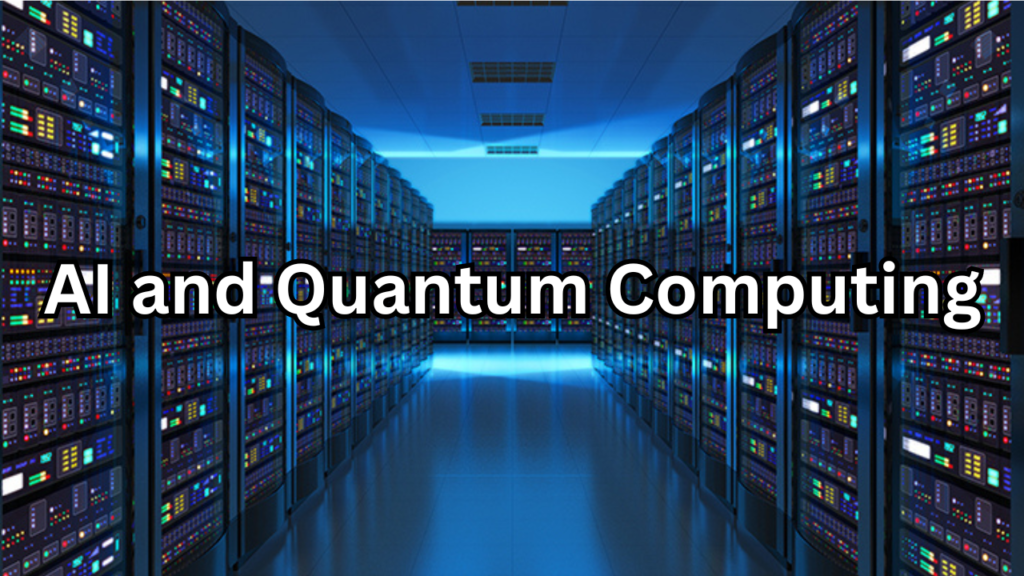
Table of Contents
Introduction
In an era defined by rapid technological advancements, the convergence of artificial intelligence (AI) and quantum computing stands out as a particularly transformative development. AI, with its ability to process and analyze vast amounts of data, has already revolutionized numerous fields, from healthcare to finance. Quantum computing, on the other hand, promises to solve complex problems that are currently beyond the reach of classical computers by leveraging the principles of quantum mechanics. The intersection of these two groundbreaking technologies holds immense potential, offering solutions to some of the world’s most pressing challenges and opening up new frontiers in scientific discovery and innovation.
Overview of AI and Quantum Computing
Artificial Intelligence (AI) refers to the simulation of human intelligence processes by machines, especially computer systems. These processes include learning, reasoning, and self-correction. AI technologies encompass machine learning, where systems can learn from data, and deep learning, which involves neural networks with many layers. Quantum computing, in contrast, is a type of computation that utilizes quantum bits (qubits). Unlike classical bits, which are binary, qubits can exist simultaneously in multiple states thanks to the principles of superposition and entanglement. This allows quantum computers to perform certain calculations much more efficiently than classical computers, potentially solving complex problems that were previously intractable.
Importance of the Intersection Between AI and Quantum Computing
The intersection of AI and quantum computing is significant because it combines the strengths of both technologies to create powerful new capabilities. AI can benefit from the enhanced processing power of quantum computers, allowing for more complex models and faster data analysis. Conversely, quantum computing can utilize AI algorithms to optimize its processes and make sense of the vast amounts of data generated by quantum experiments. This synergy can lead to breakthroughs in various fields, including cryptography, materials science, and drug discovery, accelerating advancements that could significantly impact our daily lives and global progress.
Purpose and Scope of the Article
The purpose of this article is to explore the dynamic and rapidly evolving relationship between AI and quantum computing. It aims to provide a comprehensive understanding of each technology, their individual advancements, and the potential they hold when combined. By delving into the technological foundations, practical applications, challenges, and future prospects, the article seeks to offer readers a thorough insight into this cutting-edge intersection. Additionally, it will address ethical considerations and anticipated industry impacts, ensuring a well-rounded discussion that highlights both the opportunities and the complexities involved in the convergence of AI and quantum computing.
