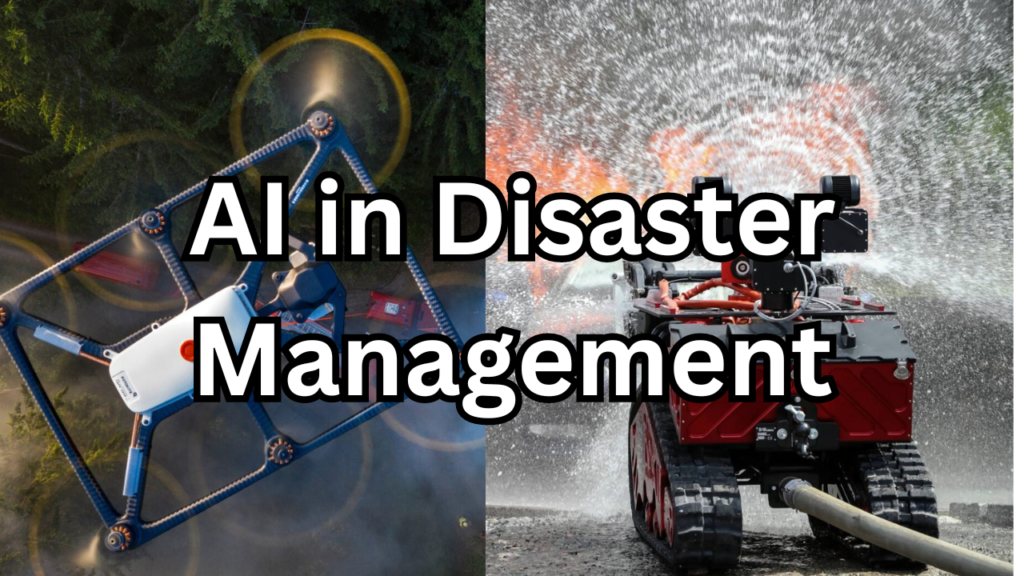
Table of Contents
Introduction:
Disasters, whether natural or man-made, pose significant challenges to societies worldwide, threatening lives, infrastructure, and economies. Effective disaster management involves a structured approach encompassing prevention, preparedness, response, and recovery phases. As the frequency and intensity of disasters increase globally, there is a pressing need for innovative solutions to enhance disaster resilience and response capabilities. This article explores the transformative role of Artificial Intelligence (AI) in revolutionizing disaster management, examining how AI technologies are reshaping traditional practices and empowering stakeholders to mitigate risks and respond more effectively to crises.
Overview of Disaster Management:
Disaster management refers to the organized efforts and procedures aimed at mitigating the impact of disasters on communities and restoring normalcy post-event. It encompasses a comprehensive framework that includes risk assessment, planning, coordination of resources, communication, and continuous monitoring. The primary phases of disaster management include prevention (reducing risk factors and vulnerabilities), preparedness (training, planning, and readiness), response (immediate actions to save lives and reduce suffering), and recovery (restoring infrastructure and supporting affected communities). Effective disaster management requires collaboration across sectors, proactive measures, and adaptive strategies to address evolving threats.
The Growing Importance of AI in Disaster Response:
In recent years, AI has emerged as a game-changer in disaster response, offering unprecedented capabilities to analyze vast amounts of data quickly and accurately. AI technologies such as machine learning, natural language processing, computer vision, and robotics enable real-time decision-making, predictive analytics, and automation of critical tasks during emergencies. These capabilities significantly enhance situational awareness, optimize resource allocation, improve coordination among response teams, and accelerate the delivery of aid to affected areas. As disasters become more complex and frequent, the integration of AI in disaster response operations has become increasingly vital for enhancing efficiency, saving lives, and minimizing economic losses.
Purpose and Scope of the Article:
This article aims to provide a comprehensive exploration of how AI is transforming disaster management practices globally. It seeks to delve into various AI technologies currently employed in disaster prevention, preparedness, response, and recovery phases, showcasing their applications through real-world examples and case studies. Additionally, the article will discuss the ethical and practical considerations associated with the use of AI in disaster management, future trends, and challenges, aiming to inform stakeholders, policymakers, and the general public about the potential and limitations of AI in enhancing disaster resilience and response capabilities.
