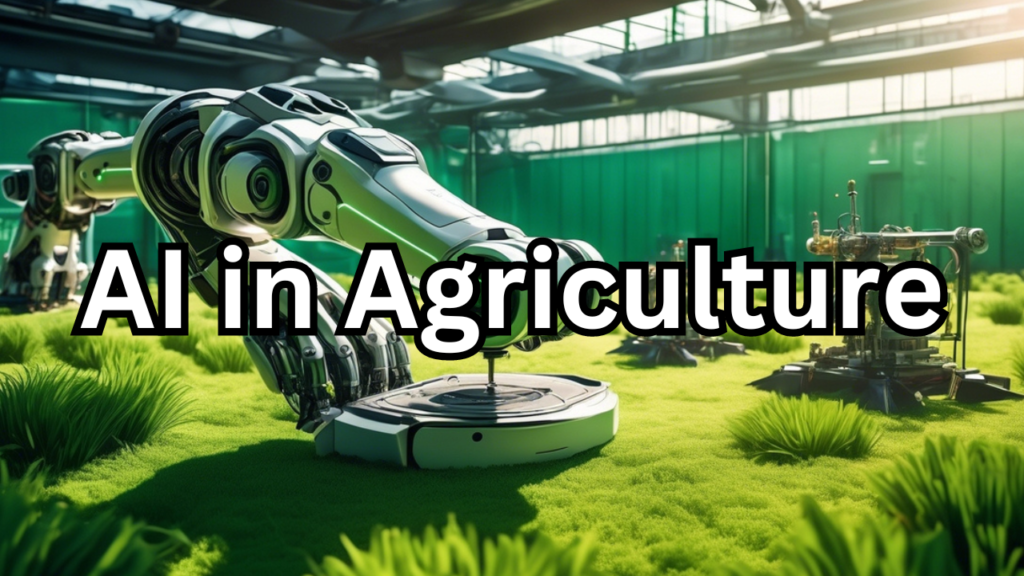
Table of Contents
Introduction to AI in Agriculture
Definition and Scope
AI in agriculture refers to the application of artificial intelligence technologies such as machine learning, computer vision, and data analytics to enhance various aspects of farming and agribusiness. It encompasses a broad range of technologies and techniques aimed at improving efficiency, productivity, and sustainability in agricultural practices. These technologies enable farmers and agricultural stakeholders to gather and analyze data more effectively, make data-driven decisions, and optimize resource management. The scope of AI in agriculture includes precision farming, automated machinery, predictive analytics for weather and crop yields, and smart systems for monitoring and managing livestock health and behavior.
Evolution of AI in Farming Practices
The evolution of AI in farming practices has been marked by significant advancements over the past few decades. Initially, AI applications were limited to basic tasks such as data collection and simple analytics. However, with the advent of more powerful computing systems and the proliferation of IoT devices, AI has become increasingly integrated into every stage of agricultural operations. From the development of autonomous tractors and robots for harvesting to sophisticated algorithms for predicting market trends and optimizing supply chains, AI is revolutionizing how farmers approach cultivation, management, and distribution of agricultural products. This evolution has not only improved productivity but also enabled more sustainable and environmentally friendly farming practices.
Importance of AI in Modern Agriculture
AI plays a crucial role in modern agriculture by addressing key challenges facing the industry, such as population growth, climate change, and the need for increased food production efficiency. By leveraging AI technologies, farmers can achieve higher crop yields with fewer resources, reduce wastage through precise monitoring and management, and mitigate risks associated with unpredictable weather patterns and pest outbreaks. Moreover, AI enables farmers to optimize their operations in real-time, making adjustments based on accurate data and predictive analytics. Beyond operational benefits, AI also contributes to the economic viability of agriculture by enhancing profitability and competitiveness in global markets. In essence, AI is transforming agriculture into a more resilient, sustainable, and technology-driven industry poised to meet the demands of the future.