Understanding AI
Definition and Basics of AI
Artificial Intelligence (AI) refers to the creation of machines or systems capable of performing tasks that typically require human intelligence. These tasks include learning from experience, recognizing patterns, understanding natural language, making decisions, and solving problems. AI systems can be categorized into narrow AI, which is designed for specific tasks like speech recognition or image classification, and general AI, which aims to perform any intellectual task that a human can do. The core of AI lies in its ability to process large amounts of data, identify patterns, and make decisions with minimal human intervention, thereby enhancing efficiency and effectiveness in various applications.
Historical Evolution of AI
The concept of AI dates back to ancient times, with myths and stories of artificial beings imbued with intelligence. However, the formal development of AI began in the mid-20th century. In 1956, the term “artificial intelligence” was coined at the Dartmouth Conference, marking the birth of AI as a field of study. Early research focused on symbolic AI and rule-based systems, but progress was slow due to limited computational power and data availability. The 1980s saw the rise of expert systems, which used rules to mimic human decision-making. The late 1990s and early 2000s brought significant advancements with the advent of machine learning, where algorithms could learn from data without being explicitly programmed. The most recent wave, driven by deep learning and neural networks, has led to breakthroughs in image and speech recognition, natural language processing, and autonomous systems.
Key Technologies in AI: Machine Learning, Deep Learning, and Neural Networks
Machine Learning
Machine Learning (ML) is a subset of AI that enables systems to learn and improve from experience without being explicitly programmed. ML algorithms use statistical techniques to analyze and interpret complex data, identifying patterns and making predictions. Supervised learning, unsupervised learning, and reinforcement learning are the three main types of machine learning. Supervised learning involves training a model on labeled data, while unsupervised learning deals with unlabeled data, seeking to uncover hidden patterns. Reinforcement learning uses a reward-based system to train models to make a sequence of decisions.
Deep Learning
Deep Learning is a specialized branch of machine learning that involves neural networks with multiple layers, known as deep neural networks. These networks mimic the human brain’s structure and function, enabling machines to process and learn from large amounts of data with high accuracy. Deep learning has been particularly successful in fields such as computer vision, natural language processing, and speech recognition. The ability of deep neural networks to automatically extract features from raw data without manual intervention has revolutionized AI applications, leading to significant improvements in performance and capabilities.
Neural Networks
Neural Networks are computational models inspired by the human brain’s neural architecture. They consist of interconnected nodes, or neurons, organized in layers: an input layer, one or more hidden layers, and an output layer. Each neuron receives input, processes it, and passes the result to the next layer. Neural networks learn by adjusting the weights of connections based on the error of the output compared to the expected result. This process, known as backpropagation, enables the network to improve its performance over time. Neural networks are the foundation of many advanced AI systems, particularly in deep learning, where they are used to model complex patterns and relationships in data.
Understanding Quantum Computing
Definition and Basics of Quantum Computing
Quantum computing is an advanced area of computing that leverages the principles of quantum mechanics to process information in fundamentally different ways compared to classical computing. While classical computers use bits as the smallest unit of information, which can be either 0 or 1, quantum computers use quantum bits, or qubits. Qubits can exist in multiple states simultaneously due to quantum phenomena such as superposition and entanglement, allowing quantum computers to perform complex calculations at speeds unattainable by classical computers. This capability makes quantum computing particularly promising for solving certain types of problems in fields like cryptography, optimization, and material science.
Historical Evolution of Quantum Computing
The theoretical foundation of quantum computing was laid in the early 1980s by physicists like Richard Feynman and David Deutsch, who proposed that quantum systems could be used to simulate physical processes that classical computers could not efficiently handle. In 1985, Deutsch introduced the concept of a universal quantum computer. Throughout the 1990s, theoretical advancements continued, including Peter Shor’s development of a quantum algorithm for factoring large numbers, which demonstrated quantum computing’s potential to break widely-used cryptographic systems. The early 2000s saw the first experimental demonstrations of basic quantum operations. Since then, progress has accelerated, with companies like IBM, Google, and startups such as D-Wave and Rigetti developing increasingly sophisticated quantum processors. In 2019, Google claimed quantum supremacy by performing a specific calculation faster than the world’s fastest supercomputers. Today, quantum computing research and development continue to advance rapidly, moving closer to practical and scalable quantum computers.