Understanding Disaster Management
Definition and Scope of Disaster Management:
Disaster management refers to the systematic approach of managing and minimizing the impact of disasters on society. It encompasses a range of activities aimed at reducing the vulnerabilities of communities and enhancing their ability to cope with and recover from disasters. The scope of disaster management includes risk assessment, planning, preparedness, response, and recovery efforts. It involves coordination among various stakeholders including government agencies, non-governmental organizations (NGOs), emergency responders, and the public. The ultimate goal of disaster management is to save lives, protect property, and promote sustainable recovery in the aftermath of disasters, whether they are natural, technological, or human-induced.
Phases of Disaster Management:
Disaster management is typically organized into four key phases: prevention, preparedness, response, and recovery. Prevention involves activities aimed at reducing or eliminating the risk factors that can lead to disasters. This includes measures such as land-use planning, building codes, environmental conservation, and public education. Preparedness focuses on enhancing the readiness of individuals, communities, and organizations to respond effectively to disasters. It involves training, exercises, early warning systems, stockpiling of resources, and developing emergency plans.
Historical Perspective on Disaster Management:
The concept of disaster management has evolved significantly over time in response to various historical events and lessons learned. Early approaches were often reactive, focusing primarily on immediate response and relief efforts. However, with the increasing frequency and impact of disasters, particularly in the 20th century, there was a shift towards more proactive and systematic approaches. Major disasters such as earthquakes, floods, hurricanes, and pandemics have shaped the evolution of disaster management policies and practices globally.
AI Technologies in Disaster Management
Machine Learning:
Machine learning is a branch of artificial intelligence (AI) focused on developing algorithms that allow computers to learn from and make predictions or decisions based on data. It involves training models using large datasets, where algorithms learn patterns and relationships, enabling them to make decisions or predictions without being explicitly programmed. Machine learning algorithms are used in various applications, including predictive analytics, pattern recognition, fraud detection, recommendation systems, and more recently, in disaster management for predicting and managing crisis situations based on historical data and real-time information.
Natural Language Processing (NLP):
Natural Language Processing is a subfield of AI that focuses on enabling computers to understand, interpret, and generate human language. NLP algorithms process and analyze large amounts of natural language data, enabling tasks such as language translation, sentiment analysis, chatbot interactions, and text summarization. In disaster management, NLP can be used to analyze social media feeds, news articles, emergency calls, and other textual data sources to extract critical information, identify trends, and understand public sentiment during crises.
Computer Vision:
Computer vision is an interdisciplinary field that enables computers to interpret and understand visual information from the world. It involves developing algorithms and techniques for acquiring, processing, analyzing, and understanding digital images and videos. Applications of computer vision include object recognition, facial recognition, image segmentation, autonomous vehicles, and medical image analysis. In disaster management, computer vision technologies are used for tasks such as damage assessment through satellite imagery, monitoring evacuation routes, identifying hazards, and facilitating search and rescue operations in hazardous environments.
Robotics:
Robotics is the branch of technology that deals with the design, construction, operation, and use of robots. Robots are programmable machines capable of carrying out tasks autonomously or semi-autonomously. In disaster management, robots are utilized for various purposes such as search and rescue missions in collapsed buildings or hazardous environments, delivering medical supplies in inaccessible areas, and performing repetitive tasks that would be dangerous for humans. Robotics combined with AI technologies like machine learning and computer vision enhance the capabilities of robots to operate effectively in dynamic and unpredictable disaster scenarios.
Geographic Information Systems (GIS):
Geographic Information Systems (GIS) are computer systems designed to capture, store, manipulate, analyze, manage, and present spatial or geographical data. GIS integrates hardware, software, and data for capturing, managing, analyzing, and displaying all forms of geographically referenced information. In disaster management, GIS plays a crucial role in mapping and visualizing disaster-prone areas, assessing risk factors, planning evacuation routes, coordinating emergency response efforts, and analyzing spatial relationships between various disaster-related factors. GIS technology enables decision-makers to make informed decisions based on spatial data, improving overall disaster preparedness and response effectiveness.
