financial decision-making and operations.
Technological Advancements
The technological advancements in AI have been pivotal in revolutionizing finance management. The development of machine learning algorithms, particularly deep learning, has significantly enhanced the ability to process and analyze vast amounts of financial data. Natural language processing (NLP) has enabled the extraction of valuable insights from unstructured data, such as news articles, social media posts, and financial reports. Additionally, advancements in robotics process automation (RPA) have facilitated the automation of repetitive tasks, such as data entry and transaction processing, thereby improving efficiency and reducing human error. Cloud computing has also played a crucial role by providing the necessary infrastructure to store and process large datasets, making advanced AI applications more accessible to financial institutions of all sizes. These technological innovations have collectively transformed AI from a theoretical concept into a practical tool that drives significant improvements in finance management.
Current Trends
In the current landscape, AI continues to make profound impacts on finance management, driven by several emerging trends. One notable trend is the increasing use of AI for predictive analytics, where machine learning models are employed to forecast market trends, asset prices, and customer behaviors. This allows financial institutions to make more informed decisions and develop proactive strategies. Another trend is the growing adoption of AI-powered chatbots and virtual assistants, which enhance customer service by providing instant responses and personalized advice. AI is also being used extensively in fraud detection and risk management, where it can identify unusual patterns and potential threats in real-time, significantly reducing the incidence of financial fraud. Additionally, there is a rising focus on ethical AI and regulatory compliance, as financial institutions seek to ensure that their AI systems are transparent, fair, and aligned with regulatory standards. These trends highlight the dynamic nature of AI in finance management and its continuous evolution in response to new challenges and opportunities.
Key AI in Finance Management
Machine Learning
Machine learning (ML) is at the heart of AI-driven finance management, enabling systems to learn from historical data and improve their performance over time without explicit programming. In the financial sector, machine learning algorithms are widely used for a variety of applications, including credit scoring, algorithmic trading, and customer segmentation. By analyzing vast amounts of data, ML models can identify patterns and correlations that are often imperceptible to human analysts. For instance, in credit scoring, machine learning can evaluate a borrower’s risk by examining numerous variables beyond traditional credit scores, leading to more accurate and inclusive lending decisions. Similarly, in trading, ML algorithms can process and react to market signals in real-time, executing trades at optimal times to maximize returns. The ability of machine learning to adapt and refine its models based on new data makes it an invaluable tool in the ever-evolving financial landscape.
Natural Language Processing
Natural language processing (NLP) is a branch of AI that focuses on the interaction between computers and human language. In finance management, NLP plays a crucial role in extracting actionable insights from unstructured data sources such as news articles, financial reports, social media posts, and customer communications. By understanding and analyzing textual information, NLP can help financial institutions gauge market sentiment, monitor news for impactful events, and enhance customer service. For example, sentiment analysis using NLP can provide traders and investors with insights into market moods and trends, influencing investment decisions. Additionally, NLP-powered chatbots and virtual assistants can engage with customers in natural language, answering queries, providing financial advice, and handling routine transactions, thus improving customer satisfaction and operational efficiency. The ability of NLP to interpret and generate human language makes it an essential component of modern financial services.
Robotics Process Automation
Robotics Process Automation (RPA) involves using software robots or “bots” to automate repetitive, rule-based tasks traditionally performed by humans. In finance management, RPA is employed to streamline various back-office operations, such as data entry, account reconciliation, transaction processing, and regulatory reporting. By automating these mundane tasks, RPA not only enhances efficiency and accuracy but also frees up human employees to focus on more strategic and value-added activities. For instance, in banking, RPA can handle the processing of loan applications by automatically extracting and validating information from various documents, thereby speeding up the approval process. Moreover, RPA ensures compliance by consistently executing processes according to predefined rules, reducing the risk of errors and regulatory breaches. The scalability and flexibility of RPA make it a powerful tool for optimizing financial operations and achieving higher productivity.