Key Principles: Superposition, Entanglement, and Quantum Gates
Superposition
Superposition is a fundamental principle of quantum mechanics that allows a qubit to exist in multiple states simultaneously. Unlike a classical bit, which must be either 0 or 1, a qubit can be in a state that is a combination, or superposition, of both 0 and 1. This property enables quantum computers to process a vast amount of information concurrently, exponentially increasing their computational power for certain tasks. Superposition is often illustrated by the analogy of Schrödinger’s cat, which is simultaneously alive and dead until observed.
Entanglement
Entanglement is another core principle of quantum mechanics, where two or more qubits become interconnected such that the state of one qubit instantaneously influences the state of the other, regardless of the distance separating them. This phenomenon, described by Einstein as “spooky action at a distance,” allows entangled qubits to share information instantaneously, providing a significant advantage in quantum computing for tasks that require coordinated operations across qubits. Entanglement is crucial for quantum algorithms and protocols, including quantum teleportation and quantum key distribution.
Quantum Gates
Quantum gates are the basic building blocks of quantum circuits, similar to classical logic gates in conventional computers. These gates manipulate qubits through quantum operations, altering their states in a controlled manner. Unlike classical gates that operate on binary values, quantum gates utilize superposition and entanglement to perform complex transformations on qubits. Common quantum gates include the Hadamard gate, which creates superposition, the Pauli-X gate, which acts as a quantum version of the NOT gate, and the CNOT gate, which entangles qubits. Quantum gates are reversible, meaning that the operations can be undone, a property that is essential for the functioning of quantum algorithms. By combining these gates, quantum circuits can be designed to perform a wide range of computational tasks.
The Synergy of AI and Quantum Computing
How AI Benefits from Quantum Computing
Artificial Intelligence (AI) stands to gain significantly from the computational power offered by quantum computing. One of the most profound benefits is the ability to handle and process massive datasets at unprecedented speeds. Quantum computers can perform complex calculations and optimizations much faster than classical computers, allowing AI models to be trained more efficiently. This acceleration can lead to the development of more sophisticated algorithms and models, capable of solving intricate problems in a fraction of the time required today.
How Quantum Computing Benefits from AI
Quantum computing itself can benefit from AI through the optimization and control of quantum systems. AI algorithms can be used to identify and correct errors in quantum computations, a critical function given the fragile nature of quantum states. Machine learning techniques can predict and mitigate noise and decoherence, which are significant challenges in maintaining qubit stability and coherence over time. Moreover, AI can aid in the development of quantum algorithms. Designing efficient quantum algorithms is complex and requires deep expertise. AI can automate parts of this process, using techniques like reinforcement learning to discover new quantum algorithms or optimize existing ones. This synergy can accelerate the progress in quantum computing by enabling more robust and efficient quantum operations, ultimately making quantum computers more practical and accessible.
Potential Combined Applications
The combination of AI and quantum computing holds transformative potential across various fields. In drug discovery, for instance, quantum computing can simulate molecular structures and interactions at a granular level, while AI can analyze these simulations to identify promising drug candidates. This synergy could significantly reduce the time and cost associated with developing new medications. In financial modeling, AI and quantum computing can work together to optimize investment strategies and risk assessments. Quantum algorithms can solve complex optimization problems that are beyond the reach of classical computers, while AI can interpret the results to provide actionable insights for financial institutions.
Technological Foundations
Quantum Algorithms Enhancing AI
Quantum algorithms have the potential to revolutionize artificial intelligence by vastly improving the efficiency and capability of AI systems. One of the most notable quantum algorithms is Shor’s algorithm, which can factorize large numbers exponentially faster than classical algorithms, posing significant implications for cryptography. Similarly, Grover’s algorithm offers quadratic speedups for unstructured search problems, which can be applied to optimize machine learning algorithms. These algorithms can reduce the time and computational resources required for tasks such as training complex neural networks, optimizing large datasets, and solving combinatorial problems. By leveraging quantum algorithms, AI systems can achieve higher performance levels, enabling more advanced applications and accelerating the development of next-generation AI technologies.