AI in Disaster Prevention
Predictive Analytics for Disaster Risk Assessment:
Predictive analytics involves the use of statistical algorithms and machine learning techniques to analyze historical data and predict future events or outcomes. In the context of disaster management, predictive analytics is used to assess and forecast the likelihood and impact of potential disasters. By analyzing historical data such as weather patterns, seismic activity, population density, infrastructure vulnerability, and socio-economic factors, predictive analytics models can identify areas at higher risk of disasters. This information helps governments, emergency responders, and communities to prioritize resources, implement preventive measures, and develop response plans to mitigate the impact of disasters.
Early Warning Systems:
Early warning systems (EWS) are crucial components of disaster preparedness and response strategies. These systems use data from various sources such as meteorological sensors, seismic detectors, satellite imagery, and social media feeds to detect and forecast imminent threats such as hurricanes, earthquakes, tsunamis, floods, and wildfires. Advanced AI algorithms process and analyze real-time data to issue timely alerts and warnings to authorities and the public, allowing them to take preventive actions, evacuate vulnerable areas, and prepare emergency responses. Early warning systems save lives by providing critical time for people to seek safety and mitigate potential damage from disasters.
Environmental Monitoring and Hazard Detection:
Environmental monitoring and hazard detection systems utilize AI technologies such as remote sensing, IoT sensors, and data analytics to continuously monitor natural environments for early signs of potential hazards. These systems track parameters such as air quality, water levels, temperature fluctuations, seismic activity, and vegetation health. AI algorithms analyze the collected data in real-time to detect anomalies or patterns indicative of impending disasters such as wildfires, landslides, volcanic eruptions, or toxic spills. By providing early detection and real-time monitoring, these AI-powered systems enable prompt response and mitigation measures, reducing the impact on ecosystems and human populations.
Case Studies: AI in Preventing Natural Disasters:
AI technologies have been increasingly applied to prevent and mitigate the impact of natural disasters through innovative approaches and case studies. For instance, AI-driven predictive models have been used to forecast hurricanes and typhoons more accurately, allowing for early evacuation and preparedness measures. In earthquake-prone regions, AI algorithms analyze seismic data to predict tremors and enhance building resilience. AI-powered flood prediction models integrate weather data with historical flood patterns to issue timely warnings and plan evacuation routes. Additionally, AI-driven wildfire detection systems use satellite imagery and machine learning to identify fire outbreaks early, enabling rapid response and containment efforts. These case studies demonstrate the transformative potential of AI in improving disaster preparedness, response efficiency, and community resilience against natural disasters.
AI in Disaster Preparedness
Simulation and Modeling for Disaster Scenarios:
Simulation and modeling are essential tools in disaster management for predicting, analyzing, and preparing for potential disaster scenarios. AI-driven simulations use complex algorithms to recreate realistic disaster scenarios based on various factors such as geographical data, population density, infrastructure layout, and environmental conditions. These simulations help emergency planners and responders to understand how disasters might unfold, assess the impact on communities, and test different response strategies. By running simulations, stakeholders can refine emergency plans, optimize resource allocation, and improve coordination among response teams before a disaster occurs. This proactive approach enhances preparedness and resilience, allowing for more effective response and recovery efforts when disasters strike.
Resource Allocation and Logistics Planning:
Effective resource allocation and logistics planning are critical components of disaster response operations, ensuring that essential supplies, personnel, and equipment are deployed efficiently to affected areas. AI technologies play a vital role in optimizing resource allocation by analyzing real-time data, such as population movements, damage assessments, and resource availability. Machine learning algorithms can predict demand for medical supplies, food, water, and shelter based on disaster severity and demographics. AI-powered logistics systems use predictive analytics to identify the most efficient transportation routes, coordinate supply chains, and prioritize delivery schedules. By leveraging AI for resource allocation and logistics planning, emergency responders can enhance their ability to meet the immediate needs of affected populations and streamline recovery efforts in the aftermath of disasters.