Predictive Analytics
Predictive analytics leverages statistical algorithms and machine learning techniques to analyze historical data and make predictions about future events. In finance management, predictive analytics is extensively used to forecast market trends, assess investment risks, and anticipate customer behaviors. By utilizing data from various sources, such as financial statements, market data, and economic indicators, predictive models can provide insights that help financial institutions make informed decisions. For example, in portfolio management, predictive analytics can identify potential investment opportunities and risks, enabling fund managers to optimize their asset allocations. In customer relationship management, predictive analytics can forecast customer needs and preferences, allowing banks and financial services providers to offer personalized products and services. The ability of predictive analytics to provide forward-looking insights empowers financial institutions to proactively address challenges and capitalize on opportunities, driving better business outcomes.
Applications of AI in Finance Management Services
Fraud Detection and Prevention
Fraud detection and prevention are critical components of financial security, and AI plays a pivotal role in enhancing these processes. Traditional methods of detecting fraud often rely on predefined rules and thresholds, which can be bypassed by sophisticated fraudsters. AI, particularly machine learning, offers a more robust solution by continuously learning and adapting to new patterns of fraudulent behavior. These systems analyze vast amounts of transaction data in real-time, identifying anomalies and suspicious activities that may indicate fraud. For example, AI can detect unusual spending patterns or login attempts from different geographic locations, triggering alerts for further investigation. Additionally, AI-powered systems can cross-reference transactions against known fraud databases and flag potential threats. By employing AI for fraud detection and prevention, financial institutions can significantly reduce the incidence of fraud, protect customer assets, and maintain trust in their services.
Customer Service Automation
Customer service automation is revolutionizing the way financial institutions interact with their clients. AI-driven technologies such as chatbots and virtual assistants provide instant, personalized responses to customer inquiries, enhancing the overall customer experience. These AI systems can handle a wide range of tasks, from answering frequently asked questions and processing transactions to providing financial advice and troubleshooting issues. By using natural language processing (NLP), these virtual assistants can understand and respond to customer queries in natural language, making interactions seamless and intuitive. Moreover, AI can analyze customer interactions to identify common issues and areas for improvement, enabling financial institutions to refine their service offerings continuously. Customer service automation not only improves efficiency and reduces operational costs but also ensures that customers receive timely and accurate assistance, thereby increasing satisfaction and loyalty.
Risk Management
Effective risk management is crucial for the stability and success of financial institutions, and AI significantly enhances this capability. AI tools can analyze vast datasets to identify potential risks and vulnerabilities that might be overlooked by human analysts. Machine learning algorithms, for example, can predict market volatility, assess credit risk, and detect signs of financial distress in real-time. By evaluating historical data and current market conditions, AI can provide early warnings of potential risks, allowing institutions to take proactive measures to mitigate them. Additionally, AI can help in stress testing and scenario analysis, enabling financial institutions to prepare for various adverse conditions and develop robust contingency plans. The integration of AI in risk management not only improves the accuracy and efficiency of risk assessments but also supports more informed and strategic decision-making, ensuring the long-term resilience of financial institutions.
Investment Strategies and Portfolio Management
AI is transforming investment strategies and portfolio management by providing advanced analytical tools and insights. Traditional investment approaches often rely on historical data and human intuition, which can be limited and subject to biases. AI, on the other hand, uses machine learning algorithms to analyze vast amounts of financial data, identify patterns, and make data-driven predictions. These algorithms can process information from diverse sources, including market trends, economic indicators, and social media sentiment, to develop more accurate investment models. AI can also continuously monitor and adjust portfolios in response to market changes, optimizing asset allocations to achieve better returns and minimize risks. Furthermore, AI-powered robo-advisors are becoming increasingly popular, offering personalized investment advice and portfolio management services to individual investors at a lower cost. By leveraging AI, investors and fund managers can enhance their decision-making processes, achieve superior performance, and stay ahead in the competitive financial markets.