AI Techniques in Quantum Computing Development
AI techniques are instrumental in advancing quantum computing development, particularly in addressing the challenges of error correction and noise reduction. Machine learning algorithms can be employed to predict and mitigate errors in quantum computations, enhancing the stability and reliability of quantum systems. AI can also assist in the calibration and optimization of quantum hardware, ensuring that qubits operate at their highest potential. Furthermore, AI-driven simulations can model quantum systems and predict their behavior, providing valuable insights that guide the design of more efficient quantum algorithms and hardware. By integrating AI into quantum computing development, researchers can overcome some of the most significant hurdles in the field, paving the way for more practical and scalable quantum computers.
Quantum Machine Learning
Quantum Machine Learning (QML) is an emerging interdisciplinary field that combines principles of quantum computing with machine learning techniques. QML leverages the computational power of quantum computers to enhance traditional machine learning models, allowing for faster processing and more accurate predictions. Quantum versions of classical algorithms, such as quantum neural networks and quantum support vector machines, can process large datasets more efficiently and uncover patterns that classical algorithms might miss. QML holds promise for various applications, including image and speech recognition, natural language processing, and complex data analysis. By harnessing the unique properties of quantum computing, QML aims to solve problems that are currently intractable for classical machine learning, opening new frontiers in AI research and application.
Practical Applications
AI and Quantum Computing in Drug Discovery
The combination of AI and quantum computing holds immense promise for revolutionizing drug discovery. Traditional drug discovery processes are time-consuming and costly, often taking years and billions of dollars to bring a new drug to market. Quantum computing can drastically accelerate this process by simulating molecular structures and interactions at a quantum level, providing insights into how potential drug compounds interact with their targets in the body. AI algorithms can then analyze these simulations, identifying promising candidates and optimizing their properties for efficacy and safety. This synergy enables researchers to explore a broader chemical space more efficiently, reducing the time and cost associated with drug development. Moreover, AI-driven models can predict the outcomes of biological experiments and clinical trials, further streamlining the pipeline from discovery to market. Together, AI and quantum computing have the potential to transform drug discovery, leading to faster development of new treatments and personalized medicine.
AI and Quantum Computing in Financial Modeling
In the financial sector, AI and quantum computing are poised to revolutionize financial modeling and decision-making processes. Quantum computing can solve complex optimization problems and perform simulations at unprecedented speeds, making it possible to analyze vast datasets and model intricate financial systems more accurately. AI algorithms can leverage these quantum capabilities to enhance risk assessment, portfolio optimization, and predictive modeling. For instance, quantum algorithms can optimize asset allocation by evaluating countless possible combinations in real time, something that classical computers struggle to achieve. AI can then interpret these results, providing financial institutions with actionable insights to make more informed decisions. Additionally, AI and quantum computing can improve fraud detection by analyzing transaction data for anomalies and patterns indicative of fraudulent activity. By combining AI’s predictive power with quantum computing’s computational prowess, the financial industry can achieve greater efficiency, accuracy, and security in its operations.
AI and Quantum Computing in Cryptography
The intersection of AI and quantum computing presents both challenges and opportunities in the field of cryptography. Quantum computing poses a significant threat to traditional cryptographic methods, as algorithms like Shor’s can break widely-used encryption schemes such as RSA and ECC by efficiently factorizing large integers. This potential vulnerability necessitates the development of quantum-resistant cryptographic algorithms. AI can play a crucial role in this effort by designing and testing new cryptographic protocols that are robust against quantum attacks. Additionally, AI can enhance quantum cryptography methods, such as Quantum Key Distribution (QKD), which leverages the principles of quantum mechanics to ensure secure communication. AI algorithms can optimize the implementation and performance of QKD systems, making them more practical for widespread use. Furthermore, AI can be used to analyze and predict potential security threats, helping to safeguard cryptographic systems in a quantum era. By combining AI with quantum computing, researchers can develop innovative solutions to protect sensitive information and maintain data security in the face of emerging quantum threats.