Applications of AI in Crop Production
Precision Agriculture
Precision agriculture refers to the practice of using advanced technologies such as GPS, sensors, drones, and AI to optimize farming practices at a granular level. This approach allows farmers to tailor their management strategies based on variability within fields, rather than applying uniform treatments across large areas. By collecting and analyzing data on soil characteristics, moisture levels, crop health, and environmental conditions, precision agriculture enables precise application of fertilizers, pesticides, and water. This not only improves resource efficiency but also enhances crop yields and reduces environmental impact by minimizing inputs where they are not needed.
Crop Monitoring and Predictive Analytics
Crop monitoring and predictive analytics involve the continuous monitoring of crops throughout their growth cycle using various technologies such as satellite imagery, drones, and IoT sensors. These technologies capture data on plant health, growth rates, pest infestations, and environmental conditions. AI-driven predictive analytics processes this data to forecast crop yields, identify potential risks such as disease outbreaks or adverse weather conditions, and recommend timely interventions. By providing farmers with actionable insights in real-time, crop monitoring and predictive analytics enable proactive decision-making to optimize production and minimize losses.
Automated Irrigation Systems
Automated irrigation systems leverage AI and IoT technologies to optimize water usage in agriculture. These systems monitor soil moisture levels, weather forecasts, and plant water requirements to deliver precise amounts of water directly to crops. AI algorithms analyze data from sensors to adjust irrigation schedules and water distribution patterns dynamically, ensuring that plants receive the right amount of water at the right time. Automated irrigation systems not only conserve water by minimizing wastage but also enhance crop health and yield by preventing under or over-watering, ultimately contributing to sustainable agriculture practices.
Soil Monitoring and Management
Soil monitoring and management technologies utilize AI-powered sensors and imaging techniques to assess soil quality, fertility levels, and nutrient content. These technologies provide farmers with detailed insights into soil health parameters such as pH levels, organic matter content, and nutrient availability. AI algorithms analyze this data to generate soil maps and recommend customized fertilization and soil amendment strategies. By optimizing soil management practices based on precise data, farmers can improve crop productivity, reduce nutrient runoff into water sources, and promote long-term soil health and sustainability in agricultural landscapes.
AI and Livestock Farming
Monitoring Animal Health
Monitoring animal health in agriculture involves the use of advanced technologies to assess and maintain the well-being of livestock. AI plays a crucial role in this process by enabling continuous monitoring through sensors and wearable devices that track vital signs such as temperature, heart rate, and activity levels. These devices provide real-time data to farmers and veterinarians, allowing early detection of health issues or anomalies. AI algorithms analyze the collected data to identify patterns indicative of illness, injury, or stress, enabling prompt intervention and treatment. By improving the accuracy and timeliness of health monitoring, AI helps farmers enhance animal welfare, reduce mortality rates, and optimize productivity in livestock farming operations.
Automated Feeding Systems
Automated feeding systems in agriculture leverage AI and robotics to optimize the feeding process for livestock. These systems use sensors and data analytics to monitor feed levels, nutritional requirements, and feeding schedules based on the specific needs of individual animals or groups. AI algorithms analyze data on animal growth rates, behavior patterns, and feed consumption to adjust feeding regimes dynamically. Automated feeders dispense precise amounts of feed at optimal times, ensuring consistent nutrition and minimizing wastage. By reducing labor costs and manual errors associated with feeding, automated systems improve efficiency, promote uniform animal growth, and support sustainable management practices in livestock farming.
Behavior Analysis and Predictive Modeling
Behavior analysis and predictive modeling in agriculture involve studying animal behavior patterns and using AI-driven algorithms to forecast future outcomes. AI technologies process vast amounts of behavioral data collected from sensors, cameras, and automated monitoring systems to recognize normal behaviors and detect deviations that may indicate stress, disease, or reproductive cycles. Predictive modeling integrates behavioral data with environmental factors such as weather conditions and feed quality to anticipate health issues, optimize breeding programs, and improve overall animal management strategies. By providing insights into behavioral trends and predictive analytics, AI empowers farmers to make informed decisions, enhance animal welfare, and optimize production efficiency in livestock farming operations.