AI in Personal Finance Management
Budgeting Tools
Budgeting tools powered by AI are transforming how individuals and businesses manage their finances. These tools leverage machine learning algorithms to analyze spending patterns, categorize expenses, and provide personalized financial insights. By integrating with bank accounts, credit cards, and other financial services, AI-driven budgeting tools offer real-time tracking of income and expenditures. They can automatically categorize transactions, identify areas where users might overspend, and suggest ways to save money. Additionally, these tools often feature predictive capabilities, allowing users to forecast future expenses based on historical data and upcoming bills. By providing a comprehensive and intuitive overview of one’s financial situation, AI-powered budgeting tools help users make informed decisions, set achievable financial goals, and maintain better control over their finances.
Personal Financial Advisors
AI-powered personal financial advisors, commonly known as robo-advisors, are revolutionizing the field of personal finance by offering accessible, affordable, and customized financial planning services. These digital platforms utilize algorithms to assess an individual’s financial situation, goals, and risk tolerance, providing personalized investment advice and portfolio management. Robo-advisors typically ask users a series of questions to understand their financial objectives and then create a tailored investment plan. They continuously monitor and adjust portfolios based on market conditions and individual preferences, ensuring optimal asset allocation and risk management. In addition to investment advice, AI-driven personal financial advisors can offer guidance on budgeting, saving, retirement planning, and more. By democratizing access to high-quality financial advice, these tools enable individuals of all income levels to achieve their financial goals and improve their overall financial health.
Credit Scoring and Lending
AI is significantly enhancing the credit scoring and lending processes, making them more accurate, inclusive, and efficient. Traditional credit scoring models rely heavily on historical credit data, which can exclude individuals with limited credit history or those with non-traditional income sources. AI-driven credit scoring systems, however, can analyze a broader range of data, including payment history, utility bills, rental payments, social media activity, and even behavioral patterns. This comprehensive approach enables lenders to assess creditworthiness more accurately and fairly. AI also automates the lending process, from application to approval, significantly reducing the time and effort required. Machine learning algorithms can swiftly evaluate applications, detect fraud, and ensure compliance with regulatory requirements. By leveraging AI, financial institutions can offer more personalized lending products, expand access to credit, and reduce default rates, ultimately creating a more inclusive and efficient lending ecosystem.
AI in Corporate Finance
Financial Planning and Analysis
Financial planning and analysis (FP&A) are critical functions in any organization, and AI is transforming these processes by providing deeper insights and more accurate forecasts. AI-driven tools can analyze historical financial data, market trends, and economic indicators to create detailed financial models and projections. These models help businesses identify growth opportunities, optimize resource allocation, and prepare for potential risks. By automating routine tasks such as data collection and reporting, AI frees up FP&A professionals to focus on strategic decision-making. Moreover, AI can provide real-time analysis and scenario planning, enabling companies to quickly adapt to changing market conditions and make informed decisions. The enhanced precision and efficiency offered by AI in financial planning and analysis lead to better financial performance and a more agile business strategy.
Expense Management
AI-powered expense management solutions streamline the tracking, categorization, and approval of business expenses. These systems can automatically capture expense data from receipts, invoices, and financial transactions, categorizing them accurately without manual intervention. Machine learning algorithms analyze spending patterns to identify anomalies and potential areas for cost savings. For instance, AI can flag duplicate expenses, detect policy violations, and ensure compliance with corporate spending policies. Additionally, AI-driven expense management tools often integrate with other financial systems, providing a seamless workflow for employees and finance teams. By automating expense reporting and analysis, businesses can reduce administrative overhead, enhance accuracy, and gain better visibility into their spending, ultimately leading to more effective cost control and financial planning.
Cash Flow Forecasting
Cash flow forecasting is essential for maintaining the financial health of a business, and AI significantly enhances the accuracy and reliability of these forecasts. Traditional cash flow forecasting methods often rely on static historical data and manual adjustments, which can be time-consuming and prone to errors. AI-driven forecasting tools, however, use machine learning algorithms to analyze a wide range of data inputs, including historical cash flows, market conditions, sales forecasts, and payment cycles. These tools can identify patterns and trends that humans might miss, providing more precise and dynamic cash flow predictions. Additionally, AI can simulate various scenarios to help businesses prepare for different financial situations, such as economic downturns or rapid growth. By leveraging AI for cash flow forecasting, companies can ensure they have sufficient liquidity to meet their obligations, make informed investment decisions, and optimize their financial strategies.