Challenges and Limitations
Technical Challenges in AI
Artificial Intelligence (AI) faces several technical challenges that researchers and developers continue to grapple with. One significant challenge is the issue of interpretability and explainability of AI models. Deep learning, in particular, often operates as a “black box,” making it difficult to understand how decisions are made. This lack of transparency poses risks in critical applications where understanding the reasoning behind AI decisions is crucial. Another challenge is the need for large labeled datasets for training AI models effectively. Acquiring and preparing high-quality data that accurately represents real-world scenarios can be costly and time-consuming. Additionally, AI models can suffer from bias if the training data is not diverse or representative, leading to unfair or discriminatory outcomes. Overcoming these technical challenges requires advancements in algorithm design, data collection and management, and ethical considerations to ensure AI systems are reliable, transparent, and unbiased in their operations.
Technical Challenges in Quantum Computing
Quantum computing presents several formidable technical challenges that limit its current practicality and scalability. One major challenge is qubit coherence and stability. Qubits are highly sensitive to external disturbances and noise, which can cause them to lose their quantum state, a phenomenon known as decoherence. Maintaining qubit coherence over time is crucial for performing reliable quantum computations. Another challenge is error correction. Quantum systems are susceptible to errors due to environmental interactions and imperfect control mechanisms. Developing robust error correction codes and protocols that can effectively detect and correct errors without compromising computational performance is essential for building reliable quantum computers. Furthermore, scaling quantum systems to accommodate a larger number of qubits while maintaining coherence and minimizing errors remains a significant technical hurdle. Current quantum processors have limited qubit counts and are prone to high error rates, posing challenges for implementing complex quantum algorithms and achieving quantum advantage over classical computers. Addressing these technical challenges requires advances in quantum hardware design, error mitigation strategies, and breakthroughs in quantum error correction and fault-tolerant quantum computing.
Integration Challenges and Compatibility Issues
Integrating AI and quantum computing poses unique challenges related to compatibility, interoperability, and resource allocation. AI algorithms often require massive computational power and specialized hardware accelerators, such as GPUs, to train and deploy models effectively. Quantum computing, on the other hand, relies on different hardware architectures and operates under distinct physical principles, such as superconducting qubits or trapped ions. Integrating these disparate technologies requires developing hybrid architectures that can effectively leverage the strengths of both AI and quantum computing without sacrificing performance or efficiency. Additionally, ensuring compatibility between AI software frameworks and quantum programming languages poses challenges in terms of data exchange, communication protocols, and software interfaces. Resource allocation is another critical consideration, as both AI and quantum computing require significant computational resources and energy consumption. Balancing resource allocation to optimize performance and minimize costs is essential for practical deployment in real-world applications. Addressing integration challenges and compatibility issues involves interdisciplinary collaboration between AI researchers, quantum physicists, and software engineers to develop unified frameworks, standards, and tools that facilitate seamless integration and harness the combined potential of AI and quantum computing effectively.
Ethical Considerations
Ethical Implications of AI
Artificial Intelligence (AI) raises significant ethical concerns that encompass privacy, bias, accountability, and job displacement. One major ethical issue is privacy invasion, as AI systems often collect and analyze vast amounts of personal data without explicit consent or awareness from individuals. This raises concerns about data security, consent, and the potential misuse of sensitive information. Moreover, AI algorithms can perpetuate biases present in training data, leading to discriminatory outcomes in decision-making processes such as hiring, loan approvals, and law enforcement. Ensuring fairness and transparency in AI algorithms is crucial to mitigate these biases and promote equitable outcomes.
Ethical Implications of Quantum Computing
Quantum computing introduces unique ethical considerations related to security, privacy, and international competitiveness. One of the primary ethical concerns is the potential impact on cybersecurity. Quantum computers could potentially break current encryption methods, threatening the security of sensitive data and communications worldwide. Ensuring the development and adoption of quantum-resistant encryption techniques is essential to safeguard privacy and maintain trust in digital systems.