Public Education and Awareness through AI Tools:
AI tools are increasingly being used to enhance public education and awareness campaigns aimed at preparing communities for disasters. Natural language processing (NLP) algorithms analyze public sentiment, social media trends, and news articles to gauge public perception and understanding of disaster risks. AI-powered chatbots and virtual assistants provide accessible information on disaster preparedness, evacuation procedures, and emergency contacts to the public. Machine learning algorithms personalize communication strategies based on demographic data, ensuring targeted outreach to vulnerable populations. Additionally, AI-driven simulations and interactive platforms simulate disaster scenarios, allowing individuals to practice emergency responses and make informed decisions during crises. By leveraging AI tools for public education and awareness, governments and organizations can empower communities to take proactive measures, enhance resilience, and reduce the impact of disasters.
Training Emergency Responders with AI Simulations:
AI simulations are transforming the training and preparedness of emergency responders by providing realistic and interactive learning experiences. Virtual reality (VR) and augmented reality (AR) simulations simulate disaster scenarios, allowing responders to practice decision-making, communication, and coordination in a safe and controlled environment. Machine learning algorithms generate dynamic scenarios that adapt to responder actions, offering personalized training experiences based on individual performance and skill levels. AI-powered simulations replicate diverse disaster situations, from natural disasters like earthquakes and floods to human-made emergencies such as terrorist attacks or industrial accidents. By integrating AI simulations into training programs, emergency responders can improve their response capabilities, teamwork, and readiness to effectively manage complex and unpredictable disaster scenarios in real-world settings.
AI in Disaster Response
Real-time Data Analysis and Decision Support:
Real-time data analysis and decision support systems powered by AI are revolutionizing disaster response efforts by providing timely and actionable insights to emergency responders and decision-makers. AI algorithms process vast amounts of data from various sources including sensors, satellites, social media, and IoT devices. By analyzing this data in real-time, these systems can detect patterns, trends, and anomalies that signify emerging threats or changing conditions during disasters such as earthquakes, hurricanes, or pandemics. This capability allows authorities to make informed decisions quickly, such as deploying resources to areas most in need, adjusting evacuation routes based on changing conditions, or prioritizing medical assistance to critically affected individuals. Real-time data analysis and decision support systems enhance situational awareness, improve response coordination, and ultimately save lives by enabling faster and more effective responses to evolving disaster scenarios.
Drones and Robots in Search and Rescue Operations:
Drones and robots equipped with AI technologies are increasingly deployed in search and rescue operations to enhance response capabilities and access hard-to-reach or hazardous areas during disasters. Drones equipped with cameras, thermal sensors, and AI-powered image recognition can quickly survey disaster-stricken areas, identify survivors or victims, and assess structural damage to buildings. AI algorithms analyze data collected by drones to generate real-time maps, pinpointing locations of survivors or hazards. Similarly, robots equipped with AI capabilities can navigate through debris, deliver medical supplies, or perform remote inspections in unsafe environments where human access is limited. By leveraging drones and robots in search and rescue operations, emergency responders can expedite response times, reduce risks to personnel, and increase the likelihood of locating and rescuing individuals trapped in disaster zones.
AI-driven Communication Systems for Coordinating Efforts:
AI-driven communication systems play a crucial role in coordinating and managing response efforts across multiple agencies, organizations, and stakeholders during disasters. These systems use AI algorithms to process and prioritize incoming data, messages, and requests for assistance from various sources such as emergency calls, social media posts, and field reports. Natural language processing (NLP) enables these systems to extract actionable information, identify critical needs, and route messages to appropriate responders or decision-makers in real-time. AI-powered chatbots and virtual assistants provide automated responses to common inquiries, freeing up human operators to focus on more complex tasks. By improving the efficiency and accuracy of communication, AI-driven systems enhance coordination among response teams, facilitate resource allocation, and ensure timely delivery of aid and services to affected communities during emergencies.