Robotics in Agriculture
Harvesting Robots
Harvesting robots are advanced agricultural machines designed to automate the process of harvesting crops. These robots utilize AI and robotics technology to perform tasks traditionally done by human laborers, such as picking fruits, vegetables, and other crops. Equipped with sensors, cameras, and AI algorithms, harvesting robots can identify ripe produce, assess its quality, and harvest it with precision and efficiency. By automating harvesting tasks, these robots help address labor shortages in agriculture, reduce dependency on seasonal workers, and improve harvesting speed and accuracy. Additionally, harvesting robots contribute to cost savings and operational efficiency for farmers, enabling timely harvests and minimizing post-harvest losses.
Autonomous Tractors and Machinery
Autonomous tractors and machinery are self-driving vehicles equipped with AI-powered navigation systems and sensors that enable them to perform various agricultural tasks without human intervention. These machines can autonomously plow fields, plant seeds, apply fertilizers and pesticides, and perform other field operations. AI algorithms process data from GPS, cameras, and onboard sensors to navigate fields, avoid obstacles, and adjust operations based on real-time conditions. Autonomous tractors and machinery improve efficiency by working continuously day and night, reducing operational costs associated with labor and fuel. Moreover, they contribute to sustainable agriculture by optimizing resource use, minimizing soil compaction, and promoting precision farming practices.
Drones in Farming Operations
Drones, or unmanned aerial vehicles (UAVs), have become integral tools in modern farming operations due to their versatility and ability to collect valuable data from above. Equipped with cameras, multispectral sensors, and LiDAR technology, drones capture high-resolution images and data on crop health, soil conditions, pest infestations, and field drainage patterns. AI algorithms process this data to generate detailed maps, detect anomalies, and provide actionable insights for farmers. Drones enable efficient monitoring of large agricultural areas that may be challenging or time-consuming to survey manually. They facilitate early detection of issues, such as nutrient deficiencies or crop diseases, allowing farmers to implement targeted interventions and optimize crop management practices. Overall, drones enhance decision-making, improve crop yields, and promote sustainable agricultural practices by reducing chemical usage and maximizing resource efficiency.
AI in Pest and Disease Management
Early Detection Systems
Early detection systems in agriculture utilize advanced technologies, including AI and IoT sensors, to identify potential threats to crops or livestock at the earliest stages. These systems continuously monitor environmental conditions, plant health parameters, and pest activity to detect deviations from normal patterns. AI algorithms analyze data collected from sensors, satellite imagery, and weather forecasts to recognize early signs of stress, disease, pest infestation, or nutrient deficiencies. By providing timely alerts to farmers, early detection systems enable proactive intervention strategies, such as targeted pest control measures or adjustments in irrigation and fertilization practices. This proactive approach helps minimize crop losses, reduce the need for chemical interventions, and support sustainable agriculture practices.
Pest Identification and Control
Pest identification and control strategies in agriculture are essential for managing and mitigating the impact of pests on crops. AI technologies play a crucial role in accurately identifying pest species and predicting their behavior patterns based on environmental data and historical trends. AI-driven image recognition systems analyze images of pests captured by cameras or drones to identify species and assess population densities. Once pests are identified, AI algorithms recommend appropriate control measures, such as precision application of pesticides or biological control methods, to minimize damage while reducing environmental impact. By optimizing pest management practices, AI helps farmers maintain crop health, preserve yield potential, and ensure food security.
Disease Prevention Strategies
Disease prevention strategies in agriculture focus on proactively managing and preventing the spread of diseases among crops or livestock. AI-powered systems analyze data from sensors, disease models, and historical records to assess disease risk factors such as weather conditions, soil health, and crop rotations. AI algorithms predict disease outbreaks and recommend preventive measures, such as crop rotation, resistant crop varieties, and early application of disease-resistant treatments or biocontrol agents. Moreover, AI facilitates real-time monitoring of disease progression and provides alerts to farmers, enabling prompt responses and targeted interventions. By integrating AI into disease prevention strategies, farmers can minimize disease-related losses, optimize resource use, and promote sustainable agricultural practices that safeguard both crop productivity and environmental health.