Ethical Considerations in Combined Use
The combined use of AI and quantum computing introduces novel ethical considerations that require careful attention and regulation. One key ethical concern is transparency and accountability in AI-enhanced quantum algorithms. As these algorithms become more complex and autonomous, ensuring transparency in decision-making processes and accountability for outcomes becomes increasingly challenging yet essential. Clear ethical guidelines and governance frameworks are needed to address these issues and maintain public trust.
Future Prospects
Upcoming Innovations in AI
The future of Artificial Intelligence (AI) holds promise for groundbreaking innovations across various domains. One of the most anticipated advancements is in the realm of natural language processing (NLP) and understanding. AI models are expected to achieve greater fluency in conversational interactions, enabling more sophisticated virtual assistants and chatbots that can understand context, emotions, and nuances in human language. Moreover, AI-driven creativity is on the rise, with developments in generative models capable of producing art, music, and literature that rivals human creations. Enhanced AI capabilities in healthcare are also anticipated, with advancements in medical imaging analysis, personalized treatment plans, and drug discovery accelerating progress towards precision medicine. Furthermore, AI’s integration with edge computing and Internet of Things (IoT) devices is expected to revolutionize automation and decision-making at the edge, enabling real-time insights and responsiveness in diverse applications from smart cities to autonomous vehicles. As AI continues to evolve, its potential to transform industries, improve quality of life, and drive economic growth remains a compelling focus of innovation and research worldwide.
Upcoming Innovations in Quantum Computing
The future of Quantum Computing promises revolutionary advancements that could redefine the limits of computational power and problem-solving capabilities. One of the most anticipated innovations is the achievement of quantum supremacy in practical applications, where quantum computers outperform classical supercomputers on specific tasks. This milestone is expected to unlock new possibilities in fields such as materials science, cryptography, and optimization, paving the way for breakthrough discoveries and technological advancements. Moreover, advancements in quantum error correction and fault-tolerant quantum computing are crucial for scaling up quantum systems and improving their reliability and performance. Quantum algorithms tailored for specific applications, such as quantum machine learning and quantum simulations, are anticipated to demonstrate unprecedented computational speed and efficiency, enabling solutions to complex problems that are currently intractable with classical methods. As research and development in quantum computing accelerate, collaborations between academia, industry, and governments are driving the quest for practical quantum technologies that promise to revolutionize industries and society in the decades to come.
Predicted Impact on Various Industries
The predicted impact of AI and quantum computing on various industries is transformative, promising to reshape business models, enhance productivity, and drive innovation across sectors. In healthcare, AI’s predictive analytics and diagnostic capabilities are expected to improve patient outcomes through personalized treatment plans, early disease detection, and precision medicine. Quantum computing’s ability to simulate molecular structures and interactions could revolutionize drug discovery and development, accelerating the discovery of new therapies and treatments. In finance, AI-powered algorithms are anticipated to optimize investment strategies, risk management, and fraud detection, while quantum computing could revolutionize portfolio optimization, trading strategies, and risk assessment with unprecedented computational power and speed. Additionally, in manufacturing and logistics, AI-driven automation and optimization are expected to streamline operations, reduce costs, and enhance supply chain efficiency. Quantum computing’s potential to solve complex optimization problems could further enhance logistical planning and resource allocation. Moreover, in telecommunications and cybersecurity, AI and quantum computing are poised to strengthen network security, enhance data encryption, and protect against emerging threats. As industries adapt and integrate these technologies, the transformative impact of AI and quantum computing on global economies and societies is expected to be profound, driving innovation, competitiveness, and sustainable growth in the digital age.
Summary
The synergy between Artificial Intelligence (AI) and Quantum Computing represents a frontier of innovation with profound implications across industries and disciplines. AI, powered by machine learning and neural networks, enhances data processing, pattern recognition, and decision-making. Quantum computing, leveraging principles like superposition and entanglement, offers unprecedented computational power to solve complex problems at speeds unimaginable with classical computing. Together, AI benefits from quantum computing’s ability to accelerate algorithmic efficiency and solve previously intractable problems, while quantum computing benefits from AI’s predictive capabilities and algorithmic enhancements. The combined potential of AI and quantum computing promises transformative advancements in healthcare, finance, cybersecurity, and beyond, revolutionizing how we approach scientific research, business strategies, and societal challenges in the coming decades.