Examples of AI in Recent Disaster Responses:
In recent years, AI technologies have been increasingly utilized in disaster responses worldwide, demonstrating their transformative impact on enhancing resilience and recovery efforts. For instance, during the 2020 COVID-19 pandemic, AI-powered predictive models were used to forecast disease spread, allocate medical resources, and guide public health interventions. In natural disasters such as hurricanes and wildfires, AI algorithms analyzed satellite imagery and weather data to predict disaster paths and intensity, enabling authorities to issue timely warnings and evacuate at-risk populations. AI-driven robotics and drones have been deployed in earthquake-stricken areas to conduct rapid damage assessments and search for survivors in collapsed buildings. Moreover, AI-driven simulation models have been employed to simulate disaster scenarios, train emergency responders, and test disaster response plans before actual events occur. These examples highlight the diverse applications of AI in improving disaster preparedness, response efficiency, and community resilience in the face of complex and evolving disaster challenges.
AI in Disaster Recovery
Damage Assessment Using AI and Drones:
AI and drones are transforming the process of damage assessment following disasters by enabling rapid and accurate evaluation of affected areas. Drones equipped with high-resolution cameras, LiDAR (Light Detection and Ranging) sensors, and AI-powered image processing capabilities can capture detailed aerial imagery of disaster-stricken regions. AI algorithms analyze this data to assess the extent of damage to infrastructure, buildings, roads, and utilities. Machine learning models can classify damage severity, identify areas requiring urgent repairs or reconstruction, and prioritize resources accordingly. By automating and accelerating the damage assessment process, AI and drones facilitate quicker response planning, resource allocation, and decision-making for recovery efforts, ultimately speeding up the recovery process and reducing overall recovery costs.
Optimizing Relief Distribution with AI:
AI technologies play a vital role in optimizing the distribution of relief supplies and resources to affected populations during and after disasters. AI-driven algorithms analyze real-time data such as population demographics, damage assessments, transportation routes, and logistics constraints to optimize supply chain operations. Machine learning models predict demand for essential goods and services based on evolving needs and conditions on the ground. AI-powered logistics systems dynamically adjust distribution routes, prioritize deliveries to areas with the greatest need, and minimize delays or wastage. By improving the efficiency and effectiveness of relief distribution, AI helps ensure that life-saving aid reaches affected communities promptly, enhances coordination among relief organizations, and maximizes the impact of humanitarian assistance in disaster-affected areas.
Monitoring and Supporting Recovery Progress:
AI technologies enable continuous monitoring and evaluation of recovery progress in post-disaster environments, facilitating data-driven decision-making and adaptive planning. AI-driven analytics platforms integrate diverse data sources, including satellite imagery, sensor data, socioeconomic indicators, and progress reports from recovery projects. Machine learning algorithms analyze this data to track key metrics such as infrastructure reconstruction, economic recovery indicators, social welfare improvements, and environmental rehabilitation efforts. AI-powered dashboards and visualization tools provide stakeholders with real-time insights into recovery trends, bottlenecks, and areas needing additional support. By monitoring recovery progress, identifying challenges early, and recommending targeted interventions, AI enhances transparency, accountability, and efficiency in the long-term recovery process, fostering resilient communities and sustainable development post-disaster.
Long-term Recovery Planning and AI:
AI contributes to long-term recovery planning by facilitating evidence-based decision-making, risk management, and resilience-building strategies in disaster-prone regions. AI-powered predictive modeling assesses future risks and vulnerabilities based on historical data, climate projections, and socioeconomic factors. These models inform long-term recovery plans that prioritize investments in resilient infrastructure, ecosystem restoration, and community-based disaster preparedness initiatives. AI-driven simulations simulate various scenarios to test the effectiveness of recovery strategies and optimize resource allocation over the long term. Moreover, AI technologies enable collaboration among stakeholders, including government agencies, international organizations, academia, and local communities, to co-create sustainable recovery plans that address complex challenges such as climate change adaptation, socio-economic recovery, and inclusive development. By harnessing AI for long-term recovery planning, decision-makers can build more resilient societies that are better prepared to withstand future disasters and thrive in a changing world.