AI in Supply Chain Management
Inventory Optimization
Inventory optimization in agriculture involves using advanced data analytics and AI algorithms to manage and streamline inventory levels efficiently. By analyzing historical sales data, current demand trends, and supply chain dynamics, AI can forecast future demand with greater accuracy. This allows farmers and agribusinesses to maintain optimal inventory levels, ensuring they have enough agricultural inputs such as seeds, fertilizers, and pesticides without excess inventory that could lead to waste or unnecessary storage costs. AI-driven inventory optimization also helps in reducing stockouts, ensuring timely availability of critical supplies, and improving overall operational efficiency.
Logistics and Transportation Efficiency
Logistics and transportation efficiency in agriculture are critical for ensuring timely delivery of agricultural products from farms to markets or processing facilities. AI technologies play a pivotal role in optimizing logistics and transportation by analyzing data on factors such as route planning, vehicle scheduling, and real-time traffic conditions. AI algorithms use predictive analytics to optimize delivery routes, minimize transportation costs, and reduce fuel consumption. Additionally, AI-enabled logistics systems provide real-time tracking of shipments, allowing farmers and distributors to monitor the movement of products and respond promptly to any delays or issues. By improving efficiency in logistics and transportation, AI contributes to reducing overall operational costs, enhancing supply chain resilience, and meeting customer demand more effectively.
Predictive Maintenance of Equipment
Predictive maintenance of equipment in agriculture involves using AI and IoT sensors to monitor the condition and performance of farm machinery and equipment. AI algorithms analyze data from sensors that measure factors such as temperature, vibration, and fluid levels to detect anomalies and predict potential failures before they occur. By identifying early signs of equipment deterioration or malfunction, predictive maintenance helps farmers schedule maintenance tasks proactively, avoiding costly unplanned downtime and repairs. Moreover, AI-driven predictive maintenance systems optimize equipment performance, extend lifespan, and reduce overall maintenance costs by enabling efficient allocation of resources and personnel. Ultimately, predictive maintenance enhances operational reliability, productivity, and safety in agricultural operations, supporting sustainable farming practices and maximizing return on investment in equipment.
Data Analytics and AI in Agribusiness
Market Forecasting and Trends
Market forecasting and trend analysis in agriculture involve using data-driven approaches, including AI and machine learning, to predict future market conditions and trends. These technologies analyze historical sales data, weather patterns, global market trends, and other relevant factors to generate accurate forecasts of supply and demand dynamics for agricultural products. By identifying emerging trends and market shifts, farmers, agribusinesses, and policymakers can make informed decisions regarding crop selection, production volumes, pricing strategies, and market timing. AI-driven market forecasting enables proactive planning, helps mitigate risks associated with market fluctuations, and supports strategic business growth in the agriculture sector.
Consumer Behavior Analysis
Consumer behavior analysis in agriculture focuses on understanding the preferences, purchasing patterns, and decision-making processes of agricultural product consumers. AI technologies play a crucial role in analyzing large datasets from consumer surveys, online interactions, and purchase histories to identify trends and insights. AI algorithms utilize techniques such as sentiment analysis and predictive modeling to segment consumer demographics, predict buying behaviors, and anticipate shifts in consumer preferences. By gaining deeper insights into consumer behavior, farmers and agribusinesses can tailor their marketing strategies, product offerings, and distribution channels to meet consumer demand effectively. This understanding helps improve customer satisfaction, build brand loyalty, and drive business growth in the competitive agricultural marketplace.
Financial Decision Support Systems
Financial decision support systems in agriculture integrate AI-powered analytics and data visualization tools to assist farmers and agribusiness managers in making sound financial decisions. These systems analyze financial data, operational metrics, market trends, and risk factors to provide actionable insights and recommendations. AI algorithms generate forecasts, evaluate investment opportunities, optimize resource allocation, and assess financial risks such as fluctuating commodity prices or production costs. By facilitating informed decision-making, financial decision support systems enable farmers to manage cash flow effectively, optimize profitability, and navigate economic uncertainties. Moreover, these systems enhance transparency and accountability in financial management practices, supporting long-term sustainability and growth in agricultural enterprises.