Regulatory Compliance
Regulatory compliance is a major concern for financial institutions, and AI plays a vital role in ensuring adherence to complex and evolving regulations. AI-powered compliance tools can automate the monitoring and reporting of financial activities, reducing the risk of human error and ensuring accuracy. These tools use machine learning algorithms to analyze transactions and identify suspicious activities, such as money laundering or fraud, in real-time. Natural language processing (NLP) can be used to scan and interpret regulatory texts, helping institutions stay up-to-date with new laws and guidelines. Additionally, AI can streamline the process of generating compliance reports and maintaining audit trails, making it easier for organizations to demonstrate adherence to regulatory requirements. By integrating AI into their compliance strategies, financial institutions can reduce the costs and complexities associated with regulatory compliance, mitigate risks, and maintain their reputations.
AI in Banking
Customer Relationship Management
Customer Relationship Management (CRM) is vital for financial institutions aiming to maintain and grow their client base. AI enhances CRM systems by providing deeper insights into customer behaviors and preferences through data analysis. AI-driven CRM tools can analyze interactions across various channels, such as email, social media, and phone calls, to build comprehensive customer profiles. This enables financial institutions to offer personalized services and targeted marketing campaigns. Predictive analytics can forecast customer needs and behaviors, allowing proactive engagement and retention strategies. Additionally, AI-powered chatbots and virtual assistants can handle routine inquiries and provide 24/7 support, improving customer satisfaction and freeing up human agents for more complex tasks. By leveraging AI, financial institutions can foster stronger relationships with their clients, enhance customer loyalty, and drive business growth.
Loan and Credit Assessment
AI is revolutionizing loan and credit assessment by making the process faster, more accurate, and more inclusive. Traditional credit assessment methods rely heavily on credit scores and financial history, which can exclude individuals with limited credit history or unconventional income sources. AI-driven systems can analyze a broader set of data, including social media activity, utility payments, and transaction history, to evaluate creditworthiness more comprehensively. Machine learning algorithms assess risk by identifying patterns and correlations that might be overlooked by conventional methods. This allows lenders to make more informed decisions and offer credit to a wider range of applicants. Additionally, AI can automate the loan application process, from initial screening to final approval, significantly reducing processing time and costs. By using AI for loan and credit assessment, financial institutions can enhance accuracy, reduce default rates, and provide better access to credit.
Automated Trading
Automated trading, also known as algorithmic trading, leverages AI to execute trades at optimal times based on predefined criteria and real-time market data. AI algorithms analyze vast amounts of financial data, including historical prices, market trends, and economic indicators, to identify trading opportunities and make split-second decisions. These systems can operate at speeds and efficiencies unattainable by human traders, executing trades at the most advantageous moments to maximize returns. Machine learning models continuously refine their strategies based on new data, adapting to changing market conditions and improving performance over time. Automated trading reduces the emotional bias and human error associated with manual trading, leading to more consistent and profitable outcomes. Financial institutions and individual investors alike benefit from AI-driven automated trading by achieving better market performance and managing risks more effectively.
Anti-Money Laundering (AML)
Anti-Money Laundering (AML) measures are crucial for financial institutions to prevent illegal activities such as money laundering and terrorism financing. AI enhances AML efforts by automating the detection and reporting of suspicious activities. Traditional AML processes often involve manual monitoring and analysis, which can be time-consuming and prone to human error. AI-powered systems use machine learning algorithms to analyze vast amounts of transaction data in real-time, identifying patterns and anomalies that may indicate money laundering. These systems can adapt to new laundering techniques by learning from past data, improving their accuracy and effectiveness. Natural language processing (NLP) helps in analyzing unstructured data, such as emails and transaction descriptions, to uncover hidden risks. By integrating AI into their AML strategies, financial institutions can enhance compliance with regulatory requirements, reduce the risk of financial crimes, and maintain the integrity of the financial system.