Ethical and Practical Considerations
Data Privacy and Security:
Data privacy and security are critical considerations when implementing AI in disaster management. AI systems rely on vast amounts of data, including personal information, geographical data, and sensitive data collected during disaster response and recovery efforts. Protecting this data from unauthorized access, breaches, or misuse is paramount to maintaining trust and compliance with regulations. Robust encryption techniques, access controls, and anonymization practices are essential to safeguarding sensitive data throughout its lifecycle. Additionally, transparency in data handling practices and informed consent mechanisms are crucial to ensuring that individuals affected by disasters understand how their data will be used and protected. By prioritizing data privacy and security, stakeholders can mitigate risks, uphold ethical standards, and maintain public confidence in AI-driven disaster management initiatives.
Real More About Impact of AI on Privacy
Bias and Fairness in AI Algorithms:
Addressing bias and ensuring fairness in AI algorithms is crucial to promoting equitable outcomes in disaster management. AI systems can inadvertently perpetuate biases present in training data, leading to unequal treatment or outcomes for different demographic groups. For example, biased algorithms may disproportionately allocate resources, prioritize response efforts, or assess risks based on factors such as race, gender, or socioeconomic status. Mitigating bias requires careful attention to data selection, algorithm design, and ongoing monitoring to detect and correct biases that may arise. Implementing diverse and inclusive datasets, conducting bias audits, and employing fairness-aware algorithms are steps towards enhancing the fairness and inclusivity of AI applications in disaster management. By striving for fairness, stakeholders can ensure that AI technologies contribute to equitable outcomes and serve the needs of all communities affected by disasters.
Read more about AI Algorithms
The Cost of Implementing AI in Disaster Management:
Implementing AI in disaster management involves significant costs associated with technology development, infrastructure deployment, training, and maintenance of AI systems. These costs encompass expenses related to acquiring AI technologies, integrating them with existing systems, and ensuring compatibility with regulatory requirements and operational needs. Moreover, the complexity of AI solutions may require investment in specialized skills, capacity building, and continuous updates to keep pace with technological advancements. Despite the initial investment, AI can generate long-term cost savings by improving response efficiency, reducing human error, and minimizing the economic impact of disasters. Cost-effectiveness considerations should weigh the potential benefits of AI in enhancing preparedness, response capabilities, and long-term resilience against the upfront costs of implementation. Collaboration and shared resources among stakeholders can help mitigate financial barriers and maximize the value of AI investments in disaster management.
Collaboration Between AI Experts and Disaster Management Professionals:
Effective collaboration between AI experts and disaster management professionals is essential for harnessing the full potential of AI in mitigating disaster risks and improving response outcomes. AI experts bring technical expertise in developing and deploying AI algorithms, data analytics, and predictive modeling capabilities tailored to disaster management contexts. They collaborate with disaster management professionals, including emergency responders, government agencies, NGOs, and community organizations, to understand operational challenges, identify priority needs, and co-design AI solutions that address real-world problems. Collaboration fosters knowledge sharing, interdisciplinary approaches, and innovative solutions that integrate AI technologies with domain-specific knowledge and operational experience. By working together, AI experts and disaster management professionals can leverage each other’s strengths to enhance disaster preparedness, response effectiveness, and community resilience in the face of increasingly complex and dynamic disaster scenarios.
Future Trends in AI and Disaster Management
Advancements in AI Technologies:
Advancements in AI technologies are continuously reshaping disaster management practices, offering new capabilities to predict, prepare for, respond to, and recover from disasters more effectively. Key advancements include the development of more sophisticated machine learning algorithms capable of handling complex data sets and making real-time decisions. Natural language processing (NLP) enables AI systems to analyze textual data from various sources for improved situational awareness and decision support. Computer vision advancements enhance the accuracy of image and video analysis for damage assessment and search-and-rescue operations. Robotics advancements integrate AI for autonomous navigation, manipulation of objects, and interaction with environments in hazardous conditions. These advancements in AI technologies empower disaster management professionals with enhanced tools and capabilities to save lives, mitigate risks, and build resilient communities.