Environmental Impact of AI in Agriculture
Sustainability Practices
Sustainability practices in agriculture involve adopting strategies and techniques that promote the long-term health and viability of farming ecosystems while minimizing environmental impact. These practices encompass a range of initiatives, including soil conservation, water management, biodiversity preservation, and integrated pest management. AI technologies contribute to sustainability by optimizing resource use through precision agriculture techniques such as targeted irrigation, fertilizer application, and pest control. AI-driven data analytics enable farmers to monitor and analyze environmental impacts, carbon footprints, and sustainability metrics more accurately. By implementing sustainable practices, farmers can enhance soil health, conserve natural resources, reduce greenhouse gas emissions, and promote ecosystem resilience, ensuring sustainable food production for future generations.
Resource Conservation
Resource conservation in agriculture focuses on efficiently managing and preserving natural resources such as water, soil, energy, and biodiversity. AI plays a crucial role in resource conservation by optimizing resource utilization through smart technologies and data-driven insights. AI-powered sensors and monitoring systems enable farmers to track soil moisture levels, nutrient content, and crop water requirements in real-time, facilitating precise irrigation and nutrient management. Additionally, AI algorithms analyze data on weather patterns, crop growth stages, and field conditions to optimize planting schedules and minimize resource inputs. By promoting efficient resource use, resource conservation practices in agriculture help mitigate environmental degradation, improve farm profitability, and support sustainable farming practices that benefit both producers and ecosystems.
Climate Change Mitigation
Climate change mitigation in agriculture involves reducing greenhouse gas emissions, enhancing carbon sequestration, and adapting farming practices to mitigate the impacts of climate change. AI technologies contribute to climate change mitigation by providing tools for precision agriculture, predictive modeling, and climate risk assessment. AI-driven analytics assess climate-related risks such as extreme weather events, shifting growing seasons, and pest outbreaks, enabling farmers to implement adaptive strategies and resilient crop management practices. Moreover, AI facilitates the development of carbon farming initiatives that enhance soil carbon storage and promote regenerative agricultural practices. By integrating AI into climate change mitigation efforts, agriculture can play a pivotal role in reducing its environmental footprint, enhancing resilience to climate variability, and contributing to global efforts to combat climate change.
Challenges and Risks of Implementing AI in Agriculture
Technological Adoption Barriers
Technological adoption barriers in agriculture refer to challenges that hinder the widespread implementation of advanced technologies, including AI and IoT, on farms. These barriers may include limited access to technology due to high costs or inadequate infrastructure in rural areas. Farmers may face challenges related to the complexity of adopting new technologies, such as lack of technical skills or knowledge required for integration and operation. Moreover, resistance to change and skepticism about the benefits of technology among traditional farmers can slow down adoption rates. Regulatory hurdles and policy constraints related to technology deployment, particularly in areas such as data ownership and use, may also pose barriers. Addressing technological adoption barriers requires investment in training and education, improving access to affordable technology solutions, fostering supportive policies, and demonstrating clear economic and operational benefits to farmers.
Data Privacy and Security Concerns
Data privacy and security concerns in agriculture arise from the collection, storage, and use of sensitive data generated by AI, IoT devices, and other digital technologies on farms. Farmers and agribusinesses are increasingly reliant on data-driven insights for decision-making, but this reliance raises concerns about the confidentiality, integrity, and ownership of agricultural data. Issues such as unauthorized access, data breaches, and misuse of personal information pose risks to farmers’ privacy and business operations. Moreover, compliance with data protection regulations, which vary globally, adds complexity to managing data privacy in agriculture. Addressing these concerns requires implementing robust cybersecurity measures, including encryption, access controls, and regular audits. Farmers must also establish clear data governance policies, inform stakeholders about data usage practices, and prioritize transparency to build trust in data-driven agricultural technologies.