AI in Insurance
Underwriting and Pricing
AI is significantly transforming underwriting and pricing processes in the insurance and finance industries. Traditional underwriting relies heavily on historical data and manual analysis, which can be time-consuming and prone to errors. AI, however, brings efficiency and accuracy to these processes by utilizing machine learning algorithms to analyze vast amounts of data, including customer information, market trends, and external data sources. This enables more precise risk assessments and pricing strategies. AI can identify patterns and correlations that humans might miss, leading to more accurate predictions of risk and more personalized pricing. For example, in insurance, AI can assess the risk of insuring a particular individual or asset by considering a wide range of factors, such as lifestyle, health, and environmental conditions. By optimizing underwriting and pricing, AI helps financial institutions improve profitability, reduce risks, and offer more competitive and tailored products to their customers.
Claims Processing
AI is revolutionizing claims processing by automating and streamlining the entire workflow. In the insurance industry, claims processing has traditionally been a labor-intensive process involving manual data entry, verification, and assessment. AI-powered systems can automate these tasks, significantly reducing processing time and improving accuracy. For example, machine learning algorithms can analyze claim submissions, verify the authenticity of the information, and detect potential fraud. Natural language processing (NLP) can be used to extract relevant information from unstructured data, such as emails and documents, further speeding up the process. Additionally, AI can prioritize claims based on complexity and urgency, ensuring that high-priority cases are addressed promptly. By enhancing the efficiency and accuracy of claims processing, AI helps insurance companies reduce operational costs, improve customer satisfaction, and expedite the resolution of claims.
Fraud Detection
Fraud detection is a critical area where AI has a profound impact, especially in the finance and insurance sectors. Traditional fraud detection methods often rely on rule-based systems that can be bypassed by sophisticated fraudsters. AI, particularly machine learning, offers a more dynamic and robust solution. These systems continuously analyze transaction data, customer behavior, and historical fraud patterns to identify anomalies and suspicious activities in real-time. AI can detect subtle indicators of fraud that are not apparent through manual analysis, such as unusual spending patterns or discrepancies in user behavior. Furthermore, AI systems can adapt to new fraud tactics by learning from emerging data, enhancing their effectiveness over time. By employing AI for fraud detection, financial institutions and insurance companies can significantly reduce the incidence of fraud, protect customer assets, and maintain trust and security in their services.
Customer Experience
AI is enhancing customer experience across the financial and insurance industries by providing personalized, efficient, and responsive services. AI-driven technologies such as chatbots and virtual assistants offer 24/7 support, handling routine inquiries and transactions quickly and accurately. These systems use natural language processing (NLP) to understand and respond to customer queries in a conversational manner, making interactions seamless and user-friendly. AI can also analyze customer data to provide personalized recommendations and offers, improving customer satisfaction and loyalty. For instance, AI can suggest financial products or insurance policies tailored to an individual’s needs and preferences based on their behavior and financial history. Additionally, AI-powered analytics can track customer interactions across various channels, helping businesses understand customer needs and pain points better. By leveraging AI to enhance customer experience, financial institutions and insurance companies can build stronger relationships, increase customer retention, and drive business growth.
Ethical and Regulatory Considerations
Data Privacy and Security
Data privacy and security are paramount concerns in the era of AI-driven technologies. As AI systems increasingly rely on vast amounts of personal and sensitive data, ensuring the protection and privacy of this data is crucial. Financial institutions and organizations must implement robust data encryption methods, access controls, and secure storage solutions to safeguard against unauthorized access and data breaches. Additionally, AI algorithms should be designed with privacy-preserving techniques, such as federated learning or differential privacy, to minimize the risk of exposing sensitive information during data processing. It is essential to adhere to data protection regulations and frameworks, such as GDPR in Europe or CCPA in California, which mandate strict guidelines on data collection, storage, and usage. By prioritizing data privacy and security, organizations can build trust with their customers, mitigate risks, and comply with legal requirements, thereby ensuring the responsible deployment of AI technologies.