Integration of AI with Other Emerging Technologies (e.g., IoT, Blockchain):
The integration of AI with other emerging technologies such as the Internet of Things (IoT) and blockchain is revolutionizing disaster management by enhancing data collection, transparency, and efficiency. IoT devices, including sensors and drones, collect real-time data on environmental conditions, infrastructure status, and human activities. AI algorithms process and analyze this data to provide actionable insights for early warning systems, resource allocation, and decision-making during disasters. Blockchain technology ensures data integrity, transparency, and traceability in supply chains, financial transactions, and aid distribution. AI-driven analytics combined with blockchain enhance accountability, streamline logistics, and prevent fraud in disaster response operations. The synergy between AI, IoT, and blockchain fosters a resilient ecosystem where real-time data-driven decisions and transparent operations improve overall disaster preparedness, response effectiveness, and recovery outcomes.
The Role of AI in Climate Change Adaptation:
AI plays a pivotal role in climate change adaptation by enabling proactive measures to mitigate the impact of climate-related disasters and build resilience in vulnerable communities. AI-powered predictive models analyze historical climate data, satellite imagery, and environmental parameters to forecast extreme weather events such as hurricanes, floods, droughts, and wildfires with greater accuracy. This forecasting capability supports early warning systems and enables timely evacuation and preparedness measures. AI algorithms optimize energy consumption, resource allocation, and urban planning to reduce greenhouse gas emissions and promote sustainable development practices. Moreover, AI facilitates climate risk assessments, ecosystem monitoring, and adaptive management strategies to safeguard biodiversity and natural habitats. By integrating AI into climate change adaptation efforts, governments, organizations, and communities can enhance their capacity to respond effectively to climate-related challenges and promote sustainable resilience strategies.
Potential Challenges and Solutions:
Implementing AI in disaster management poses several challenges that require careful consideration and proactive solutions. One challenge is the ethical use of AI, including data privacy, bias, and fairness in algorithmic decision-making. Robust data governance frameworks, transparency measures, and bias mitigation techniques are essential to uphold ethical standards and trust in AI applications. Another challenge is the complexity and interoperability of AI systems with existing infrastructure and technologies. Standardization efforts, interoperable platforms, and collaborative partnerships can address these challenges by ensuring seamless integration and scalability of AI solutions across different disaster scenarios and regions.
Conclusion
In conclusion, the integration of artificial intelligence (AI) into disaster management represents a paradigm shift in how societies prepare for and respond to crises. AI technologies offer unprecedented capabilities in predictive analytics, real-time data analysis, decision support, and autonomous operations, fundamentally transforming disaster resilience and response efforts. By harnessing AI, stakeholders can enhance early warning systems, optimize resource allocation, streamline logistics, and improve coordination among response teams. These advancements not only enable quicker and more effective responses to disasters but also contribute to long-term recovery and resilience building. Throughout this discussion, we explored various facets of AI in disaster management. We started by examining AI applications such as predictive analytics for disaster risk assessment and real-time data analysis for decision support. Drones and robots equipped with AI enhance search and rescue operations, while AI-driven communication systems improve coordination among response efforts. We also discussed the integration of AI with other emerging technologies like IoT and blockchain, highlighting their synergistic benefits in enhancing resilience and transparency. Furthermore, we explored AI’s role in climate change adaptation, emphasizing its potential to forecast and mitigate climate-related disasters. The transformative potential of AI in disaster management lies in its ability to innovate and optimize every phase of disaster preparedness, response, recovery, and adaptation. AI enables proactive planning, rapid response, and data-driven decision-making, thereby saving lives, reducing economic losses, and building more resilient communities. By leveraging AI technologies, stakeholders can anticipate risks, mitigate impacts, and foster sustainable development in the face of increasingly complex and frequent disasters. As AI continues to evolve and integrate with other technologies, its role in disaster management will only become more pivotal, shaping a future where communities are better prepared and more resilient in the face of adversity.