FAQs
What is the primary advantage of combining AI and Quantum Computing?
The primary advantage of combining AI and Quantum Computing lies in their complementary strengths. AI excels in processing vast amounts of data, learning patterns, and making predictions. Quantum computing, on the other hand, offers exponential computational power, enabling complex calculations and optimizations that classical computers cannot perform efficiently. Together, AI can enhance quantum computing by optimizing algorithms, improving error correction, and interpreting results. Conversely, quantum computing can accelerate AI by speeding up training processes, enhancing model accuracy, and solving complex problems faster. This synergy promises to unlock new frontiers in machine learning, optimization, and scientific research, pushing the boundaries of what is possible in technology and innovation.
How soon can we expect practical applications from this synergy?
Practical applications from the synergy between AI and quantum computing are already emerging in research and development labs. While widespread commercial adoption may still be several years away, promising advancements in quantum machine learning, quantum cryptography, and optimization algorithms are underway. Companies and research institutions are actively exploring applications in drug discovery, financial modeling, cybersecurity, and more. Quantum computing hardware continues to evolve, with companies like IBM, Google, and startups like Rigetti and IonQ making significant strides in developing scalable quantum processors. As these technologies mature and overcome current technical challenges, practical applications are expected to accelerate, potentially within the next decade.
Are there any prominent companies or research institutions leading in this field?
Several prominent companies and research institutions are leading the charge in AI and quantum computing integration. In quantum computing, companies like IBM, Google, Microsoft, and startups such as Rigetti Computing, IonQ, and D-Wave Systems are at the forefront of developing quantum hardware and algorithms. These companies are actively collaborating with academic institutions and government research labs to advance quantum technologies and explore practical applications. In AI, tech giants like Google, Amazon, Microsoft, and specialized AI research labs at universities such as Stanford, MIT, and Carnegie Mellon are driving innovations in machine learning, natural language processing, and computer vision. These organizations play pivotal roles in shaping the future of AI and quantum computing and are key players in the global race for quantum advantage and AI-driven breakthroughs.
What are the main ethical concerns with AI and Quantum Computing?
The main ethical concerns with AI and quantum computing span issues of privacy, bias, security, and societal impact. AI raises concerns about data privacy and security, as algorithms process sensitive personal information and make decisions with potential implications for individuals’ rights and freedoms. Bias in AI algorithms, perpetuated by biased training data, can lead to discriminatory outcomes in areas like hiring, lending, and law enforcement, exacerbating social inequalities. In quantum computing, ethical concerns include the potential disruption of cybersecurity through quantum-enabled attacks on encryption systems, compromising data integrity and confidentiality. Moreover, the dual-use nature of quantum technologies raises ethical questions about their application in military, surveillance, and geopolitical contexts. Addressing these ethical challenges requires transparent governance, robust regulation, and interdisciplinary collaboration to ensure responsible development and deployment of AI and quantum computing technologies.
How does Quantum Machine Learning differ from traditional Machine Learning?
Quantum Machine Learning (QML) differs from traditional machine learning primarily in its computational framework and capabilities. Traditional machine learning algorithms, such as supervised learning and neural networks, operate on classical computers using binary bits to process and analyze data. In contrast, QML harnesses the principles of quantum mechanics and quantum computing to perform computations using qubits, which can exist in superposition and entanglement states. This quantum advantage allows QML algorithms to explore vast solution spaces simultaneously, offering potential speedups in processing and optimizing complex datasets. QML algorithms, such as quantum neural networks and quantum support vector machines, aim to enhance pattern recognition, optimization, and decision-making tasks beyond the capabilities of classical machine learning. While still in its infancy, QML holds promise for revolutionizing fields such as drug discovery, financial modeling, and materials science by leveraging the quantum computational power to tackle previously unsolvable problems efficiently.