Bias and Fairness in AI
Bias and fairness in AI algorithms are critical considerations, particularly in financial and insurance sectors where decisions can have significant impacts on individuals’ lives. AI systems learn from historical data, which may contain biases reflecting societal inequalities and historical prejudices. These biases can lead to discriminatory outcomes in areas such as credit scoring, loan approvals, and insurance premiums. Addressing bias requires careful data collection, preprocessing, and algorithm design to mitigate biases and ensure fairness. Techniques such as algorithmic auditing, bias detection, and fairness-aware learning can help identify and mitigate biases in AI models. Moreover, diverse and inclusive datasets, as well as multidisciplinary teams, can contribute to developing fairer AI systems. It is essential for organizations to prioritize fairness and transparency in their AI development processes to build equitable solutions that benefit all stakeholders.
Regulatory Frameworks
AI technologies in finance and insurance are subject to stringent regulatory frameworks aimed at ensuring ethical use, consumer protection, and data privacy. Regulatory bodies worldwide are developing guidelines and standards to govern the deployment and operation of AI systems. These frameworks address various aspects such as data protection, algorithmic transparency, accountability, and ethical considerations. Financial institutions and insurance companies must comply with regulations specific to their regions, such as GDPR in Europe, the CCPA in California, and sector-specific regulations like Basel III in banking. Compliance involves implementing measures to assess and mitigate risks associated with AI, ensuring transparency in decision-making processes, and establishing mechanisms for accountability and oversight. By adhering to regulatory frameworks, organizations can mitigate legal risks, enhance trust with stakeholders, and foster responsible AI innovation.
Transparency and Explainability
Transparency and explainability are crucial for building trust and ensuring accountability in AI-driven decisions, particularly in finance and insurance. AI systems often operate as black boxes, making it challenging to understand how decisions are made or to verify the reasoning behind them. Transparency involves making AI processes and decisions understandable to stakeholders, including customers, regulators, and internal teams. Explainability refers to the ability to provide clear explanations of AI decisions in a human-understandable manner. Techniques such as model interpretability, feature importance analysis, and algorithmic transparency can enhance transparency and explainability in AI systems. Financial institutions and insurance companies must prioritize these aspects to ensure that AI-driven decisions are fair, unbiased, and aligned with ethical standards. By promoting transparency and explainability, organizations can foster trust, facilitate regulatory compliance, and promote responsible AI adoption in the financial and insurance sectors.
Challenges and Limitations of AI in Finance
Data Quality and Availability
Data quality and availability are fundamental challenges in leveraging AI within the financial and insurance sectors. Effective AI applications depend on access to large volumes of high-quality data, which may include structured financial records, transaction histories, customer profiles, and market data. Ensuring data accuracy, completeness, and consistency is crucial for training reliable AI models and making informed decisions. However, financial institutions often encounter issues such as data silos, inconsistent formats, and incomplete datasets, which can hinder AI initiatives. Addressing these challenges requires implementing data governance frameworks, data cleansing processes, and robust data integration strategies. Additionally, organizations may need to collaborate with data providers and invest in technologies that improve data capture and storage capabilities. By enhancing data quality and availability, financial and insurance companies can unlock the full potential of AI, driving innovation, and achieving competitive advantages.
Integration with Legacy Systems
Integrating AI technologies with existing legacy systems poses significant challenges for financial and insurance organizations. Legacy systems, which may be outdated, monolithic, and proprietary, were not designed to support modern AI applications. This can lead to compatibility issues, data integration complexities, and increased implementation costs. Organizations must undertake careful planning and assessment to determine how AI solutions can be seamlessly integrated with legacy systems without disrupting core operations. This may involve adopting middleware solutions, APIs, and data interoperability standards to facilitate communication between AI platforms and legacy systems. Additionally, organizations may consider phased migration strategies or hybrid approaches to gradually modernize their infrastructure while leveraging AI capabilities. Overcoming these integration challenges is essential for maximizing the benefits of AI, improving operational efficiency, and delivering superior customer experiences.