FAQs
What is the primary role of AI in disaster management?
The primary role of AI in disaster management is to enhance the capabilities of monitoring, predicting, and responding to various types of disasters. AI systems analyze vast amounts of data from multiple sources, such as satellite imagery, social media, weather forecasts, and sensor networks, to detect early signs of natural or man-made disasters. These systems can identify patterns and anomalies that might indicate an impending disaster, enabling early warning systems to alert authorities and populations. Additionally, AI facilitates real-time decision-making during disaster events by providing situational awareness, resource allocation recommendations, and logistical support to first responders. By automating these processes, AI helps reduce response times, minimize human error, and improve overall disaster management efficiency.
How does AI improve the efficiency of disaster response efforts?
AI improves the efficiency of disaster response efforts by automating data analysis, enhancing communication, and optimizing resource deployment. For example, AI algorithms can quickly process satellite and drone imagery to assess damage, identify blocked roads, and locate survivors, allowing emergency teams to prioritize and allocate resources more effectively. Machine learning models can predict the impact of disasters on infrastructure and populations, guiding pre-emptive evacuations and preparations. AI-powered chatbots and virtual assistants can disseminate accurate information to the public, manage inquiries, and reduce the burden on human operators. Furthermore, AI can streamline logistics by predicting supply chain disruptions and suggesting optimal routes for delivering aid. Overall, AI’s ability to process large datasets, provide real-time insights, and support decision-making significantly enhances the speed and accuracy of disaster response.
What are the main challenges of integrating AI into disaster management?
Integrating AI into disaster management faces several challenges, including data quality, interoperability, and trust. High-quality, real-time data is essential for AI systems to function effectively, yet collecting and maintaining such data can be difficult due to infrastructure limitations, especially in developing regions. Ensuring interoperability between AI systems and existing disaster management frameworks is another challenge, as these systems often rely on different data formats and communication protocols. Additionally, there is a need to build trust among stakeholders—emergency responders, government agencies, and the public—regarding AI’s reliability and decision-making processes. Concerns about transparency, accountability, and the potential for bias in AI algorithms must be addressed to ensure that these systems are accepted and effectively utilized. Lastly, the ethical and privacy implications of using AI in sensitive situations require careful consideration and governance.
Can AI help in predicting natural disasters accurately?
AI holds significant promise in improving the accuracy of natural disaster predictions by leveraging advanced data analytics and machine learning techniques. Through the analysis of historical data and real-time sensor inputs, AI models can identify patterns and signals that precede events like earthquakes, hurricanes, floods, and wildfires. For instance, AI can analyze seismic data to forecast earthquakes or evaluate weather patterns to predict hurricanes and their potential paths. These models can also incorporate environmental factors, such as temperature, humidity, and vegetation levels, to predict the likelihood and spread of wildfires. While AI cannot eliminate the inherent uncertainty in predicting natural disasters, it can significantly enhance the precision and lead time of forecasts, enabling better preparedness and mitigation strategies.
What ethical considerations are important when using AI in disaster management?
When using AI in disaster management, several ethical considerations are crucial to ensure that these technologies are used responsibly and equitably. First, issues of transparency and accountability must be addressed. It is essential that AI systems are transparent in their operations and that the decision-making processes are understandable to stakeholders. Accountability mechanisms should be in place to address errors or biases in AI predictions and recommendations. Second, the potential for bias in AI algorithms must be mitigated to avoid disproportionately affecting vulnerable populations. Data privacy is another critical concern, as the collection and analysis of large datasets for AI applications should comply with regulations and respect individual privacy rights. Finally, the deployment of AI in disaster management should promote inclusivity and equitable access to ensure that all communities benefit from advancements in technology and are not left behind. Ethical frameworks and guidelines must be established to govern the development and use of AI in this sensitive field.