Economic Viability and Cost Factors
Economic viability and cost factors are critical considerations for farmers and agribusinesses when adopting new technologies in agriculture. While AI, robotics, and IoT promise efficiency gains and productivity improvements, initial investment costs can be significant barriers to adoption. High costs associated with purchasing and maintaining technology hardware and software, as well as upgrading infrastructure, may deter smaller farms or those with limited financial resources from adopting advanced technologies. Additionally, ongoing operational costs, such as training, technical support, and data management, contribute to the overall economic burden. Farmers need to carefully assess the return on investment (ROI) and long-term financial benefits of adopting new technologies compared to traditional farming practices. Government incentives, subsidies, and financing options can help mitigate financial barriers and promote widespread adoption of cost-effective agricultural technologies that enhance profitability, sustainability, and resilience in farming operations.
Future Trends in AI and Agriculture
Integration of AI with IoT and Big Data
The integration of AI with IoT (Internet of Things) and Big Data in agriculture represents a transformative approach to enhancing farm management and decision-making processes. IoT devices such as sensors, drones, and automated machinery generate vast amounts of real-time data from fields, crops, and livestock. AI algorithms analyze this data to derive actionable insights and optimize farming operations. For example, AI can process data from soil moisture sensors and weather forecasts to dynamically adjust irrigation schedules, improving water efficiency. Integrating AI with IoT and Big Data enables predictive analytics for predicting crop yields, detecting pest outbreaks early, and optimizing resource allocation based on environmental conditions. This synergy not only enhances productivity and sustainability but also supports data-driven precision agriculture practices that maximize yield while minimizing input costs and environmental impact.
AI-driven Genetic Engineering
AI-driven genetic engineering in agriculture refers to the application of AI technologies to accelerate the development of new crop varieties and livestock breeds with desired traits. AI algorithms analyze genomic data to identify genetic markers associated with traits such as disease resistance, drought tolerance, and improved yield potential. This analysis speeds up traditional breeding processes by predicting the outcomes of genetic combinations and guiding the selection of breeding pairs. Moreover, AI enables precision editing techniques such as CRISPR-Cas9, which allows researchers to edit specific genes to introduce or enhance desirable traits in plants and animals. AI-driven genetic engineering holds promise for addressing global food security challenges by developing resilient crops that thrive in changing climates and increasing agricultural productivity sustainably.
Policy and Regulatory Developments
Policy and regulatory developments in agriculture play a crucial role in shaping the adoption and deployment of AI technologies and genetic engineering in the farming sector. Governments worldwide are increasingly recognizing the potential benefits and risks associated with these technologies and are developing frameworks to guide their responsible use. Policies may address issues such as data privacy and ownership, intellectual property rights related to genetic modifications, environmental impacts, and ethical considerations. Regulatory bodies work to establish standards for AI algorithms used in farming, ensure transparency in data management practices, and safeguard biodiversity and consumer safety. International collaboration is essential for harmonizing regulatory approaches and facilitating technology transfer while addressing cultural and regional differences. By fostering supportive policies and regulations, governments can promote innovation, mitigate risks, and create a conducive environment for sustainable and equitable adoption of AI and genetic engineering in agriculture.
Ethical Considerations in AI-powered Farming
Fairness and Bias in AI Algorithms
Fairness and bias in AI algorithms are critical considerations in agriculture and other sectors where AI technologies are increasingly integrated. AI systems rely on large datasets to make decisions and predictions, but these datasets can reflect biases present in the data collection process or historical inequalities. In agriculture, biased AI algorithms may disproportionately affect certain demographic groups or regions, leading to inequities in access to resources, services, or opportunities. Addressing fairness and bias requires implementing measures such as data preprocessing to identify and mitigate biases, developing diverse and representative datasets, and using transparent and explainable AI models. Moreover, ongoing monitoring and evaluation of AI systems are essential to ensure fairness and prevent unintended consequences. By promoting fairness in AI algorithms, agriculture can harness the full potential of technology while upholding ethical standards and promoting inclusivity.