High Costs of Implementation
The implementation of AI technologies in finance and insurance often entails substantial costs, including investments in technology infrastructure, software development, data management, and talent acquisition. AI projects require significant upfront investments in hardware, software licenses, and cloud computing services to support data processing and AI model training. Moreover, organizations must allocate resources for hiring skilled AI professionals, data scientists, and engineers capable of developing and maintaining AI solutions. The costs of implementation are further compounded by the need for rigorous testing, regulatory compliance, and ongoing support and maintenance. Financial institutions and insurance companies must carefully assess the return on investment (ROI) and long-term benefits of AI adoption to justify these upfront costs. Cost-effective strategies may include partnering with AI vendors, leveraging open-source AI frameworks, and adopting scalable cloud-based solutions to manage expenses while driving innovation and operational efficiency.
Skills and Talent Gap
The shortage of skilled AI professionals represents a significant challenge for financial and insurance sectors looking to implement AI initiatives effectively. AI expertise encompasses a range of specialized skills, including machine learning, data science, natural language processing (NLP), and algorithm development. However, the demand for these skills often outpaces the supply, resulting in a talent gap that impedes AI adoption and innovation. Financial institutions and insurance companies must invest in talent development programs, partnerships with academic institutions, and recruitment strategies to attract and retain AI talent. Upskilling existing employees through training programs and certifications can also help bridge the skills gap and build a capable AI workforce internally. Additionally, organizations may explore collaborative approaches, such as outsourcing AI projects to specialized firms or forming partnerships with technology providers, to access external expertise and accelerate AI initiatives. By addressing the skills and talent gap, financial and insurance sectors can build sustainable AI capabilities, drive digital transformation, and remain competitive in a rapidly evolving landscape.
The Future of AI in Finance
Emerging Technologies
Emerging technologies continue to reshape the landscape of finance and insurance, offering new opportunities for innovation and efficiency. Key technologies include blockchain, quantum computing, Internet of Things (IoT), and advanced AI applications. Blockchain technology, for example, enables secure and transparent transactions through decentralized ledgers, potentially revolutionizing payment systems and reducing fraud. Quantum computing holds promise for solving complex mathematical problems and enhancing data encryption, which could transform risk analysis and cybersecurity in finance. IoT devices provide real-time data from physical assets, enabling insurers to offer usage-based insurance and personalized risk assessments. These technologies are poised to drive significant advancements, offering new business models, enhancing operational efficiencies, and improving customer experiences in the financial and insurance sectors.
Potential Impact on Financial Jobs
The adoption of AI and automation technologies in finance raises concerns about the potential impact on employment. While AI can streamline operations, automate routine tasks, and improve decision-making processes, it may also lead to job displacement in certain roles. For instance, AI-powered chatbots and virtual assistants can handle customer inquiries, reducing the need for human customer service representatives. Similarly, automated trading algorithms can execute transactions with minimal human intervention, impacting roles in trading and asset management. However, AI also creates new opportunities for jobs that require skills in data analysis, AI development, cybersecurity, and regulatory compliance. The future workforce in finance is likely to evolve with a blend of human expertise and AI capabilities, necessitating continuous reskilling and upskilling to remain competitive in a digital economy.
Collaboration Between Humans and AI
Successful integration of AI in finance and insurance hinges on effective collaboration between humans and AI systems. AI excels at processing vast amounts of data, identifying patterns, and making data-driven predictions. Human expertise, on the other hand, provides contextual understanding, ethical judgment, and creativity that AI currently lacks. Collaboration between humans and AI enables complementary strengths: AI enhances efficiency by automating repetitive tasks and providing analytical insights, while humans contribute domain expertise, critical thinking, and interpersonal skills. In customer service, for example, AI-powered chatbots can handle routine inquiries, freeing human agents to focus on complex issues and building stronger client relationships. This symbiotic relationship fosters innovation, improves decision-making processes, and enhances the overall effectiveness of financial and insurance operations.