Impact on Rural Communities
The impact of AI technologies on rural communities in agriculture is multifaceted, influencing economic, social, and cultural dynamics. On one hand, AI-driven innovations such as precision agriculture and automated machinery offer opportunities to enhance productivity, reduce labor-intensive tasks, and improve livelihoods for rural farmers. These technologies can empower smallholder farmers by providing access to real-time data, market information, and decision support systems that optimize farming practices and increase yields. However, the adoption of AI may also lead to shifts in employment patterns, with potential job displacement in traditional agricultural roles. Moreover, access to and affordability of AI technologies may vary across rural communities, creating disparities in adoption and benefiting larger, wealthier farms over smaller operations. Addressing the impact of AI on rural communities requires policies that promote inclusive development, provide training and support for technology adoption, and foster local innovation ecosystems that benefit all stakeholders.
Human Labor vs. Automation Debate
The debate between human labor and automation in agriculture revolves around balancing the efficiency gains and economic benefits of technology with the social and ethical considerations of workforce displacement and job quality. Automation technologies such as robotics, AI-driven machinery, and automated systems offer potential advantages in terms of productivity, operational efficiency, and cost reduction. These technologies can perform tasks faster and more accurately than human labor, particularly in repetitive or physically demanding roles. However, the shift towards automation raises concerns about the impact on rural livelihoods, the quality of jobs, and the social fabric of farming communities. Proponents argue that automation can free up human resources for higher-value tasks, improve job safety, and attract younger generations to agriculture by offering opportunities in technology-driven roles. Critics emphasize the importance of preserving traditional farming practices, supporting rural employment, and ensuring equitable distribution of benefits from technological advancements. Achieving a balanced approach requires collaborative efforts among policymakers, farmers, industry stakeholders, and communities to optimize the use of technology while safeguarding the well-being and sustainability of agricultural economies and societies.
Summary of AI’s Influence on Agriculture
AI’s influence on agriculture has been profound, revolutionizing traditional farming practices through advanced technologies such as machine learning, robotics, and data analytics. These innovations enable farmers to make data-driven decisions, optimize resource management, and enhance productivity while promoting sustainability. Key benefits include improved efficiency and productivity, enhanced resource management, and sustainability practices like precise monitoring of crops and livestock, reducing environmental impact, and supporting resilient farming systems. However, challenges include high initial costs, infrastructure limitations, data privacy concerns, regulatory complexities, and the need to address biases in AI algorithms for fairness. Looking ahead, the future of AI in agriculture holds promise for continued innovation, with integrated IoT and big data analytics creating interconnected farming ecosystems that optimize production efficiency and environmental stewardship. Enhanced AI capabilities will support personalized agriculture, fostering inclusive growth and resilience in global food systems through responsible and ethical AI deployment.
FAQs about AI in Agriculture
What is precision agriculture?
Precision agriculture refers to the use of advanced technologies, including AI, IoT, and data analytics, to optimize farming practices at a precision level. It involves collecting real-time data on soil conditions, weather patterns, crop health, and other relevant factors to make informed decisions. By leveraging AI, precision agriculture enables farmers to apply inputs such as water, fertilizers, and pesticides precisely where and when they are needed, maximizing efficiency, reducing waste, and enhancing crop yields.
How does AI help in pest management?
AI aids in pest management by facilitating early detection, prediction, and control of pest outbreaks. AI algorithms analyze data from sensors, satellites, and historical pest patterns to detect signs of pest presence or potential outbreaks. This early detection allows farmers to take timely preventive measures, such as targeted application of pesticides or deployment of biological control methods, minimizing crop damage and reducing reliance on chemical inputs. Moreover, AI-driven pest management systems continuously learn and adapt based on new data, improving their effectiveness over time.
What are the risks associated with AI adoption in farming?
AI adoption in farming presents several risks that need to be addressed. These include high initial costs of technology adoption and infrastructure requirements, which may be prohibitive for smaller farms or developing regions. Data privacy and security concerns arise from the collection, storage, and use of sensitive agricultural data, requiring robust cybersecurity measures and regulatory frameworks. Moreover, AI algorithms may exhibit biases that could lead to unfair outcomes or exacerbate existing disparities in access to technology and resources among farmers. Ensuring equitable access, ethical AI deployment, and comprehensive risk management strategies are crucial for mitigating these risks and maximizing the benefits of AI in agriculture.