Predictions for the Next Decade
Looking ahead, the next decade is expected to witness profound transformations in the financial and insurance sectors driven by AI and digital technologies. AI adoption will continue to expand, leading to smarter, more personalized financial services and insurance products. Advances in machine learning and predictive analytics will enable better risk management, fraud detection, and customer segmentation. Moreover, technologies like blockchain and IoT will foster greater transparency, efficiency, and security in financial transactions and insurance claims processing. The workforce will undergo significant changes, with a growing emphasis on AI literacy, data science skills, and interdisciplinary knowledge. Regulatory frameworks will evolve to address ethical concerns, data privacy issues, and the equitable deployment of AI technologies. Overall, the next decade promises to be transformative, characterized by innovation, adaptation to new technologies, and the reshaping of business models in finance and insurance.
Conclusion
In conclusion, AI represents a transformative force with immense potential to revolutionize the finance industry. From enhancing customer experiences and optimizing operational efficiencies to improving risk management and regulatory compliance, AI is reshaping traditional practices and paving the way for innovation. As financial institutions and insurance companies embrace AI technologies, they must navigate challenges such as data privacy, integration with legacy systems, and skills gaps while leveraging emerging technologies like blockchain and IoT. Despite these challenges, AI promises to unlock new opportunities for growth, resilience, and competitive advantage. The future landscape of finance will be characterized by collaborative efforts between humans and AI systems, driving continuous evolution and adaptation in response to technological advancements and changing consumer expectations. By embracing AI responsibly and ethically, the finance sector can harness its transformative potential to create value, mitigate risks, and deliver superior outcomes for businesses and customers alike.
FAQs
What is AI’s role in financial management?
AI plays a pivotal role in financial management by automating and optimizing various processes, such as risk assessment, investment management, and customer service. AI algorithms analyze vast amounts of data to identify patterns, trends, and insights that human analysts may overlook. This enables more accurate financial forecasting, personalized recommendations, and efficient decision-making, ultimately enhancing overall operational efficiency and profitability in financial institutions.
How does AI improve fraud detection?
AI enhances fraud detection by leveraging machine learning algorithms to analyze large datasets and detect patterns indicative of fraudulent activities. AI systems can identify anomalies in transaction behavior, flag suspicious activities in real-time, and adapt to new fraud patterns through continuous learning. This proactive approach not only reduces false positives but also enhances the accuracy and speed of fraud detection, thereby minimizing financial losses and protecting customer assets.
Can AI provide personalized financial advice?
Yes, AI can provide personalized financial advice by analyzing individual financial data, goals, and risk profiles. AI-powered financial advisory platforms, known as robo-advisors, use algorithms to assess a client’s financial situation and preferences, recommend suitable investment options, and optimize portfolio allocations. These systems continuously monitor market conditions and adjust recommendations accordingly, providing clients with tailored financial guidance and improving overall investment outcomes.
What are the ethical considerations of using AI in finance?
Ethical considerations in AI adoption in finance include concerns about data privacy, algorithmic bias, transparency, and accountability. AI systems rely on vast amounts of personal and sensitive data, raising questions about consent, data protection, and user privacy. Algorithmic bias, where AI models reflect societal biases present in training data, can lead to discriminatory outcomes in credit scoring, lending decisions, and insurance premiums. Ensuring transparency in AI decision-making processes and establishing mechanisms for accountability are essential to mitigate these risks and uphold ethical standards in the use of AI in finance.
What are the challenges in implementing AI in financial institutions?
Implementing AI in financial institutions faces several challenges, including data quality and integration, legacy system compatibility, high implementation costs, and the skills gap. Financial data often resides in disparate systems with varying formats and quality, making data aggregation and preprocessing complex. Legacy systems, designed before the AI era, may lack interoperability with modern AI technologies, necessitating costly upgrades or middleware solutions. Moreover, recruiting and retaining skilled AI talent poses a challenge, as demand outpaces supply in the rapidly evolving field of AI. Overcoming these challenges requires strategic planning, investment in technology infrastructure, and fostering a culture of AI literacy and innovation within financial institutions.